用以下代码:import random def choice_n (n): n_ = [x for x in range(10)] m_ = [] if n > 0: for i in range(n): #书写答案 #书写答案 #书写答案 t = [str(x) for x in m_] t = "".join(t) return eval(t) else: return -1 print(choice_n(7))
时间: 2024-02-10 16:17:17 浏览: 47
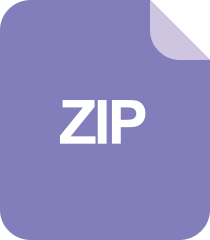
jjj.zip_jjj aloha_slotted aloha _zip
好的,我知道你想要我完成的任务了。你需要我在 `# 书写答案` 的位置上写入代码,以便 `choice_n` 函数可以随机选择 `n` 个不同的数字,并将它们组成一个整数并返回。
以下是代码示例:
```python
import random
def choice_n(n):
n_ = [x for x in range(10)]
m_ = []
if n > 0:
for i in range(n):
num = random.choice(n_)
m_.append(num)
n_.remove(num) # 从列表中移除已经选择的数字
t = [str(x) for x in m_]
t = "".join(t)
return int(t)
else:
return -1
print(choice_n(7))
```
这个代码会在 `n_` 列表中选择 `n` 个不同的数字,并将它们放入 `m_` 列表中,然后将 `m_` 中的数字拼接成一个整数并返回。注意,我们需要使用 `random.choice()` 函数来从列表中随机选择一个数字,并且需要在选择后从 `n_` 中移除该数字,以确保不会选择重复的数字。
阅读全文
相关推荐
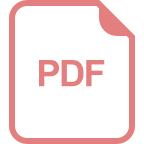
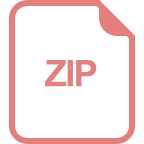
















