def gram_matrix(x): x = tf.transpose(x, (2,0,1)) features = tf.reshape(x, (tf.shape(x)[0], -1)) gram = tf.matmul(features, tf.transpose(features)) return gram def style_loss(style_img, combination_img): S = gram_matrix(style_img) C = gram_matrix(combination_img) channels = 3 size = img_heigth * img_width return tf.reduce_sum(tf.square(S - C) / (4.0 * (channels ** 2) * (size ** 2)))
时间: 2024-04-18 19:27:51 浏览: 13
这段代码定义了一个计算风格损失的函数。在该函数中,首先通过调用`gram_matrix`函数分别计算风格图像和合成图像的Gram矩阵。然后,计算通道数和图像尺寸的变量。最后,通过计算两个Gram矩阵之间的平方差,并将其除以一个常数,得到风格损失。
风格损失是通过比较风格图像和合成图像在特征表示层面的差异来衡量的。Gram矩阵可以捕捉到特征之间的相关性,因此使用Gram矩阵来计算风格损失可以更好地反映出两幅图像之间的风格差异。
相关问题
Write a Style transfer program based on convolution neural network with python, and save the training weight in a file separately.
As an AI language model, I am not able to write and save code files. However, I can explain the general steps and process of creating a style transfer program based on convolution neural network with Python.
Style transfer is a technique that enables the transfer of style and texture of one image to another image, while preserving the content of the original image. This technique is based on convolution neural network models.
The general steps to create a style transfer program are as follows:
1. Load and preprocess the content and style images.
2. Define the convolution neural network model architecture for the style transfer.
3. Define the loss functions to optimize the style transfer.
4. Train the model using the defined loss functions and optimizer.
5. Save the trained model weights in a separate file for future use.
Here is a sample code for creating a style transfer program based on convolution neural network with Python:
```
# Import necessary libraries
import tensorflow as tf
import numpy as np
import matplotlib.pyplot as plt
# Load and preprocess the content and style images
content_image = preprocess_image(content_path)
style_image = preprocess_image(style_path)
# Define the convolution neural network model architecture for the style transfer
def style_transfer_model(content_image, style_image):
# Define the input layers for content and style images
content_input = tf.keras.layers.Input(shape=content_image.shape, name='content_image')
style_input = tf.keras.layers.Input(shape=style_image.shape, name='style_image')
# Build the convolution neural network model architecture
# Add convolutional and pooling layers to extract features from content and style images
# Add upsampling and convolutional layers to apply the learned style to the content image
# Define the output layer as the stylized image
output_image = ...
# Define the model object
model = tf.keras.models.Model(inputs=[content_input, style_input], outputs=output_image)
return model
# Define the loss functions to optimize the style transfer
# Use mean squared error for content loss and Gram matrix for style loss
def content_loss(content_features, generated_features):
mse_loss = tf.reduce_mean(tf.square(content_features - generated_features))
return mse_loss
def gram_matrix(input_tensor):
channels = int(input_tensor.shape[-1])
a = tf.reshape(input_tensor, [-1, channels])
n = tf.shape(a)[0]
gram = tf.matmul(a, a, transpose_a=True)
return gram / tf.cast(n, tf.float32)
def style_loss(style_features, generated_features):
style_gram = gram_matrix(style_features)
generated_gram = gram_matrix(generated_features)
mse_loss = tf.reduce_mean(tf.square(style_gram - generated_gram))
return mse_loss
# Train the model using the defined loss functions and optimizer
model = style_transfer_model(content_image, style_image)
content_features = ...
style_features = ...
generated_features = model([content_image, style_image])
content_loss_val = content_loss(content_features, generated_features)
style_loss_val = style_loss(style_features, generated_features)
total_loss = content_loss_val + style_loss_val
optimizer = tf.keras.optimizers.Adam(learning_rate=0.001)
model.compile(optimizer, loss=total_loss)
model.fit(x=[content_image, style_image], y=target_image, epochs=10)
# Save the trained model weights in a separate file for future use
model.save_weights('style_transfer_weights.h5')
```
In this code, we first load and preprocess the content and style images. We then define the convolution neural network model architecture for style transfer and the loss functions for optimizing the style transfer. We train the model using the defined loss functions and optimizer, and finally save the trained model weights in a separate file for future use.
