in inplace_predict raise ValueError( ValueError: Feature shape mismatch, expected: 2, got 5
时间: 2024-05-17 08:18:28 浏览: 8
这个错误可能是由于数据形状不匹配导致的。在进行预测时,模型期望输入的特征形状为2,但是实际输入的特征形状为5,因此引发了该错误。您需要检查您的代码,确保输入的特征与模型期望的特征形状相匹配。您可以查看数据的形状,并将其与模型的输入形状进行比较,以确定数据是否正确。另外,您还可以检查您的模型代码,确保输入层的形状与您的数据一致。
相关问题
File D:\Anaconda3\lib\site-packages\xgboost\core.py:2268 in inplace_predict raise ValueError( ValueError: Feature shape mismatch, expected: 2, got 5
这个错误提示表明你使用的 XGBoost 模型期望输入的特征形状为 (n_samples, 2),但是实际上输入的特征形状为 (n_samples, 5),因此出现了形状不匹配的错误。
建议你检查一下模型训练时使用的特征数量是否与测试时使用的特征数量一致,以及测试数据的特征形状是否正确。你可以使用以下代码检查测试数据的特征形状:
```
import numpy as np
test_data = np.random.rand(n_samples, 5) # 假设测试数据有 n_samples 个样本,每个样本有 5 个特征
print(test_data.shape) # 打印测试数据的特征形状
```
如果测试数据的特征形状确实为 (n_samples, 5),那么你需要检查一下训练模型时使用的特征数量,确保其与测试时使用的特征数量一致。
绘制训练集和测试集的真实值和预测值图像 train_predict_plot = np.empty_like(data_scaled) train_predict_plot[:, :] = np.nan train_predict_plot[time_steps:len(train_predict) + time_steps, :] = train_predict test_predict_plot = np.empty_like(data_scaled) test_predict_plot[:, :] = np.nan test_predict_plot[len(train_predict) + time_steps * 2 + 1:len(data_scaled) - 1, :] = test_predict plt.figure(figsize=(10, 6)) plt.plot(scaler.inverse_transform(data_scaled)) plt.plot(train_predict_plot) plt.plot(test_predict_plot) plt.legend(['True', 'Train Predict', 'Test Predict']) plt.xlabel('Time/h') plt.ylabel('kwh') plt.show(改写以上代码,使得训练集部分和测试集部分分别绘制在两张图上
下面是改写后的代码,将训练集和测试集的真实值和预测值分别绘制在两张图上:
```
train_predict_plot = np.empty_like(data_scaled)
train_predict_plot[:, :] = np.nan
train_predict_plot[time_steps:len(train_predict) + time_steps, :] = train_predict
test_predict_plot = np.empty_like(data_scaled)
test_predict_plot[:, :] = np.nan
test_predict_plot[len(train_predict) + time_steps * 2 + 1:len(data_scaled) - 1, :] = test_predict
# 绘制训练集部分真实值和预测值图像
plt.figure(figsize=(10, 6))
plt.plot(scaler.inverse_transform(data_scaled[:len(train_predict)]))
plt.plot(train_predict_plot[:len(train_predict)])
plt.legend(['True', 'Train Predict'])
plt.xlabel('Time/h')
plt.ylabel('kwh')
plt.title('Training Set')
plt.show()
# 绘制测试集部分真实值和预测值图像
plt.figure(figsize=(10, 6))
plt.plot(scaler.inverse_transform(data_scaled[len(train_predict) + time_steps * 2 + 1:]))
plt.plot(test_predict_plot[len(train_predict) + time_steps * 2 + 1:])
plt.legend(['True', 'Test Predict'])
plt.xlabel('Time/h')
plt.ylabel('kwh')
plt.title('Test Set')
plt.show()
```
相关推荐
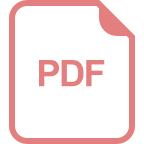
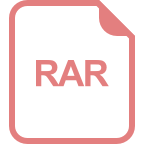
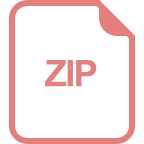












