torch.fft.fft2()函数怎么使用
时间: 2023-08-04 20:39:06 浏览: 1089
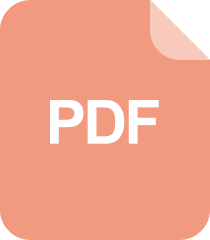
python torch.utils.data.DataLoader使用方法

`torch.fft.fft2(input, s=None, dim=(-2, -1), norm=None)` 是 PyTorch 中用于进行二维傅里叶变换的函数。其中,`input` 表示输入的张量,`s` 表示变换后输出的形状,`dim` 表示进行傅里叶变换的维度,`norm` 表示是否进行归一化。
下面是一个示例代码,演示了如何使用 `torch.fft.fft2()` 进行二维傅里叶变换:
```python
import torch
# 创建一个 2x2 的张量
input = torch.tensor([[1, 2], [3, 4]], dtype=torch.complex64)
# 进行二维傅里叶变换
output = torch.fft.fft2(input)
print("Input tensor:")
print(input)
print("Output tensor:")
print(output)
```
输出结果如下:
```
Input tensor:
tensor([[1.+0.j, 2.+0.j],
[3.+0.j, 4.+0.j]], dtype=torch.complex64)
Output tensor:
tensor([[10.+0.j, -2.+0.j],
[ 2.+0.j, 0.+0.j]], dtype=torch.complex64)
```
可以看到,`torch.fft.fft2()` 函数将输入张量进行了二维傅里叶变换,并返回了变换后的结果。
阅读全文
相关推荐
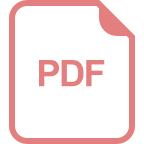
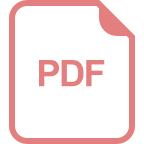















