df['trade_date']= convert_date_from_int(df['trade_date']) df.index = df['trade_date']出现错误ValueError: time data '20230428' does not match format '%Y-%m-%d'怎么解决
时间: 2023-08-15 09:08:19 浏览: 137
根据错误提示,你提供的时间格式`'%Y-%m-%d'`与数据中的时间格式`'20230428'`不匹配。因此,你需要更改转换日期的方式,以匹配数据中的时间格式。
你可以使用datetime库中的strptime方法将字符串转换为日期对象,然后再将其转换为所需的格式。例如,如果你的数据中的日期格式是`'%Y%m%d'`,则可以使用以下代码:
```python
from datetime import datetime
# 定义旧日期格式
OLD_FORMAT = '%Y%m%d'
# 将日期列转换为日期对象
df['trade_date'] = df['trade_date'].apply(lambda x: datetime.strptime(str(x), OLD_FORMAT))
# 将日期列转换为新格式
NEW_FORMAT = '%Y-%m-%d'
df['trade_date'] = df['trade_date'].apply(lambda x: datetime.strftime(x, NEW_FORMAT))
# 将日期列设置为索引
df.index = df['trade_date']
```
使用上述代码,你可以将数据中的日期字符串转换为指定的日期格式,并将其设置为DataFrame的索引,以便更轻松地进行时间序列分析。
相关问题
param = {'num_leaves': 31, 'min_data_in_leaf': 20, 'objective': 'binary', 'learning_rate': 0.06, "boosting": "gbdt", "metric": 'None', "verbosity": -1} trn_data = lgb.Dataset(trn, trn_label) val_data = lgb.Dataset(val, val_label) num_round = 666 # clf = lgb.train(param, trn_data, num_round, valid_sets=[trn_data, val_data], verbose_eval=100, # early_stopping_rounds=300, feval=win_score_eval) clf = lgb.train(param, trn_data, num_round) # oof_lgb = clf.predict(val, num_iteration=clf.best_iteration) test_lgb = clf.predict(test, num_iteration=clf.best_iteration)thresh_hold = 0.5 oof_test_final = test_lgb >= thresh_hold print(metrics.accuracy_score(test_label, oof_test_final)) print(metrics.confusion_matrix(test_label, oof_test_final)) tp = np.sum(((oof_test_final == 1) & (test_label == 1))) pp = np.sum(oof_test_final == 1) print('accuracy1:%.3f'% (tp/(pp)))test_postive_idx = np.argwhere(oof_test_final == True).reshape(-1) # test_postive_idx = list(range(len(oof_test_final))) test_all_idx = np.argwhere(np.array(test_data_idx)).reshape(-1) stock_info['trade_date_id'] = stock_info['trade_date'].map(date_map) stock_info['trade_date_id'] = stock_info['trade_date_id'] + 1tmp_col = ['ts_code', 'trade_date', 'trade_date_id', 'open', 'high', 'low', 'close', 'ma5', 'ma13', 'ma21', 'label_final', 'name'] stock_info.iloc[test_all_idx[test_postive_idx]] tmp_df = stock_info[tmp_col].iloc[test_all_idx[test_postive_idx]].reset_index() tmp_df['label_prob'] = test_lgb[test_postive_idx] tmp_df['is_limit_up'] = tmp_df['close'] == tmp_df['high'] buy_df = tmp_df[(tmp_df['is_limit_up']==False)].reset_index() buy_df.drop(['index', 'level_0'], axis=1, inplace=True)buy_df['buy_flag'] = 1 stock_info_copy['sell_flag'] = 0tmp_idx = (index_df['trade_date'] == test_date_min+1) close1 = index_df[tmp_idx]['close'].values[0] test_date_max = 20220829 tmp_idx = (index_df['trade_date'] == test_date_max) close2 = index_df[tmp_idx]['close'].values[0]tmp_idx = (stock_info_copy['trade_date'] >= test_date_min) & (stock_info_copy['trade_date'] <= test_date_max) tmp_df = stock_info_copy[tmp_idx].reset_index(drop=True)from imp import reload import Account reload(Account) money_init = 200000 account = Account.Account(money_init, max_hold_period=20, stop_loss_rate=-0.07, stop_profit_rate=0.12) account.BackTest(buy_df, tmp_df, index_df, buy_price='open')tmp_df2 = buy_df[['ts_code', 'trade_date', 'label_prob', 'label_final']] tmp_df2 = tmp_df2.rename(columns={'trade_date':'buy_date'}) tmp_df = account.info tmp_df['buy_date'] = tmp_df['buy_date'].apply(lambda x: int(x)) tmp_df = tmp_df.merge(tmp_df2, on=['ts_code', 'buy_date'], how='left')最终的tmp_df是什么?tmp_df[tmp_df['label_final']==1]又选取了什么股票?
