python编程实现编写有放回采样 BoostTr 划分测试集。 X_train, y_train, X_test, y_test=BoostTr(X,y,random_state)。该方法有放回采样 n 次得到训练集,n 为 X 中样本 数目。未被采样到的样本为测试样本。
时间: 2024-05-19 11:15:15 浏览: 86
这是一个关于Python编程的问题,我可以回答。实现有放回采样可以使用numpy中的random.choice()函数,代码示例如下:
import numpy as np
def BoostTr(X, y, random_state=None):
# 获取样本数目
n_samples = X.shape[0]
# 设置随机种子
np.random.seed(random_state)
# 生成n个随机数,表示有放回地从样本中采样
indices = np.random.choice(n_samples, size=n_samples, replace=True)
# 返回训练集和测试集
X_train, y_train = X[indices], y[indices]
X_test, y_test = np.delete(X, indices, axis=0), np.delete(y, indices, axis=0)
return X_train, y_train, X_test, y_test
需要注意的是,这里的X和y都是numpy数组,如果是其他数据类型需要进行相应的转换。
相关问题
2 编写有放回采样 BoostTr 划分测试集。 X_train, y_train, X_test, y_test=BoostTr(X,y,random_state)。该方法有放回采样 n 次得到训练集,n 为 X 中样本 数目。未被采样到的样本为测试样本
。代码如下:
```python
import numpy as np
def BoostTr(X, y, random_state):
np.random.seed(random_state)
n = X.shape[0]
idx = np.random.choice(n, size=n, replace=True)
X_train, y_train = X[idx], y[idx]
X_test, y_test = X[~idx], y[~idx]
return X_train, y_train, X_test, y_test
```
其中,np.random.choice() 方法用于从样本中有放回地随机抽取样本,其中 size 参数指定抽取的样本数量,replace=True 表示有放回地进行抽样。 ~idx 表示取反索引,即未被选中的样本。
def get_CIFAR10_data(num_training=500, num_validation=50, num_test=50): """ Load the CIFAR-10 dataset from disk and perform preprocessing to prepare it for classifiers. These are the same steps as we used for the SVM, but condensed to a single function. """ # Load the raw CIFAR-10 data cifar10_dir = 'C:/download/cifar-10-python/cifar-10-batches-py/data_batch_1' X_train, y_train, X_test, y_test = load_CIFAR10(cifar10_dir) print (X_train.shape) # Subsample the data mask = range(num_training, num_training + num_validation) X_val = X_train[mask] y_val = y_train[mask] mask = range(num_training) X_train = X_train[mask] y_train = y_train[mask] mask = range(num_test) X_test = X_test[mask] y_test = y_test[mask] # Normalize the data: subtract the mean image mean_image = np.mean(X_train, axis=0) X_train -= mean_image X_val -= mean_image X_test -= mean_image # Transpose so that channels come first X_train = X_train.transpose(0, 3, 1, 2).copy() X_val = X_val.transpose(0, 3, 1, 2).copy() X_test = X_test.transpose(0, 3, 1, 2).copy() # Package data into a dictionary return { 'X_train': X_train, 'y_train': y_train, 'X_val': X_val, 'y_val': y_val, 'X_test': X_test, 'y_test': y_test, }
这段代码定义了一个函数get_CIFAR10_data,用于加载和预处理CIFAR-10数据集,并返回一个包含训练集、验证集和测试集的字典。
具体来说,这个函数完成了以下几个步骤:
1. 调用load_CIFAR10函数加载CIFAR-10数据集,得到原始的训练集和测试集数据(X_train, y_train, X_test, y_test)。
2. 对原始数据进行子采样,得到num_training个样本作为训练集,num_validation个样本作为验证集,num_test个样本作为测试集。
3. 对训练集、验证集和测试集进行均值归一化,即将每个像素减去训练集的均值像素值。这个操作可以提高模型的训练效果和泛化能力。
4. 对训练集、验证集和测试集进行通道转置,即将原来的(样本数, 高, 宽, 通道数)的四维数组转换为(样本数, 通道数, 高, 宽)的四维数组。这个操作是因为在深度学习中,通常把通道数放在第二个维度上,方便卷积计算。
5. 将处理后的训练集、验证集和测试集打包成一个字典,返回给调用者。
需要注意的是,这个函数中使用了load_CIFAR10函数,也就是另一个函数,用于从文件中加载CIFAR-10数据集。另外,这里的cifar10_dir变量指定了CIFAR-10数据集的路径,需要根据实际情况进行修改。
阅读全文
相关推荐
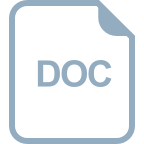
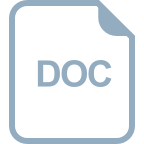
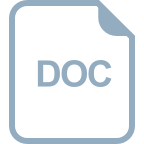













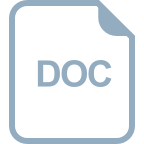