import cv2 import torch import argparse from pathlib import Path from models.experimental import attempt_load from utils.general import non_max_suppression, scale_coords from utils.torch_utils import select_device # 定义命令行参数 parser = argparse.ArgumentParser() parser.add_argument('--source', type=str, default='e:/pythonproject/pythonproject/runs/detect/exp2/test1.mp4', help='视频文件路径') parser.add_argument('--weights', type=str, default='e:/pythonproject/pythonproject/best.pt', help='YOLOv5 模型权重文件路径') parser.add_argument('--conf-thres', type=float, default=0.25, help='预测置信度阈值') parser.add_argument('--iou-thres', type=float, default=0.45, help='NMS 的 IoU 阈值') parser.add_argument('--device', default='0', help='使用的 GPU 编号,或者 -1 表示使用 CPU') args = parser.parse_args() # 加载 YOLOv5 模型 device = select_device(args.device) model = attempt_load(args.weights, device=device).to(device).eval() # 加载视频 vid_path = args.source vid_name = Path(vid_path).stem vid_writer = None if vid_path != '0': vid_cap = cv2.VideoCapture(vid_path) else: vid_cap = cv2.VideoCapture(0) assert vid_cap.isOpened(), f'无法打开视频:{vid_path}' # 视频帧循环 while True: # 读取一帧 ret, frame = vid_cap.read() if not ret: break # 对图像进行目标检测 img = torch.from_numpy(frame).to(device) img = img.permute(2, 0, 1).float().unsqueeze(0) / 255.0 pred = model(img)[0] pred = non_max_suppression(pred, args.conf_thres, args.iou_thres, classes=None, agnostic=False) # 处理检测结果 boxes = [] for i, det in enumerate(pred): if len(det): det[:, :4] = scale_coords(img.shape[2:], det[:, :4], frame.shape).round() for xyxy, conf, cls in reversed(det): label = f'{model.names[int(cls)]} {conf:.2f}' boxes.append((int(xyxy[0]), int(xyxy[1]), int(xyxy[2]), int(xyxy[3]), label)) # 绘制矩形框 if len(boxes) > 0: for box in boxes: x1, y1, x2, y2, label = box cv2.rectangle(frame, (x1, y1), (x2, y2), (0, 255, 0), 2) cv2.putText(frame, label, (x1, y1 - 10), cv2.FONT_HERSHEY_SIMPLEX, 0.5, (0, 255, 0), 2) # 显示帧 cv2.imshow(vid_name, frame) # 写入输出视频 if vid_writer is None: fourcc = cv2.VideoWriter_fourcc('mp4v') vid_writer = cv2.VideoWriter(f'{vid_name}_output.mp4', fourcc, 30, (frame.shape[1], frame.shape[0]), True) vid_writer.write(frame) # 按下 q 键退出 if cv2.waitKey(1) == ord('q'): break # 释放资源 vid_cap.release() if vid_writer is not None: vid_writer.release() cv2.destroyAllWindows(),请指出这段代码的错误
时间: 2023-11-22 18:52:15 浏览: 132
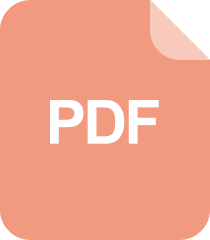
Pycharm中import torch报错的快速解决方法
这段代码没有明显的语法错误,但是需要确保以下几点:
1. 确保已经正确安装了 `cv2` 和 `torch` 库。
2. 确保视频文件路径和权重文件路径是正确的。
3. 确保输入的视频文件格式与代码中指定的编解码器相匹配,否则可能无法写入输出视频。
4. 确保设备编号是正确的,如果使用 GPU,请确保 CUDA 已经正确安装并配置。
另外,建议加上一些错误处理和日志输出,以便更好地追踪代码运行过程中的问题。
阅读全文
相关推荐
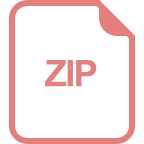
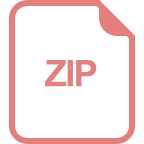















