model.add(Bidirectional(LSTM(units=128,activation='relu',return_sequences=True)))
时间: 2024-04-19 12:25:36 浏览: 35
这段代码是在使用 Keras 构建一个神经网络模型。`Bidirectional` 是一个包装器,它使得 RNN 层既可以正向处理输入序列,又可以逆向处理输入序列。`LSTM` 是一种循环神经网络层,它具有长期依赖记忆能力,并且可以处理序列数据。
在这段代码中,`units=128` 表示 LSTM 层的神经元数量为 128。`activation='relu'` 表示 LSTM 层使用 ReLU 激活函数。`return_sequences=True` 表示 LSTM 层返回完整的输出序列,而不仅仅是最后一个时间步的输出。
最后,`model.add()` 是用于向模型添加层的方法。通过将 `Bidirectional(LSTM(...))` 作为参数传递给 `model.add()`,将双向 LSTM 层添加到模型中。
相关问题
如何修改为seq2seq : input_data1 = Input(shape=(time_steps1, input_dim1)) # 添加卷积层, 增大卷积大小 conv1d_1 = Conv1D(filters=64, kernel_size=5, activation='relu')(input_data1) # 添加多头self-attention机制 context1 = multi_head_attention(conv1d_1,5) # 通过增加层数和隐藏单元的数量,可以增加模型的复杂度和表现能力,但也可能导致过拟合问题 lstm1 = Bidirectional(LSTM(128, return_sequences=True))(context1) # 加入双向LSTM层 lstm1 = Bidirectional(LSTM(64, return_sequences=True))(lstm1) lstm1 = Bidirectional(LSTM(32, return_sequences=True))(lstm1) lstm1 = BatchNormalization()(lstm1) #lstm1 = LSTM(128, return_sequences=True)(context_dim2) # 加入LSTM层 lstm_out1 = Dense(64, activation='relu')(lstm1) lstm_out1 = Dense(32, activation='tanh')(lstm_out1) lstm_out1 = Dense(16, activation='softmax')(lstm_out1)
要将这段代码修改为seq2seq模型,需要使用Encoder-Decoder框架。具体步骤如下:
1. 定义Encoder层,在这里可以使用LSTM或者GRU等循环神经网络。将输入数据input_data1传入Encoder层,得到encoder_output和encoder_state。
2. 定义Decoder层,在这里也可以使用LSTM或者GRU等循环神经网络。将Encoder层的输出encoder_output和encoder_state作为Decoder层的输入,得到decoder_output。
3. 定义输出层,将decoder_output传入Dense层,得到最终的输出。
修改后的代码如下所示:
```python
from keras.layers import Input, Conv1D, LSTM, Bidirectional, Dense, BatchNormalization
from keras.models import Model
# 定义Encoder层
input_data1 = Input(shape=(time_steps1, input_dim1))
conv1d_1 = Conv1D(filters=64, kernel_size=5, activation='relu')(input_data1)
context1 = multi_head_attention(conv1d_1, 5)
encoder_lstm1 = Bidirectional(LSTM(128, return_state=True))
encoder_outputs, forward_h, forward_c, backward_h, backward_c = encoder_lstm1(context1)
encoder_state = [forward_h, forward_c, backward_h, backward_c]
# 定义Decoder层
decoder_input = Input(shape=(time_steps2, input_dim2))
decoder_lstm1 = Bidirectional(LSTM(128, return_sequences=True))
decoder_lstm2 = Bidirectional(LSTM(64, return_sequences=True))
decoder_lstm3 = Bidirectional(LSTM(32, return_sequences=True))
decoder_bn = BatchNormalization()
decoder_dense1 = Dense(64, activation='relu')
decoder_dense2 = Dense(32, activation='tanh')
decoder_dense3 = Dense(16, activation='softmax')
decoder_outputs = decoder_lstm1(decoder_input, initial_state=encoder_state)
decoder_outputs = decoder_lstm2(decoder_outputs)
decoder_outputs = decoder_lstm3(decoder_outputs)
decoder_outputs = decoder_bn(decoder_outputs)
decoder_outputs = decoder_dense1(decoder_outputs)
decoder_outputs = decoder_dense2(decoder_outputs)
decoder_outputs = decoder_dense3(decoder_outputs)
