# 定义获得时序最大值坐标的函数 def TSMaxIndex(array, first = True): array = np.array(array).flatten() if first: return np.argmax(array) else: max_index = np.argwhere(array == np.amax(array)).flatten() return max_index[-1]
时间: 2024-04-19 13:26:34 浏览: 135
TSMaxIndex函数是一个用于获得时序最大值坐标的函数。它接受一个数组作为输入,并可选地指定返回第一个最大值的索引还是最后一个最大值的索引。首先,将输入数组转换为一维数组。如果指定返回第一个最大值的索引(first参数为True),则使用numpy的argmax方法返回第一个最大值的索引。如果指定返回最后一个最大值的索引(first参数为False),则使用numpy的argwhere方法找到所有最大值的索引,然后返回最后一个最大值的索引。
相关问题
解释:def levenberg_marquardt(fun, grad, jacobian, x0, iterations, tol): """ Minimization of scalar function of one or more variables using the Levenberg-Marquardt algorithm. Parameters ---------- fun : function Objective function. grad : function Gradient function of objective function. jacobian :function function of objective function. x0 : numpy.array, size=9 Initial value of the parameters to be estimated. iterations : int Maximum iterations of optimization algorithms. tol : float Tolerance of optimization algorithms. Returns ------- xk : numpy.array, size=9 Parameters wstimated by optimization algorithms. fval : float Objective function value at xk. grad_val : float Gradient value of objective function at xk. grad_log : numpy.array The record of gradient of objective function of each iteration. """ fval = None # y的最小值 grad_val = None # 梯度的最后一次下降的值 x_log = [] # x的迭代值的数组,n*9,9个参数 y_log = [] # y的迭代值的数组,一维 grad_log = [] # 梯度下降的迭代值的数组 x0 = asarray(x0).flatten() if x0.ndim == 0: x0.shape = (1,) # iterations = len(x0) * 200 k = 1 xk = x0 updateJ = 1 lamda = 0.01 old_fval = fun(x0) gfk = grad(x0) gnorm = np.amax(np.abs(gfk)) J = [None] H = [None] while (gnorm > tol) and (k < iterations): if updateJ == 1: x_log = np.append(x_log, xk.T) yk = fun(xk) y_log = np.append(y_log, yk) J = jacobian(x0) H = np.dot(J.T, J) H_lm = H + (lamda * np.eye(9)) gfk = grad(xk) pk = - np.linalg.inv(H_lm).dot(gfk) pk = pk.A.reshape(1, -1)[0] # 二维变一维 xk1 = xk + pk fval = fun(xk1) if fval < old_fval: lamda = lamda / 10 xk = xk1 old_fval = fval updateJ = 1 else: updateJ = 0 lamda = lamda * 10 gnorm = np.amax(np.abs(gfk)) k = k + 1 grad_log = np.append(grad_log, np.linalg.norm(xk - x_log[-1:])) fval = old_fval grad_val = grad_log[-1] return xk, fval, grad_val, x_log, y_log, grad_log
这段代码实现了使用Levenberg-Marquardt算法最小化一个或多个变量的标量函数。其中fun参数是目标函数,grad参数是目标函数的梯度函数,jacobian参数是目标函数的雅可比矩阵函数,x0参数是参数的初始值,iterations参数是算法的最大迭代次数,tol参数是算法的容差。该函数返回最小化目标函数的参数值xk,目标函数在xk处的值fval,目标函数在xk处的梯度grad_val,迭代过程中x的值的数组x_log,迭代过程中y的值的数组y_log,以及梯度下降迭代值的数组grad_log。算法的核心是通过计算目标函数的梯度和雅可比矩阵,使用Levenberg-Marquardt算法不断迭代优化参数值,直到达到指定的容差或最大迭代次数为止。
def load_cora(): path = 'data/cora/' data_name = 'cora' print('Loading from raw data file...') idx_features_labels = np.genfromtxt("{}{}.content".format(path, data_name), dtype=np.dtype(str)) features = sp.csr_matrix(idx_features_labels[:, 1:-1], dtype=np.float32) _, _, labels = np.unique(idx_features_labels[:, -1], return_index=True, return_inverse=True) idx = np.array(idx_features_labels[:, 0], dtype=np.int32) idx_map = {j: i for i, j in enumerate(idx)} edges_unordered = np.genfromtxt("{}{}.cites".format(path, data_name), dtype=np.int32) edges = np.array(list(map(idx_map.get, edges_unordered.flatten())), dtype=np.int32).reshape(edges_unordered.shape) adj = sp.coo_matrix((np.ones(edges.shape[0]), (edges[:, 0], edges[:, 1])), shape=(labels.shape[0], labels.shape[0]), dtype=np.float32) adj = adj.T + adj adj = adj.minimum(1) return features.toarray(), idx_map, adj.toarray(), labels
这是一个函数,用于从Cora数据集的原始数据文件中加载数据。它返回特征、节点索引映射、邻接矩阵和标签。具体来说,它使用numpy库中的genfromtxt函数从包含节点特征和标签的文件中加载数据;使用coo_matrix函数从包含节点之间引用关系的文件中加载数据,并将其转换为邻接矩阵形式;最后,对邻接矩阵进行了处理,使其成为一个二元关系矩阵。
阅读全文
相关推荐

















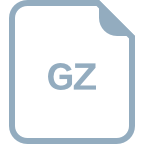