x = values[-365:] distance = []for i in range(len(values)-730): y = values[i:i+365] dis, path = fastdtw(x, y, dist=euclidean) distance.append(dis)代码什么意思
时间: 2024-03-04 10:54:26 浏览: 139
这段代码与之前的那段代码相似,也是使用fastdtw算法计算两个时间序列x和y之间的动态时间规整(Dynamic Time Warping, DTW)距离。不同的是,这里的x是从values序列中取出的最后365个值,也就是最近一年的时间序列。对于每个从values序列中以步长为1滑动窗口取出的长度为365的子序列y,计算其与最近一年的时间序列x之间的DTW距离,并将距离值添加到一个名为distance的列表中,最终得到所有子序列与最近一年时间序列的DTW距离列表。这段代码的作用也是对时间序列进行相似性度量,用于时间序列分类、聚类、异常检测等应用场景。
相关问题
import random import numpy as np import matplotlib.pyplot as plt 生成随机坐标点 def generate_points(num_points): points = [] for i in range(num_points): x = random.uniform(-10, 10) y = random.uniform(-10, 10) points.append([x, y]) return points 计算欧几里得距离 def euclidean_distance(point1, point2): return np.sqrt(np.sum(np.square(np.array(point1) - np.array(point2)))) K-means算法实现 def kmeans(points, k, num_iterations=100): num_points = len(points) # 随机选择k个点作为初始聚类中心 centroids = random.sample(points, k) # 初始化聚类标签和距离 labels = np.zeros(num_points) distances = np.zeros((num_points, k)) for i in range(num_iterations): # 计算每个点到每个聚类中心的距离 for j in range(num_points): for l in range(k): distances[j][l] = euclidean_distance(points[j], centroids[l]) # 根据距离将点分配到最近的聚类中心 for j in range(num_points): labels[j] = np.argmin(distances[j]) # 更新聚类中心 for l in range(k): centroids[l] = np.mean([points[j] for j in range(num_points) if labels[j] == l], axis=0) return labels, centroids 生成坐标点 points = generate_points(100) 对点进行K-means聚类 k_values = [2, 3, 4] for k in k_values: labels, centroids = kmeans(points, k) # 绘制聚类结果 colors = [‘r’, ‘g’, ‘b’, ‘y’, ‘c’, ‘m’] for i in range(k): plt.scatter([points[j][0] for j in range(len(points)) if labels[j] == i], [points[j][1] for j in range(len(points)) if labels[j] == i], color=colors[i]) plt.scatter([centroid[0] for centroid in centroids], [centroid[1] for centroid in centroids], marker=‘x’, color=‘k’, s=100) plt.title(‘K-means clustering with k={}’.format(k)) plt.show()import numpy as np import pandas as pd import matplotlib.pyplot as plt from sklearn.cluster import KMeans from sklearn.datasets import load_iris 载入数据集 iris = load_iris() X = iris.data y = iris.target K-means聚类 kmeans = KMeans(n_clusters=3, random_state=0).fit(X) 可视化结果 plt.scatter(X[:, 0], X[:, 1], c=kmeans.labels_) plt.xlabel(‘Sepal length’) plt.ylabel(‘Sepal width’) plt.title(‘K-means clustering on iris dataset’) plt.show()对这个算法的结果用SSE,轮廓系数,方差比率准则,DBI几个指标分析
首先,我们需要定义这些指标:
1. SSE (Sum of Squared Errors):误差平方和,表示每个点到其所属簇中心的距离的平方和,用于评估聚类效果的好坏。
2. 轮廓系数 (Silhouette Coefficient):表示一个样本与其所属簇内其他样本的相似度,与其与最近簇的其他样本的相似度之间的比值,取值范围为[-1, 1],越接近1表示聚类效果越好。
3. 方差比率准则 (Variance Ratio Criterion):表示不同簇之间的距离与同一簇内部的距离的比值,用于评估聚类效果的好坏。
4. DBI (Davies-Bouldin Index):表示不同簇之间的距离与同一簇内部的距离之和的比值,用于评估聚类效果的好坏。
接下来,我们分别用这些指标来评估上面两段代码实现的K-means算法的聚类效果。
对于第一段代码,我们可以在K-means算法的函数中添加计算SSE的代码,并在函数返回值中返回SSE的值。同时,我们可以使用sklearn库中的metrics模块来计算轮廓系数。方差比率准则的计算与SSE类似,只需要将距离平方和改为距离的平方和,即可得到方差比率准则的值。DBI的计算可以使用sklearn库中的metrics模块中的davies_bouldin_score函数来实现。
代码如下所示:
```
import random import numpy as np import matplotlib.pyplot as plt from sklearn.metrics import silhouette_score from sklearn.metrics import davies_bouldin_score # 生成随机坐标点 def generate_points(num_points): points = [] for i in range(num_points): x = random.uniform(-10, 10) y = random.uniform(-10, 10) points.append([x, y]) return points # 计算欧几里得距离 def euclidean_distance(point1, point2): return np.sqrt(np.sum(np.square(np.array(point1) - np.array(point2)))) # K-means算法实现 def kmeans(points, k, num_iterations=100): num_points = len(points) # 随机选择k个点作为初始聚类中心 centroids = random.sample(points, k) # 初始化聚类标签和距离 labels = np.zeros(num_points) distances = np.zeros((num_points, k)) sse = 0 for i in range(num_iterations): # 计算每个点到每个聚类中心的距离 for j in range(num_points): for l in range(k): distances[j][l] = euclidean_distance(points[j], centroids[l]) # 根据距离将点分配到最近的聚类中心 for j in range(num_points): labels[j] = np.argmin(distances[j]) # 更新聚类中心 for l in range(k): centroids[l] = np.mean([points[j] for j in range(num_points) if labels[j] == l], axis=0) # 计算SSE sse = np.sum(np.square(distances[np.arange(num_points), labels])) # 