logit_scale
时间: 2023-09-13 17:03:04 浏览: 101
logit_scale 是一个用于缩放模型生成的对数几率(logits)的参数。在机器学习中,logits 是指模型的输出,在分类问题中表示每个类别的得分或概率。通过调整 logit_scale,可以改变模型输出的范围和分布。较大的 logit_scale 值会使得模型输出的范围更广,可能会增加模型对于极端情况的敏感性,而较小的 logit_scale 值则可能使得模型输出更集中在中间区域。调整 logit_scale 的值可以根据具体任务和数据的特点进行优化,以提升模型性能和适应性。
相关问题
clip logit_scale
logit_scale的作用是对logit值进行裁剪或限制在一个指定区间内。在给定的代码引用中,通过使用torch.clamp函数对logit_scale进行裁剪,将其限制在0和4.6052之间。这样做的目的是为了确保logit_scale的取值范围在一个合理的区间内,并且避免出现过大或过小的值影响模型的训练和预测结果。具体来说,logit_scale可以用来调整模型中logit值的范围,从而影响模型对不同类别的概率估计。<span class="em">1</span><span class="em">2</span><span class="em">3</span>
#### 引用[.reference_title]
- *1* *2* *3* [借京东图文识别baseline 来看clip训练过程。 clip是怎样练成的 。](https://blog.csdn.net/YI_SHU_JIA/article/details/123714897)[target="_blank" data-report-click={"spm":"1018.2226.3001.9630","extra":{"utm_source":"vip_chatgpt_common_search_pc_result","utm_medium":"distribute.pc_search_result.none-task-cask-2~all~insert_cask~default-1-null.142^v93^chatsearchT3_1"}}] [.reference_item style="max-width: 100%"]
[ .reference_list ]
rstudio中logit模型代码
### 回答1:
在 RStudio 中使用逻辑回归(logit 模型)的代码示例如下:
```
# 首先需要加载相应的包
library(glm)
# 假设我们有一个数据框 df,其中包含了自变量和因变量
# 建立逻辑回归模型
logit_model <- glm(formula = 因变量 ~ 自变量, family = binomial(link = "logit"), data = df)
# 打印模型结果
summary(logit_model)
# 使用模型进行预测
predictions <- predict(logit_model, newdata = df, type = "response")
```
在上述代码中,"因变量" 和 "自变量" 分别表示您希望预测的目标变量和用于预测的自变量。这些变量应该是您的数据框中的列名称。您可以使用 `formula` 参数来指定模型中使用的变量,并使用 `family = binomial(link = "logit")` 参数来指定逻辑回归模型。最后,使用 `data` 参数指定要使用的数据框。
在使用 `summary()` 函数打印模型结果之后,您可以使用 `predict()` 函数来使用模型进行预测。这里,使用 `newdata` 参数指定要用于预测的数据,并使用 `type = "response"` 参数指定输出类型。
希望这些信息能帮到您。
### 回答2:
在RStudio中,我们可以使用logit模型对二分类问题进行建模和预测。logit模型是一种经典的统计模型,常用于分析因变量为二元型(例如0和1)的情况。
首先,我们需要安装并导入适用于logit模型的包,如“caret”和“glm”包。可以使用以下命令进行安装和导入:
```R
install.packages("caret")
install.packages("glm")
library(caret)
library(glm)
```
接下来,我们需要准备数据并进行预处理。假设我们的数据集名为“data”,且拥有一个二元的因变量“y”,和一组自变量“x1”和“x2”:
```R
# 读取数据
data <- read.csv("data.csv")
# 数据预处理,例如去除缺失值或对字符串进行编码等
data <- preProcess(data, method = c("center", "scale"))
# 创建训练集和测试集
set.seed(123)
trainIndex <- createDataPartition(data$y, p = 0.8, list = FALSE)
train <- data[trainIndex, ]
test <- data[-trainIndex, ]
```
接下来,我们可以使用logit模型进行建模和预测。logit模型是通过最大似然估计(Maximum Likelihood Estimation,MLE)来拟合因变量和自变量之间的关系。
```R
# 建立logit模型
logitModel <- glm(y ~ x1 + x2, data = train, family = binomial)
# 输出模型摘要
summary(logitModel)
# 预测测试集中的结果
predictions <- predict(logitModel, newdata = test, type = "response")
```
最后,我们可以评估模型的性能。常见的评估指标包括准确率、精确率、召回率等。可以使用混淆矩阵来计算这些指标:
```R
# 创建混淆矩阵
confMatrix <- confusionMatrix(predictions, test$y)
# 输出评估结果
print(confMatrix)
```
以上就是在RStudio中进行logit模型建模和预测的简单代码示例。希望对你有所帮助!
### 回答3:
在RStudio中实现logit模型有多种方式,其中最常用的是使用`glm()`函数。以下是一个可能的logit模型代码示例:
```R
# 导入数据集(假设数据集包含对响应变量和解释变量的观测)
data <- read.csv("data.csv")
# 构建logit模型
model <- glm(Response ~ Predictor1 + Predictor2, data = data, family = binomial(link = "logit"))
# 模型概览
print(summary(model))
# 预测
predictions <- predict(model, newdata = data, type = "response")
# 预测结果转为二进制分类(根据设定的阈值)
binary_predictions <- ifelse(predictions > 0.5, 1, 0)
# 绘制ROC曲线
library(pROC)
roc_obj <- roc(data$Response, predictions)
plot(roc_obj, print.auc = TRUE, main = "ROC Curve")
# 获取系数和显著性
coefficients <- summary(model)$coefficients
```
在上述代码中,`data.csv`是数据集文件的名称,` Response`是响应变量的名称,而`Predictor1`和`Predictor2`是解释变量的名称。`binomial(link = "logit")`参数指定了使用logit函数作为模型的链接函数。`glm()`函数返回模型对象,可使用`summary()`函数显示模型的详细结果,包括系数、显著性和AUC等。使用`predict()`函数进行预测,并使用`pROC`包中的`roc()`和`plot()`函数绘制ROC曲线。最后,`summary(model)$coefficients`可以获取模型的系数和显著性。根据具体需求,可以根据实际情况对代码进行调整和优化。
相关推荐
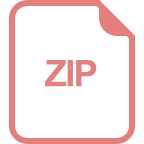