根据代码逐行分析,tmp_df 是一个 DataFrame,包含了股票的信息以及回测结果。其中,选取了 label_final 为 1 的股票,也就是模型预测为涨的股票,并且过滤掉了当天涨停的股票。最终买入的股票信息保存在 buy_df 中,回测时将其与 stock_info_copy、index_df 进行合并,得到了回测的结果,并将买入的股票信息与回测结果合并,生成了最终的 tmp_df。
import matplotlib.pyplot as plt import pandas as pd import numpy as np import tushare as ts pro = ts.pro_api('68055e3ab5b3a9b50c8fef138c44546dbd25bea5474439a22134df3d') start_date = '20180510' end_date = '20201030' code_list = ['000568.SZ', '000858.SZ', '600519.SH', '600809.SH', '002304.SZ'] for i in code_list: df = pro.daily(ts_code=i, start_date=start_date, end_date=end_date) df = df[['ts_code','trade_date','close']] df.to_csv(f'{i}.csv',encoding='gbk') df1 = pd.read_csv('000568.SZ.csv') df2 = pd.read_csv('000858.SZ.csv') df3 = pd.read_csv('600519.SH.csv') df4 = pd.read_csv('600809.SH.csv') df5 = pd.read_csv('002304.SZ.csv') df=pd.concat([df1,df2,df3,df4,df], ignore_index=True) df['trade_date'] = pd.to_datetime(df['trade_date'], format='%Y%m%d') df['close'] = df['close'].astype(float) df = df.set_index('trade_date') returns1 = df.loc[df['ts_code'] == '000568.SZ', 'close'].pct_change() returns2 = df.loc[df['ts_code'] == '000858.SZ', 'close'].pct_change() returns3 = df.loc[df['ts_code'] == '600519.SH', 'close'].pct_change() returns4 = df.loc[df['ts_code'] == '600809.SH', 'close'].pct_change() returns5 = df.loc[df['ts_code'] == '002304.SZ', 'close'].pct_change() returns = 0.32 * returns1 + 0.15 * returns2 + 0.10 * returns3 + 0.18 * returns4 + 0.25 * returns5
你的代码是用于计算不同股票的加权平均收益率的。其中,代码列表中包含了5只股票,分别是 '000568.SZ', '000858.SZ', '600519.SH', '600809.SH', '002304.SZ',每只股票的交易数据都是从 '20180510' 到 '20201030' 的。代码首先通过 tushare 库获取每只股票的交易数据,并将其保存到相应的 csv 文件中。接着,代码使用 pandas 库将这些 csv 文件读取到 DataFrame 中,并将这些 DataFrame 合并到一起。最后,代码计算了每只股票的收益率,并按照一定的权重对这些收益率进行加权平均。
阅读全文
相关推荐
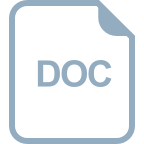
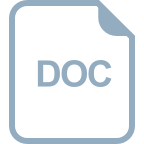
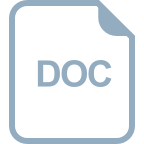













