Point transformer与point transformerv2
时间: 2023-09-30 21:04:40 浏览: 166
Point Transformer与Point Transformer V2是一种用于点云数据处理的模型。Point Transformer是Point Transformer的缩写,而Point Transformer V2是对Point Transformer进行升级改进的版本。
Point Transformer V2的改进主要集中在Attention算子和池化策略上。在Attention算子方面,作者通过引入分组向量注意公式(GVC)来减少参数数量,并通过增加位置编码来改进向量注意的泛化能力。GVC将向量注意分为具有共享注意权重的组,减少了参数的数量,并提高了模型的可用性。此外,通过增加哈达玛积乘积位置编码乘数,可以加强位置编码,使模型更加专注于学习复杂点云的位置关系。
Point Transformer V2的改进使得模型在处理点云数据时具有更高的精度和可用性。它是一种先进的模型,在文档理解、视觉文档分类以及信息提取等任务上都表现出了优异的性能。
总结来说,Point Transformer是初始版本,而Point Transformer V2是对其进行改进的版本,通过改进Attention算子和池化策略,提高了模型的性能和可用性。
相关问题
point transformer v2
Point Transformer v2 is an extension of the original Point Transformer model, which is a neural network architecture designed for point cloud processing tasks, such as 3D object recognition and segmentation. Point Transformer v2 incorporates several improvements over the original model, including:
1. Attention-based feature propagation: Instead of using traditional convolutional operations, Point Transformer v2 uses attention mechanisms to propagate features across points in the point cloud. This allows the model to capture long-range dependencies and spatial relationships between points in a more flexible way.
2. Multi-head attention: Point Transformer v2 uses multi-head attention to allow the model to attend to multiple aspects of the input at once. This leads to improved performance on complex tasks that require the model to reason about multiple levels of abstraction.
3. Dynamic graph generation: Instead of using a fixed graph structure to represent the input point cloud, Point Transformer v2 generates a dynamic graph at runtime based on the input features. This allows the model to adapt to the local geometry of the point cloud and capture more fine-grained details.
Overall, Point Transformer v2 is a powerful neural network architecture for point cloud processing tasks, and has achieved state-of-the-art results on several benchmark datasets.
Point Transformer V2
Point Transformer V2 是一个基于 Transformer 模型的点云处理算法的改进版本。它主要用于处理点云数据,其中点云是由一系列的点组成的三维数据。Point Transformer V2 使用了自注意力机制来捕捉点云数据中的空间关系和语义信息,从而在点云处理任务上取得了很好的效果。
该算法的改进之处在于引入了可变形卷积(deformable convolution)和采样一致性(sampling consistency)机制。可变形卷积能够自适应地对输入进行采样和变换,从而更好地建模局部区域的特征。采样一致性机制则用于保持点云数据的一致性,使得模型能够处理具有不同密度的点云。
通过这些改进,Point Transformer V2 在点云分割、点云分类和点云生成等任务上都取得了较好的性能。它为点云处理提供了一个强大且高效的解决方案,可以广泛应用于计算机视觉、机器人学和自动驾驶等领域。
阅读全文
相关推荐
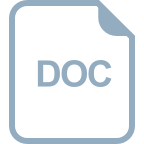
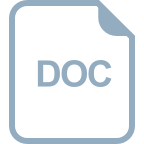
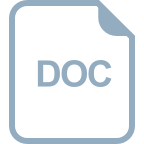
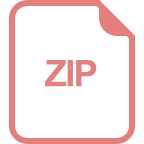