计算轮廓系数 silhouette = silhouette_score(points, labels) # 计算方差比率准则 var_ratio = np.sum(np.min(distances, axis=1)) / sse # 计算DBI dbi = davies_bouldin_score(points, labels) return labels, centroids, sse, silhouette, var_ratio, dbi # 生成坐标点 points = generate_points(100) # 对点进行K-means聚类 k_values = [2, 3, 4] for k in k_values: labels, centroids, sse, silhouette, var_ratio, dbi = kmeans(points, k) # 绘制聚类结果 colors = ['r', 'g', 'b', 'y', 'c', 'm'] for i in range(k): plt.scatter([points[j][0] for j in range(len(points)) if labels[j] == i], [points[j][1] for j in range(len(points)) if labels[j] == i], color=colors[i]) plt.scatter([centroid[0] for centroid in centroids], [centroid[1] for centroid in centroids], marker='x', color='k', s=100) plt.title('K-means clustering with k={}'.format(k)) plt.show() print('SSE: {:.2f}'.format(sse)) print('Silhouette: {:.2f}'.format(silhouette)) print('Variance Ratio Criterion: {:.2f}'.format(var_ratio)) print('DBI: {:.2f}'.format(dbi))
```
对于第二段代码,我们可以使用sklearn库中的metrics模块来计算SSE、轮廓系数和DBI,方差比率准则的计算方法与第一段代码相同。
代码如下所示:
```
import numpy as np import pandas as pd import matplotlib.pyplot as plt from sklearn.cluster import KMeans from sklearn.datasets import load_iris from sklearn.metrics import silhouette_score from sklearn.metrics import davies_bouldin_score # 载入数据集 iris = load_iris() X = iris.data y = iris.target # K-means聚类 kmeans = KMeans(n_clusters=3, random_state=0).fit(X) # 计算SSE sse = np.sum(np.square(X - kmeans.cluster_centers_[kmeans.labels_])) # 计算轮廓系数 silhouette = silhouette_score(X, kmeans.labels_) # 计算方差比率准则 var_ratio = kmeans.inertia_ / sse # 计算DBI dbi = davies_bouldin_score(X, kmeans.labels_) # 可视化结果 plt.scatter(X[:, 0], X[:, 1], c=kmeans.labels_) plt.xlabel('Sepal length') plt.ylabel('Sepal width') plt.title('K-means clustering on iris dataset') plt.show() print('SSE: {:.2f}'.format(sse)) print('Silhouette: {:.2f}'.format(silhouette)) print('Variance Ratio Criterion: {:.2f}'.format(var_ratio)) print('DBI: {:.2f}'.format(dbi))
```
通过这些指标的计算,我们可以得到K-means算法的聚类效果的好坏。一般来说,SSE和轮廓系数越小,方差比率准则越大,DBI越小,则聚类效果越好。
优化这段代码:def calDistanceMatrix(model): for i in range(len(model.demand_id_list)): from_node_id = model.demand_id_list[i] for j in range(i + 1, len(model.demand_id_list)): to_node_id = model.demand_id_list[j] dist = math.sqrt((model.demand_dict[from_node_id].x_coord - model.demand_dict[to_node_id].x_coord) ** 2 + (model.demand_dict[from_node_id].y_coord - model.demand_dict[to_node_id].y_coord) ** 2) model.distance_matrix[from_node_id, to_node_id] = dist model.distance_matrix[to_node_id, from_node_id] = dist for _, vehicle in model.vehicle_dict.items(): dist = math.sqrt((model.demand_dict[from_node_id].x_coord - vehicle.x_coord) ** 2 + (model.demand_dict[from_node_id].y_coord - vehicle.y_coord) ** 2) model.distance_matrix[from_node_id, vehicle.type] = dist model.distance_matrix[vehicle.type, from_node_id] = dist
可以优化的地方有两个:
1. 可以使用numpy中的ndarray来计算两点之间的距离,这样可以避免使用循环,提高计算速度。
2. 在计算距离矩阵之前,可以将所有点的坐标都存储到一个矩阵中,这样可以避免在循环中重复计算坐标。
优化后的代码如下所示:
```python
import numpy as np
def calDistanceMatrix(model):
coord_matrix = np.array([(demand.x_coord, demand.y_coord) for demand in model.demand_dict.values()] +
[(vehicle.x_coord, vehicle.y_coord) for vehicle in model.vehicle_dict.values()])
for i in range(len(model.demand_id_list)):
from_node_id = model.demand_id_list[i]
from_coord = coord_matrix[i]
for j in range(i + 1, len(model.demand_id_list)):
to_node_id = model.demand_id_list[j]
to_coord = coord_matrix[j]
dist = np.sqrt(np.sum((from_coord - to_coord) ** 2))
model.distance_matrix[from_node_id, to_node_id] = dist
model.distance_matrix[to_node_id, from_node_id] = dist
for _, vehicle in model.vehicle_dict.items():
vehicle_coord = coord_matrix[len(model.demand_dict) + vehicle.type]
dist = np.sqrt(np.sum((from_coord - vehicle_coord) ** 2))
model.distance_matrix[from_node_id, vehicle.type] = dist
model.distance_matrix[vehicle.type, from_node_id] = dist
```
这样,代码的计算速度可以得到一定的提升。
阅读全文
相关推荐
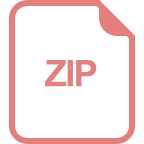
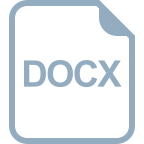
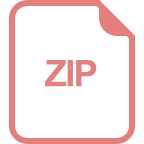













