for k, v in self.netG.named_parameters(): # can optimize for a part of the model if v.requires_grad: optim_params.append(v)解释该段代码
时间: 2023-06-09 15:01:56 浏览: 179
这段代码是用来遍历self.netG模型中的每一个参数,并将参数名和参数值分别赋值给变量k和v。其中,self.netG是一个神经网络模型的实例,named_parameters()是该模型实例的函数,返回包含该模型中所有参数的生成器。
相关问题
for p in netD.parameters(): # reset requires_grad p.requires_grad = False # avoid computation netG.zero_grad() input_attv = Variable(input_att) noise.normal_(0, 1) noisev = Variable(noise) fake = netG(noisev, input_attv) criticG_fake = netD(fake, input_attv) criticG_fake = criticG_fake.mean() G_cost = -criticG_fake # classification loss c_errG = cls_criterion(pretrain_cls.model(fake), Variable(input_label)) errG = G_cost + opt.cls_weight*c_errG errG.backward() optimizerG.step() mean_lossG /= data.ntrain / opt.batch_size mean_lossD /= data.ntrain / opt.batch_size print('[%d/%d] Loss_D: %.4f Loss_G: %.4f, Wasserstein_dist: %.4f, c_errG:%.4f' % (epoch, opt.nepoch, D_cost.data[0], G_cost.data[0], Wasserstein_D.data[0], c_errG.data[0]))
这段代码是用于训练生成器(netG)的部分。
首先,通过循环遍历判别器(netD)的参数,将它们的`requires_grad`属性设置为False,以避免进行梯度计算。
然后,通过调用`zero_grad`方法清空生成器的梯度。
接下来,创建变量input_attv和noisev,分别将输入的属性特征(input_att)和噪声(noise)转换为可求导的变量。
使用生成器netG生成合成图像fake,并将其输入到判别器netD中得到判别器对合成图像的评分criticG_fake。将criticG_fake取平均值。
计算生成器的损失函数G_cost,为负的criticG_fake。
计算分类损失函数c_errG,通过将合成图像fake输入预训练的分类器pretrain_cls.model,并与真实标签(input_label)进行比较。
计算总体损失函数errG,为G_cost加上分类损失乘以权重参数opt.cls_weight。
通过调用`backward`方法进行反向传播,计算梯度。
通过调用`step`方法执行一步优化器(optimizerG)更新生成器模型的参数。
在每个epoch的训练中,累积计算平均损失mean_lossG和mean_lossD。
最后,打印出当前epoch的损失函数值(Loss_D和Loss_G)、Wasserstein距离(Wasserstein_dist)和分类损失(c_errG)。
请注意,这段代码中引用了一些变量和模型,如netD、netG、pretrain_cls等,可能需要事先定义或导入。在实际应用中,可能需要根据具体需求对这些代码进行适当的修改和调用。
fix_fake_imgs = netg(fix_noises) for epoch in iter(epochs): for ii, (img, _) in tqdm.tqdm(enumerate(dataloader)): real_img = img.to(device) if ii % opt.d_every == 0: # 训练判别器 optimizer_d.zero_grad() ## 尽可能的把真图片判别为正确 output = netd(real_img) error_d_real = criterion(output, true_labels) error_d_real.backward() ## 尽可能把假图片判别为错误 noises.data.copy_(t.randn(opt.batch_size, opt.nz, 1, 1)) fake_img = netg(noises).detach() # 根据噪声生成假图 output = netd(fake_img) error_d_fake = criterion(output, fake_labels) error_d_fake.backward() optimizer_d.step() error_d = error_d_fake + error_d_real errord_meter.add(error_d.item())的含义
这段代码是一个训练GAN(生成对抗网络)的代码,其中包含了判别器的训练过程。GAN包含两个网络:生成器和判别器。生成器负责生成假图片,判别器负责判断一张图片是真实还是假的。训练过程中,首先将真实图片输入判别器,并将输出与真实标签进行比较,计算真实图片的误差;然后将生成器生成的假图片输入判别器,并将输出与假标签进行比较,计算假图片的误差;最后将真实图片误差和假图片误差相加,用于更新判别器的权重参数。其中,true_labels和fake_labels是真实图片和假图片的标签,criterion是损失函数,optimizer_d是判别器的优化器。
阅读全文
相关推荐
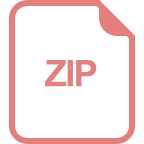
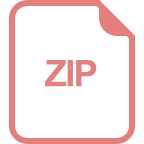














