R语言 fit th Poisson GLM with a logarithmic link function ,using the systematic component Count~Species+factor(Quadrat),plot the deviance residuals against the fitted values and against thefitted values transformed to the constant information scale
时间: 2024-02-25 12:56:54 浏览: 25
Sure, I can help you with that. Here's how you can fit a Poisson GLM with a logarithmic link function in R:
```R
# Load the necessary packages
library(stats)
# Fit the Poisson GLM with a logarithmic link function
fit <- glm(Count ~ Species + factor(Quadrat), family = "poisson", link = "log")
# Plot the deviance residuals against the fitted values
plot(fit$fitted.values, residuals(fit, type = "deviance"), xlab = "Fitted values", ylab = "Deviance residuals")
# Plot the deviance residuals against the fitted values transformed to the constant information scale
plot(qnorm((fit$fitted.values + 1/2) / sum(fit$fitted.values + 1/2)), residuals(fit, type = "deviance"), xlab = "Fitted values transformed to constant information scale", ylab = "Deviance residuals")
```
In the first plot, we plot the deviance residuals against the fitted values, which gives us an idea of whether the model is fitting the data well or not. In the second plot, we plot the deviance residuals against the fitted values transformed to the constant information scale, which is a way of standardizing the fitted values and allows for easier comparison of the residuals across different parts of the fitted values.
相关推荐
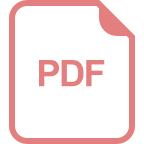











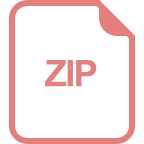