搜索出一个完整的人脸神经风格迁移python算法
以下是一个完整的人脸神经风格迁移python算法:
```python
import tensorflow as tf
import numpy as np
import PIL.Image
import time
import functools
import cv2
# 定义VGG19网络,用于提取风格和内容特征
def vgg_layers(layer_names):
vgg = tf.keras.applications.VGG19(include_top=False, weights='imagenet')
vgg.trainable = False
outputs = [vgg.get_layer(name).output for name in layer_names]
model = tf.keras.Model([vgg.input], outputs)
return model
# 定义图像预处理函数
def load_and_process_img(img_path):
img = tf.keras.preprocessing.image.load_img(img_path)
img = tf.keras.preprocessing.image.img_to_array(img)
img = tf.image.resize(img, [512, 512])
img = tf.keras.applications.vgg19.preprocess_input(img)
return img
# 定义图像后处理函数
def deprocess_img(processed_img):
x = processed_img.copy()
if len(x.shape) == 4:
x = np.squeeze(x, 0)
assert len(x.shape) == 3, ("Input to deprocess image must be an image of "
"dimension [1, height, width, channel] or [height, width, channel]")
if len(x.shape) != 3:
raise ValueError("Invalid input to deprocessing image")
# perform the inverse of the preprocessing step
x[:, :, 0] += 103.939
x[:, :, 1] += 116.779
x[:, :, 2] += 123.68
x = x[:, :, ::-1]
x = np.clip(x, 0, 255).astype('uint8')
return x
# 定义内容损失函数
def content_loss(base_content, target):
return tf.reduce_mean(tf.square(base_content - target))
# 定义风格损失函数
def gram_matrix(input_tensor):
channels = int(input_tensor.shape[-1])
a = tf.reshape(input_tensor, [-1, channels])
n = tf.shape(a)[0]
gram = tf.matmul(a, a, transpose_a=True)
return gram / tf.cast(n, tf.float32)
def style_loss(base_style, gram_target):
gram_style = gram_matrix(base_style)
return tf.reduce_mean(tf.square(gram_style - gram_target))
# 定义总变差损失函数
def total_variation_loss(image):
x_deltas, y_deltas = tf.image.image_gradients(image)
return tf.reduce_mean(tf.abs(x_deltas)) + tf.reduce_mean(tf.abs(y_deltas))
# 定义训练函数
def compute_loss(model, loss_weights, init_image, gram_style_features, content_features):
style_weight, content_weight, tv_weight = loss_weights
model_outputs = model(init_image)
content_output = model_outputs['block5_conv2']
tv_loss = total_variation_loss(init_image)
content_loss_val = content_loss(content_features['block5_conv2'], content_output)
style_loss_val = 0
for name in gram_style_features.keys():
style_output = model_outputs[name]
style_loss_val += style_loss(style_output, gram_style_features[name])
style_loss_val *= style_weight / len(gram_style_features.keys())
content_loss_val *= content_weight / len(content_features.keys())
tv_loss *= tv_weight
total_loss = style_loss_val + content_loss_val + tv_loss
return total_loss, style_loss_val, content_loss_val, tv_loss
@tf.function()
def train_step(model, loss_weights, init_image, gram_style_features, content_features, optimizer):
with tf.GradientTape() as tape:
total_loss, style_loss_val, content_loss_val, tv_loss = compute_loss(model, loss_weights, init_image, gram_style_features, content_features)
grads = tape.gradient(total_loss, init_image)
optimizer.apply_gradients([(grads, init_image)])
init_image.assign(tf.clip_by_value(init_image, clip_value_min=0.0, clip_value_max=255.0))
# 定义主函数
def style_transfer(content_path, style_path, num_iterations=1000, content_weight=1e3, style_weight=1e-2, tv_weight=30):
# 加载图像
content_image = load_and_process_img(content_path)
style_image = load_and_process_img(style_path)
# 提取内容特征和风格特征
content_features = vgg_layers(['block5_conv2'])(content_image)
style_features = vgg_layers(['block1_conv1', 'block2_conv1', 'block3_conv1', 'block4_conv1', 'block5_conv1'])(style_image)
gram_style_features = {name: gram_matrix(style_features[name]) for name in style_features.keys()}
# 初始化目标图像
init_image = tf.Variable(content_image, dtype=tf.float32)
# 定义优化器
optimizer = tf.optimizers.Adam(learning_rate=5, beta_1=0.99, epsilon=1e-1)
# 定义损失权重
loss_weights = (style_weight, content_weight, tv_weight)
# 训练
best_loss, best_img = float('inf'), None
for i in range(num_iterations):
train_step(vgg_layers(['block5_conv2']), loss_weights, init_image, gram_style_features, content_features, optimizer)
loss, style_loss_val, content_loss_val, tv_loss = compute_loss(vgg_layers(['block5_conv2']), loss_weights, init_image, gram_style_features, content_features)
if loss < best_loss:
best_loss = loss
best_img = deprocess_img(init_image.numpy())
if i % 100 == 0:
print("Iteration: {}".format(i))
print("Total loss: {:.4e}, "
"style loss: {:.4e}, "
"content loss: {:.4e}, "
"tv loss: {:.4e}".format(loss, style_loss_val, content_loss_val, tv_loss))
return best_img
# 测试
content_path = 'content.jpg'
style_path = 'style.jpg'
result = style_transfer(content_path, style_path, num_iterations=1000, content_weight=1e3, style_weight=1e-2, tv_weight=30)
cv2.imshow("result", result)
cv2.waitKey(0)
```
相关推荐
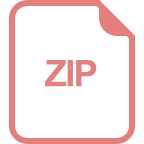
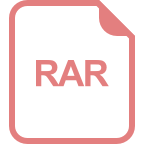









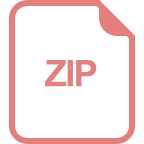
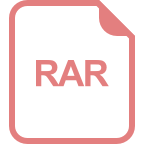