import numpy as np ; l = 100; x, y = np.indices((l, l))这段代码是什么意思
时间: 2023-06-16 22:03:47 浏览: 56
这段代码使用了NumPy库来创建了一个二维数组 x 和 y,分别表示在一个 lxl 的矩阵中,每个元素的行和列编号。
- `import numpy as np`:导入NumPy库并将其命名为np;
- `l = 100`:将l赋值为100,表示矩阵的大小为100x100;
- `x, y = np.indices((l, l))`:使用np.indices()函数创建了两个矩阵x和y,其元素的值为它们在矩阵中的行和列编号。np.indices()函数的参数为一个元组,表示矩阵的大小,这里是(l, l)表示为(100, 100)。因此,x和y的大小也是100x100。
举个例子,当l=3时,x和y的值分别为:
```
x = array([[0, 0, 0],
[1, 1, 1],
[2, 2, 2]])
y = array([[0, 1, 2],
[0, 1, 2],
[0, 1, 2]])
```
它们分别表示了一个3x3的矩阵中每个元素的行和列编号。
相关问题
给我在Python中3D的折线图,散点图,柱状图,曲面图的代码含有import numpy as np
3D折线图:
```python
import numpy as np
import matplotlib.pyplot as plt
from mpl_toolkits.mplot3d import Axes3D
# Generate some data
t = np.linspace(0, 10*np.pi, 1000)
x = np.sin(t)
y = np.cos(t)
z = t
# Create figure and 3D axis
fig = plt.figure()
ax = fig.add_subplot(111, projection='3d')
# Plot 3D line
ax.plot(x, y, z)
# Show plot
plt.show()
```
3D散点图:
```python
import numpy as np
import matplotlib.pyplot as plt
from mpl_toolkits.mplot3d import Axes3D
# Generate some data
np.random.seed(19680801)
n = 100
x, y, z = np.random.rand(3, n)
# Create figure and 3D axis
fig = plt.figure()
ax = fig.add_subplot(111, projection='3d')
# Plot 3D scatter plot
ax.scatter(x, y, z, c=z, cmap='viridis')
# Show plot
plt.show()
```
3D柱状图:
```python
import numpy as np
import matplotlib.pyplot as plt
from mpl_toolkits.mplot3d import Axes3D
# Generate some data
np.random.seed(19680801)
n = 10
x, y, z = np.indices((n, n, n))
voxels = (x < 5) & (y < 5) & (z < 5)
# Create figure and 3D axis
fig = plt.figure()
ax = fig.add_subplot(111, projection='3d')
# Plot 3D bar plot
ax.voxels(voxels)
# Show plot
plt.show()
```
3D曲面图:
```python
import numpy as np
import matplotlib.pyplot as plt
from mpl_toolkits.mplot3d import Axes3D
# Generate some data
X = np.arange(-5, 5, 0.25)
Y = np.arange(-5, 5, 0.25)
X, Y = np.meshgrid(X, Y)
R = np.sqrt(X**2 + Y**2)
Z = np.sin(R)
# Create figure and 3D axis
fig = plt.figure()
ax = fig.add_subplot(111, projection='3d')
# Plot 3D surface
ax.plot_surface(X, Y, Z)
# Show plot
plt.show()
```
import pandas as pd import numpy as np from sklearn.model_selection import train_test_split pd.set_option('display.max_columns', None) # 所有列 pd.set_option('display.max_rows', None) # 所有行 data = pd.read_excel('半监督数据.xlsx') X = data.drop(columns=['label']) # 特征矩阵 y = data['label'] # 标签列 # 划分数据集 X_train, X_test, y_train, y_test = train_test_split(X, y, test_size=0.1, stratify=None, shuffle=True, random_state=0) # 划分带标签数据集 labeled_size = 0.3 n_labeled = int(labeled_size * len(X_train)) indices = np.arange(len(X_train)) unlabeled_indices = np.delete(indices, y_train.index[:n_labeled]) X_unlabeled = X_train.iloc[unlabeled_indices] y_unlabeled = y_train.iloc[unlabeled_indices] X_labeled = X_train.iloc[y_train.index[:n_labeled]] y_labeled = y_train.iloc[y_train.index[:n_labeled]] from sklearn import preprocessing pre_transform=preprocessing.StandardScaler() pre_transform.fit(np.vstack([train_datas, test_datas])) train_datas=pre_transform.transform(train_datas) test_datas=pre_transform.transform(train_datas) from LAMDA_SSL.Algorithm.Regression.CoReg import CoReg model=CoReg() model.fit(X=train_datas,y=labeled_y,test_datas=unlabeled_X) pred_y=model.predict(X=test_X) from LAMDA_SSL.Evaluation.Regressor.Mean_Squared_Error import Mean_Squared_Error performance = Mean_Squared_Error().scoring(test_y, pred_y)帮我看一下这段代码有什么问题?怎么修改?
在代码中,预处理部分中使用了未定义的变量 `train_datas` 和 `test_datas`,应该将其改为 `X_train` 和 `X_test`。
另外,在调用 `CoReg` 模型时,传入的参数名 `test_datas` 应该改为 `unlabeled_X`,因为在之前的代码中,`X_unlabeled` 被赋值给了 `unlabeled_X`。
最后,在计算预测结果时,应该将 `test_X` 改为 `X_test`。
修改后的代码如下:
```
import pandas as pd
import numpy as np
from sklearn.model_selection import train_test_split
from sklearn import preprocessing
from LAMDA_SSL.Algorithm.Regression.CoReg import CoReg
from LAMDA_SSL.Evaluation.Regressor.Mean_Squared_Error import Mean_Squared_Error
pd.set_option('display.max_columns', None) # 所有列
pd.set_option('display.max_rows', None) # 所有行
data = pd.read_excel('半监督数据.xlsx')
X = data.drop(columns=['label']) # 特征矩阵
y = data['label'] # 标签列
# 划分数据集
X_train, X_test, y_train, y_test = train_test_split(X, y, test_size=0.1, stratify=None, shuffle=True, random_state=0)
# 划分带标签数据集
labeled_size = 0.3
n_labeled = int(labeled_size * len(X_train))
indices = np.arange(len(X_train))
unlabeled_indices = np.delete(indices, y_train.index[:n_labeled])
X_unlabeled = X_train.iloc[unlabeled_indices]
y_unlabeled = y_train.iloc[unlabeled_indices]
X_labeled = X_train.iloc[y_train.index[:n_labeled]]
y_labeled = y_train.iloc[y_train.index[:n_labeled]]
# 数据预处理
pre_transform=preprocessing.StandardScaler()
pre_transform.fit(np.vstack([X_train, X_test]))
X_train = pre_transform.transform(X_train)
X_test = pre_transform.transform(X_test)
# 构建和训练模型
model = CoReg()
model.fit(X=X_train, y=y_labeled, test_datas=X_unlabeled)
pred_y = model.predict(X=X_test)
# 计算性能指标
performance = Mean_Squared_Error().scoring(y_test, pred_y)
```
相关推荐
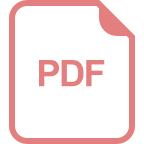
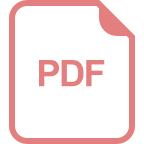
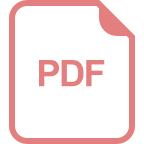













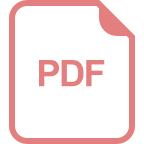