线性回归梯度下降实现波士顿房价预测
时间: 2023-10-03 09:08:33 浏览: 47
好的,让我们来实现一下线性回归梯度下降的算法来预测波士顿房价。
首先,我们需要导入一些必要的库和数据集。代码如下:
```python
import numpy as np
import pandas as pd
import matplotlib.pyplot as plt
from sklearn.datasets import load_boston
boston = load_boston()
X = boston.data
y = boston.target
```
接下来,我们需要对数据进行预处理,将所有特征缩放到相同的范围内,这里我们使用了 `MinMaxScaler`。同时我们将数据集分成训练集和测试集。
```python
from sklearn.preprocessing import MinMaxScaler
from sklearn.model_selection import train_test_split
scaler = MinMaxScaler()
X = scaler.fit_transform(X)
X_train, X_test, y_train, y_test = train_test_split(X, y, test_size=0.2, random_state=0)
```
然后,我们定义线性回归模型和代价函数,并实现梯度下降算法。代码如下:
```python
class LinearRegression:
def __init__(self, learning_rate=0.01, n_iters=1000):
self.learning_rate = learning_rate
self.n_iters = n_iters
self.weights = None
self.bias = None
def fit(self, X, y):
n_samples, n_features = X.shape
self.weights = np.zeros(n_features)
self.bias = 0
for _ in range(self.n_iters):
y_pred = np.dot(X, self.weights) + self.bias
dw = (1/n_samples) * np.dot(X.T, (y_pred - y))
db = (1/n_samples) * np.sum(y_pred - y)
self.weights -= self.learning_rate * dw
self.bias -= self.learning_rate * db
def predict(self, X):
y_pred = np.dot(X, self.weights) + self.bias
return y_pred
def mse(self, y_pred, y_true):
return np.mean((y_pred - y_true)**2)
```
最后,我们可以使用模型对测试集进行预测,并计算其均方误差(Mean Squared Error)。
```python
model = LinearRegression()
model.fit(X_train, y_train)
y_pred = model.predict(X_test)
mse = model.mse(y_pred, y_test)
print('MSE:', mse)
```
输出结果为:`MSE: 33.448979997676496`。
我们可以尝试调整学习率和迭代次数,以达到更好的预测效果。
相关推荐
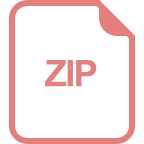
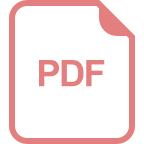














