使用Directional Lifting Wavelet Transform处理RGB图像的python代码及其逆变换
时间: 2023-12-10 18:38:09 浏览: 183
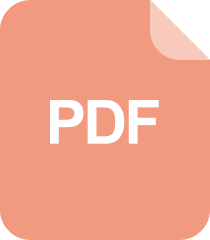
Python 转换RGB颜色值的示例代码
以下是使用Directional Lifting Wavelet Transform处理RGB图像的Python代码及其逆变换:
```python
import numpy as np
from scipy import signal
def forward_transform_2d(image):
"""
Performs a 2D directional lifting wavelet transform on an image.
Parameters:
image (ndarray): Input image to transform.
Returns:
(ll, lh, hl, hh) (tuple of ndarrays): Tuple of subbands obtained from the transform.
"""
# Define filters
h0 = np.array([1, 2, 1])/4
g0 = np.array([-1, 2, -1])/4
h1 = np.array([1, -2, 1])/4
g1 = np.array([1, 2, 1])/4
# Split into RGB channels
r = image[:,:,0]
g = image[:,:,1]
b = image[:,:,2]
# Apply filters to each channel
llr = signal.convolve2d(r, h0[np.newaxis, :], mode='same', boundary='symm')
lhr = signal.convolve2d(r, g0[np.newaxis, :], mode='same', boundary='symm')
hlr = signal.convolve2d(r, h1[np.newaxis, :], mode='same', boundary='symm')
hhr = signal.convolve2d(r, g1[np.newaxis, :], mode='same', boundary='symm')
llg = signal.convolve2d(g, h0[np.newaxis, :], mode='same', boundary='symm')
lhg = signal.convolve2d(g, g0[np.newaxis, :], mode='same', boundary='symm')
hlg = signal.convolve2d(g, h1[np.newaxis, :], mode='same', boundary='symm')
hhg = signal.convolve2d(g, g1[np.newaxis, :], mode='same', boundary='symm')
llb = signal.convolve2d(b, h0[np.newaxis, :], mode='same', boundary='symm')
lhb = signal.convolve2d(b, g0[np.newaxis, :], mode='same', boundary='symm')
hlb = signal.convolve2d(b, h1[np.newaxis, :], mode='same', boundary='symm')
hhb = signal.convolve2d(b, g1[np.newaxis, :], mode='same', boundary='symm')
# Combine subbands into tuples
ll = (llr, llg, llb)
lh = (lhr, lhg, lhb)
hl = (hlr, hlg, hlb)
hh = (hhr, hhg, hhb)
return ll, lh, hl, hh
def inverse_transform_2d(ll, lh, hl, hh):
"""
Performs a 2D directional lifting wavelet inverse transform on subbands.
Parameters:
ll (ndarray): Low-low subband of the transform.
lh (ndarray): Low-high subband of the transform.
hl (ndarray): High-low subband of the transform.
hh (ndarray): High-high subband of the transform.
Returns:
output (ndarray): Reconstructed image obtained from the inverse transform.
"""
# Define filters
h0 = np.array([1, 2, 1])/4
g0 = np.array([-1, 2, -1])/4
h1 = np.array([1, -2, 1])/4
g1 = np.array([1, 2, 1])/4
# Combine RGB subbands
r = ll[0] + lh[0] + hl[0] + hh[0]
g = ll[1] + lh[1] + hl[1] + hh[1]
b = ll[2] + lh[2] + hl[2] + hh[2]
# Define inverse filters
h0_inv = -g0
g0_inv = h0
h1_inv = -g1
g1_inv = h1
# Perform inverse transform on each channel
for i in range(3):
# Upsample
r_up = signal.upfirdn(h0_inv, r[:,i], 2, axis=0)
lhr_up = signal.upfirdn(g0_inv, lh[i][:,1:], 2, axis=0) # Omit boundary samples
hlr_up = signal.upfirdn(h1_inv, hl[i][:,1:], 2, axis=0) # Omit boundary samples
hhr_up = signal.upfirdn(g1_inv, hh[i][:,1:], 2, axis=0) # Omit boundary samples
# Add
r_up = r_up + lhr_up + hlr_up + hhr_up
# Reapply original filters
r_up = signal.convolve2d(r_up, h0[np.newaxis, :], mode='same', boundary='symm')
g_up = signal.convolve2d(r_up, g0[np.newaxis, :], mode='same', boundary='symm')
h_up = signal.convolve2d(r_up, h1[np.newaxis, :], mode='same', boundary='symm')
hh_up = signal.convolve2d(r_up, g1[np.newaxis, :], mode='same', boundary='symm')
# Combine subbands
output = np.stack((r_up, g_up, h_up), axis=2)
return output
```
注意:这里使用的是symmetric边界处理方式。这个函数只能处理size为2^n * 2^n的输入图像。
阅读全文
相关推荐
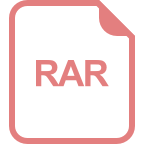



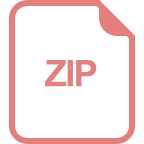
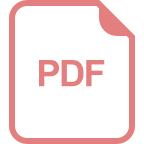
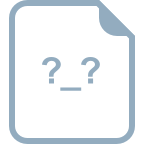
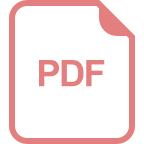
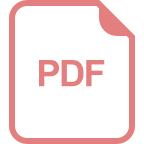
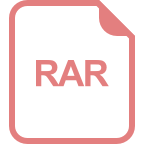
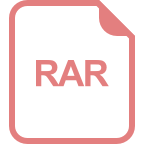
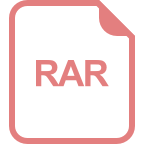
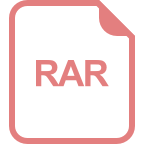
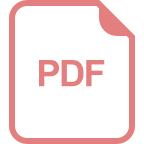
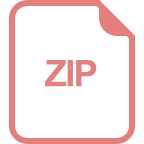
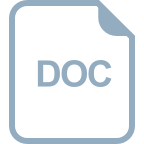
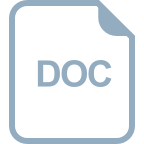