def update(self, predictions): img_ids = list(np.unique(list(predictions.keys()))) self.img_ids.extend(img_ids) for iou_type in self.iou_types: results = self.prepare(predictions, iou_type) coco_dt = loadRes(self.coco_gt, results) if results else COCO() coco_eval = self.coco_eval[iou_type] coco_eval.cocoDt = coco_dt coco_eval.params.imgIds = list(img_ids) img_ids, eval_imgs = evaluate(coco_eval) self.eval_imgs[iou_type].append(eval_imgs)
时间: 2024-04-09 21:27:15 浏览: 25
这段代码是一个`update`方法,它接受一个预测结果`predictions`作为输入。该方法用于更新评估器对象的状态并计算评估指标。
具体步骤如下:
1. 获取预测结果中的图像ID,并将其与之前的图像ID列表进行合并,以确保所有图像ID都被记录在`self.img_ids`中。
2. 对每个IOU类型进行循环迭代(可能是不同的IOU阈值或IOU计算方式)。
3. 调用`self.prepare(predictions, iou_type)`方法来准备预测结果,将其转换为COCO API可接受的格式。
4. 如果结果不为空,则使用`loadRes`函数加载预测结果到一个`coco_dt`对象中,否则创建一个空的`COCO`对象。
5. 获取对应IOU类型的`coco_eval`对象,并设置其`cocoDt`属性为刚刚生成的`coco_dt`对象。
6. 将图像ID列表设置为刚刚合并的图像ID列表,并调用`evaluate`函数来计算评估指标。此过程会返回更新后的图像ID列表`img_ids`和评估结果`eval_imgs`。
7. 将更新后的图像ID列表和评估结果分别添加到`self.eval_imgs[iou_type]`和`self.eval_imgs[iou_type]`中。
这段代码的目的是通过调用COCO API来计算评估指标,并将结果存储在评估器对象的相应属性中。
如果还有其他问题,请随时提问。
相关问题
帮我为下面的代码加上注释:class SimpleDeepForest: def __init__(self, n_layers): self.n_layers = n_layers self.forest_layers = [] def fit(self, X, y): X_train = X for _ in range(self.n_layers): clf = RandomForestClassifier() clf.fit(X_train, y) self.forest_layers.append(clf) X_train = np.concatenate((X_train, clf.predict_proba(X_train)), axis=1) return self def predict(self, X): X_test = X for i in range(self.n_layers): X_test = np.concatenate((X_test, self.forest_layers[i].predict_proba(X_test)), axis=1) return self.forest_layers[-1].predict(X_test[:, :-2]) # 1. 提取序列特征(如:GC-content、序列长度等) def extract_features(fasta_file): features = [] for record in SeqIO.parse(fasta_file, "fasta"): seq = record.seq gc_content = (seq.count("G") + seq.count("C")) / len(seq) seq_len = len(seq) features.append([gc_content, seq_len]) return np.array(features) # 2. 读取相互作用数据并创建数据集 def create_dataset(rna_features, protein_features, label_file): labels = pd.read_csv(label_file, index_col=0) X = [] y = [] for i in range(labels.shape[0]): for j in range(labels.shape[1]): X.append(np.concatenate([rna_features[i], protein_features[j]])) y.append(labels.iloc[i, j]) return np.array(X), np.array(y) # 3. 调用SimpleDeepForest分类器 def optimize_deepforest(X, y): X_train, X_test, y_train, y_test = train_test_split(X, y, test_size=0.2) model = SimpleDeepForest(n_layers=3) model.fit(X_train, y_train) y_pred = model.predict(X_test) print(classification_report(y_test, y_pred)) # 4. 主函数 def main(): rna_fasta = "RNA.fasta" protein_fasta = "pro.fasta" label_file = "label.csv" rna_features = extract_features(rna_fasta) protein_features = extract_features(protein_fasta) X, y = create_dataset(rna_features, protein_features, label_file) optimize_deepforest(X, y) if __name__ == "__main__": main()
# Define a class named 'SimpleDeepForest'
class SimpleDeepForest:
# Initialize the class with 'n_layers' parameter
def __init__(self, n_layers):
self.n_layers = n_layers
self.forest_layers = []
# Define a method named 'fit' to fit the dataset into the classifier
def fit(self, X, y):
X_train = X
# Use the forest classifier to fit the dataset for 'n_layers' times
for _ in range(self.n_layers):
clf = RandomForestClassifier()
clf.fit(X_train, y)
# Append the classifier to the list of forest layers
self.forest_layers.append(clf)
# Concatenate the training data with the predicted probability of the last layer
X_train = np.concatenate((X_train, clf.predict_proba(X_train)), axis=1)
# Return the classifier
return self
# Define a method named 'predict' to make predictions on the test set
def predict(self, X):
X_test = X