# 定义模型
model = Model([input_data1, decoder_input], decoder_outputs)
```
我有一个单步模型,若改为多步预测,如何修改 : input1 = Input(shape=(trainX1.shape[1], trainX1.shape[2])) conv1 = Conv1D(filters=128 , kernel_size=config.CK, strides=1, activation='relu') # for input1 # 多输入权重共享 conv2 = Conv1D(filters=128 , kernel_size=config.CK, strides=config.CK, activation='relu') # for input2 conv2.set_weights(conv1.get_weights()) # at least use same weight # CNN conv1out = conv1(input1) conv1out = Dropout(config.dropout)(conv1out) # RNN lstm1out = LSTM(config.lstm_batch_size)(conv1out) lstm1out = Dropout(config.dropout)(lstm1out) # Input2: long-term time series with period input2 = Input(shape=(trainX2.shape[1], trainX2.shape[2])) # CNN conv2out = conv2(input2) conv2out = Dropout(config.dropout)(conv2out) # RNN lstm2out = LSTM(config.lstm_batch_size)(conv2out) lstm2out = Dropout(config.dropout)(lstm2out) lstm_out = concatenate([lstm1out,lstm2out]) model.add(keras.layers.Embedding(input_dim=10000, output_dim=300, mask_zero=True)) model.add(keras.layers.Bidirectional(keras.layers.LSTM(units=128, return_sequences=True))) #model.add(SeqSelfAttention(attention_activation='sigmoid')) model.add(keras.layers.Dense(units=5)) #x = BatchNormalization() #x = Activation('relu')(x) res = Dense(trainY.shape[1])(lstm_out)
若要将单步预测模型改为多步预测模型,需要进行以下修改:
1. 修改网络结构。多步预测模型需要考虑多个时间步的输出,因此需要对模型进行修改,使其能够同时预测多个时间步的输出。可以在模型最后添加一个长度为多步预测步数的全连接层,输出预测结果。
2. 修改损失函数和评价指标。多步预测模型需要考虑多个时间步的输出,因此需要使用适合多步预测的损失函数和评价指标。例如,可以使用平均绝对误差(mean absolute error,MAE)作为损失函数和评价指标。
下面是可能的修改代码:
```
# 修改网络结构
input1 = Input(shape=(trainX1.shape[1], trainX1.shape[2]))
conv1 = Conv1D(filters=128 , kernel_size=config.CK, strides=1, activation='relu') # for input1
# 多输入权重共享
conv2 = Conv1D(filters=128 , kernel_size=config.CK, strides=config.CK, activation='relu') # for input2
conv2.set_weights(conv1.get_weights()) # at least use same weight
# CNN
conv1out = conv1(input1)
conv1out = Dropout(config.dropout)(conv1out)
# RNN
lstm1out = LSTM(config.lstm_batch_size)(conv1out)
lstm1out = Dropout(config.dropout)(lstm1out)
# Input2: long-term time series with period
input2 = Input(shape=(trainX2.shape[1], trainX2.shape[2]))
# CNN
conv2out = conv2(input2)
conv2out = Dropout(config.dropout)(conv2out)
# RNN
lstm2out = LSTM(config.lstm_batch_size)(conv2out)
lstm2out = Dropout(config.dropout)(lstm2out)
lstm_out = concatenate([lstm1out,lstm2out])
model.add(keras.layers.Embedding(input_dim=10000, output_dim=300, mask_zero=True))
model.add(keras.layers.Bidirectional(keras.layers.LSTM(units=128, return_sequences=True)))
#model.add(SeqSelfAttention(attention_activation='sigmoid'))
model.add(keras.layers.Dense(units=5))
model.add(keras.layers.Dense(units=多步预测步数)) # 添加多步预测输出层
# 修改损失函数和评价指标
model.compile(loss='mean_absolute_error', optimizer='adam', metrics=['mean_absolute_error'])
```
需要注意的是,多步预测模型的训练时间较长,需要更多的训练数据和计算资源。
相关推荐
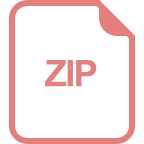












