def generate_individual(): return random.uniform(-10, 10)
时间: 2023-11-25 17:03:38 浏览: 31
函数generate_individual()的作用是生成一个随机的浮点数,范围在-10到10之间。这个函数利用了random模块中的uniform()方法,该方法可以生成指定范围内的随机浮点数。调用generate_individual()函数时,会返回一个在-10到10之间的随机浮点数。这个函数可以用于遗传算法中个体的初始化,也可以用于各种需要随机生成浮点数的场景。例如,可以将生成的随机浮点数作为某个问题的初始解,或者用于随机初始化神经网络的权重和偏置等。总之,generate_individual()函数是一个简单但非常有用的工具函数,可以帮助我们快速生成指定范围内的随机浮点数。
相关问题
Traceback (most recent call last): File "E:\Duzhuan\anaconda\lib\site-packages\IPython\core\interactiveshell.py", line 3460, in run_code exec(code_obj, self.user_global_ns, self.user_ns) File "<ipython-input-2-c833f4fdcae2>", line 1, in <module> runfile('C:\\Users\\zhangxiao\\Desktop\\Algorithm Integration\\apc_1.py', wdir='C:\\Users\\zhangxiao\\Desktop\\Algorithm Integration') File "E:\Duzhuan\JetBrainsPyCharm 2023.1\PyCharm 2023.1\plugins\python\helpers\pydev\_pydev_bundle\pydev_umd.py", line 198, in runfile pydev_imports.execfile(filename, global_vars, local_vars) # execute the script File "E:\Duzhuan\JetBrainsPyCharm 2023.1\PyCharm 2023.1\plugins\python\helpers\pydev\_pydev_imps\_pydev_execfile.py", line 18, in execfile exec(compile(contents+"\n", file, 'exec'), glob, loc) File "C:\Users\zhangxiao\Desktop\Algorithm Integration\apc_1.py", line 189, in <module> integrated_optimization() File "C:\Users\zhangxiao\Desktop\Algorithm Integration\apc_1.py", line 177, in integrated_optimization x_after, y_after = final_solution[i][0], final_solution[i][1] IndexError: invalid index to scalar variable.
这个错误是由于 `final_solution` 是一个标量变量,无法进行索引操作。在集成优化框架的代码中,`final_solution` 应该是一个包含多个传感器位置的列表,而不是单个位置变量。为了解决这个问题,您可以检查粒子群优化算法的实现,并确保它返回一个包含多个传感器位置的列表。以下是修改后的代码示例:
```python
# 粒子群优化算法
def particle_swarm_optimization(obj_func, num_particles, num_iterations):
positions = np.random.uniform(low=0, high=1, size=(num_particles, 2))
velocities = np.random.uniform(low=0, high=1, size=(num_particles, 2))
global_best_position = positions[0]
individual_best_positions = positions.copy()
for _ in range(num_iterations):
inertia_weight = 0.8
cognitive_weight = 1.5
social_weight = 1.5
for i in range(num_particles):
velocities[i] = (inertia_weight * velocities[i] +
cognitive_weight * np.random.uniform(0, 1) * (individual_best_positions[i] - positions[i]) +
social_weight * np.random.uniform(0, 1) * (global_best_position - positions[i]))
positions[i] += velocities[i]
for i in range(num_particles):
if obj_func(positions[i]) < obj_func(individual_best_positions[i]):
individual_best_positions[i] = positions[i]
if obj_func(positions[i]) < obj_func(global_best_position):
global_best_position = positions[i]
return individual_best_positions
# 使用集成框架进行优化
def integrated_optimization():
num_sensors = 100
num_anchors = 30
num_nests = 10
num_generations = 10
num_particles = 10
# 生成传感器节点
sensors_before = generate_sensors(num_sensors, num_anchors)
# 使用布谷鸟算法进行全局搜索
global_best_nest = cuckoo_search(objective_function, num_nests, num_generations)
# 使用遗传算法进行局部搜索
local_best_individual = genetic_algorithm(objective_function, num_nests, num_generations)
# 使用粒子群优化算法进行微调
final_solution = particle_swarm_optimization(objective_function, num_particles, num_generations)
# 更新传感器位置
sensors_after = []
for i in range(num_sensors):
x_before, y_before, is_anchor = sensors_before[i]
x_after, y_after = final_solution[i]
sensors_after.append((x_after * 100, y_after * 100, is_anchor))
# 计算定位误差
error = compute_localization_error(sensors_before, sensors_after)
# 绘制节点分布图
plot_sensor_locations(sensors_before, sensors_after)
print("Localization error:", error)
# 运行集成优化框架
integrated_optimization()
```
请尝试运行这段代码,看看是否解决了问题。如果还有其他问题,请随时提问。
帮我用python语言编写一段遗传算法代码
好的,我会为您提供关于遗传算法的代码实现。以下是一个简单的遗传算法的示例,用于求解一个函数的最优解。在这个示例中,我们将最小化函数 f(x) = x^2,将个体定义为实数,最大进化代数为100,种群大小为100,交叉概率为0.8,变异概率为0.1。
```python
import random
def fitness(individual):
return individual**2
def generate_population(pop_size):
population = []
for i in range(pop_size):
population.append(random.uniform(-10, 10))
return population
def selection(population):
selected = random.sample(population, 2)
return sorted(selected, key=lambda x: fitness(x))[0]
def crossover(parent_1, parent_2):
if random.random() < crossover_prob:
return (parent_1 + parent_2) / 2
else:
return parent_1
def mutate(individual):
if random.random() < mutation_prob:
return individual + random.uniform(-1, 1)
else:
return individual
def genetic_algorithm(pop_size, gens):
population = generate_population(pop_size)
for i in range(gens):
new_population = []
for j in range(pop_size):
parent_1 = selection(population)
parent_2 = selection(population)
offspring = crossover(parent_1, parent_2)
offspring = mutate(offspring)
new_population.append(offspring)
population = new_population
return min(population, key=lambda x: fitness(x))
if __name__ == '__main__':
pop_size = 100
gens = 100
crossover_prob = 0.8
mutation_prob = 0.1
result = genetic_algorithm(pop_size, gens)
print("最优解为:", result)
```
以上是一个遗传算法的简单实现,您可以根据自己的需求修改代码并调整参数。希望能对您有所帮助!
相关推荐
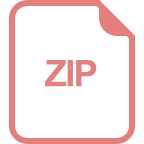
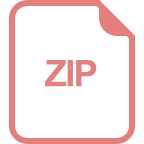