# Concatenate the test data with the predicted probability of each layer
for i in range(self.n_layers):
X_test = np.concatenate((X_test, self.forest_layers[i].predict_proba(X_test)), axis=1)
# Return the predictions of the last layer
return self.forest_layers[-1].predict(X_test[:, :-2])
# Define a function named 'extract_features' to extract sequence features
def extract_features(fasta_file):
features = []
# Parse the fasta file to extract sequence features
for record in SeqIO.parse(fasta_file, "fasta"):
seq = record.seq
gc_content = (seq.count("G") + seq.count("C")) / len(seq)
seq_len = len(seq)
features.append([gc_content, seq_len])
# Return the array of features
return np.array(features)
# Define a function named 'create_dataset' to create the dataset
def create_dataset(rna_features, protein_features, label_file):
labels = pd.read_csv(label_file, index_col=0)
X = []
y = []
# Create the dataset by concatenating the RNA and protein features
for i in range(labels.shape[0]):
for j in range(labels.shape[1]):
X.append(np.concatenate([rna_features[i], protein_features[j]]))
y.append(labels.iloc[i, j])
# Return the array of features and the array of labels
return np.array(X), np.array(y)
# Define a function named 'optimize_deepforest' to optimize the deep forest classifier
def optimize_deepforest(X, y):
# Split the dataset into training set and testing set
X_train, X_test, y_train, y_test = train_test_split(X, y, test_size=0.2)
# Create an instance of the SimpleDeepForest classifier with 3 layers
model = SimpleDeepForest(n_layers=3)
# Fit the training set into the classifier
model.fit(X_train, y_train)
# Make predictions on the testing set
y_pred = model.predict(X_test)
# Print the classification report
print(classification_report(y_test, y_pred))
# Define the main function to run the program
def main():
rna_fasta = "RNA.fasta"
protein_fasta = "pro.fasta"
label_file = "label.csv"
# Extract the RNA and protein features
rna_features = extract_features(rna_fasta)
protein_features = extract_features(protein_fasta)
# Create the dataset
X, y = create_dataset(rna_features, protein_features, label_file)
# Optimize the DeepForest classifier
optimize_deepforest(X, y)
# Check if the program is being run as the main program
if __name__ == "__main__":
main()
class Age_Model(): def __init__(self): self.model = self.loadModel() self.output_indexes = np.array([i for i in range(0, 101)]) def predict_age(self,face_image): image_preprocesing = self.transform_face_array2age_face(face_image) age_predictions = self.model.predict(image_preprocesing )[0,:] result_age = self.findApparentAge(age_predictions) return result_age def loadModel(self): model = VGGFace.baseModel() #-------------------------- classes = 101#101 base_model_output = Sequential() base_model_output = Convolution2D(classes, (1, 1), name='predictions')(model.layers[-4].output) base_model_output = Flatten()(base_model_output) base_model_output = Activation('softmax')(base_model_output) #-------------------------- age_model = Model(inputs=model.input, outputs=base_model_output) #-------------------------- home = str(Path.home()) age_model.load_weights(home+'/.deepface/weights/age_model_weights.h5') return age_model
这段代码是一个基于VGGFace模型的年龄预测模型。其中,loadModel函数用于加载预训练的模型权重;predict_age函数用于对输入的人脸图像进行预测,返回预测结果的年龄。
具体来说,该模型使用VGGFace模型作为基础模型。在VGGFace模型上,我们添加了一个具有101个输出类别的全连接层,用于预测101个年龄区间。具体的输出层添加代码为:
```
base_model_output = Convolution2D(classes, (1, 1), name='predictions')(model.layers[-4].output)
base_model_output = Flatten()(base_model_output)
base_model_output = Activation('softmax')(base_model_output)
```
在预测时,输入的人脸图像经过预处理之后,通过该模型进行预测,得到101个年龄区间的预测概率。最终,根据预测概率的分布,计算出预测的年龄值。
相关推荐
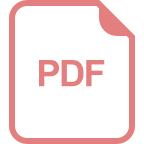
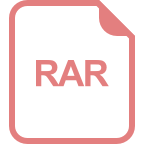













