out4 = torch.cpu().detach().numpy()
时间: 2023-03-29 09:02:02 浏览: 70
这是一个关于 PyTorch 的代码问题,我可以回答。这行代码的作用是将 PyTorch 张量 out4 转换为 NumPy 数组,并将其从计算设备(如 GPU)中分离出来,转移到 CPU 上进行处理。
相关问题
def plot _ pred ( dv _ set , model , device , lim =35., preds = None , targetsNone ): if preds is Mone or targets is Mone : model . eval () preds , targets =[],[] for x , y in dv _ set : x , y = x . to ( device ), y . to ( device ) with torch . no _ grad (): pred = model ( x ) preds . append ( pred . detach (). cpu ()) targets . append ( y . detach (). cpu ()) preds = torch . cat ( preds , dim =0). numpy () targets = torch . cat ( targets , dim =0). numpy ()
这段代码存在一些错误,我来帮你修正一下:
```python
def plot_pred(dv_set, model, device, lim=35., preds=None, targets=None):
if preds is None or targets is None:
model.eval()
preds, targets = [], []
for x, y in dv_set:
x, y = x.to(device), y.to(device)
with torch.no_grad():
pred = model(x)
preds.append(pred.detach().cpu())
targets.append(y.detach().cpu())
preds = torch.cat(preds, dim=0).numpy()
targets = torch.cat(targets, dim=0).numpy()
# 绘制图形的代码缺失,你可以在这里添加绘图的相关代码
# 返回预测值和目标值
return preds, targets
```
修正后的代码将原来缺失的绘图代码部分注释掉了,你可以在这里添加你希望使用的绘图代码。同时,我添加了一个返回语句,这样函数可以返回预测值和目标值给调用者。
请注意,由于缺失了绘图代码,你需要根据你的需求添加合适的绘图逻辑来完成预测结果的可视化。
import torch import numpy as np import matplotlib.pyplot as plt x = np.linspace(-10, 10, num=100) act = torch.nn.SiLU() pred = act(torch.from_numpy(x)) y = pred.detach().numpy() plt.plot(x, y) plt.show()使图像一直停留界面
可以在代码最后加上`plt.waitforbuttonpress()`或者`plt.pause(0.001)`,来使图像停留在界面上。例如:
```
import torch
import numpy as np
import matplotlib.pyplot as plt
x = np.linspace(-10, 10, num=100)
act = torch.nn.SiLU()
pred = act(torch.from_numpy(x))
y = pred.detach().numpy()
plt.plot(x, y)
plt.show()
plt.waitforbuttonpress()
```
或者
```
import torch
import numpy as np
import matplotlib.pyplot as plt
x = np.linspace(-10, 10, num=100)
act = torch.nn.SiLU()
pred = act(torch.from_numpy(x))
y = pred.detach().numpy()
plt.plot(x, y)
plt.show()
plt.pause(0.001)
```
这样就可以使图像一直停留在界面上,直到用户手动关闭。
相关推荐
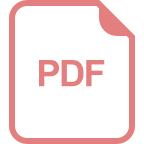













