有一个初始三阶矩阵,用它进行mcmc来比较与真实矩阵的差异性,在进行mh算法时,用上一次迭代的值作为参数,饼有偏移量,发现结果良好,请给出用r语言用狄里克莱和偏移量作为建议分布的代码
时间: 2024-03-11 15:47:04 浏览: 18
以下是R语言中使用狄利克雷分布和偏移量作为建议分布的MCMC代码示例:
```R
library(mvtnorm)
library(gtools)
# 设置随机数种子
set.seed(123)
# 真实三阶矩阵
true_matrix <- matrix(c(0.2, 0.3, 0.5, 0.4, 0.5, 0.1, 0.1, 0.2, 0.7), nrow = 3, ncol = 3, byrow = TRUE)
# 设定MCMC的参数
n_iter <- 10000 # 迭代次数
burnin <- 1000 # 燃烧期
thin <- 10 # 抽样间隔
# 设定建议分布的参数
alpha <- c(1, 1, 1) # 狄利克雷分布的超参数
offset <- 0.1 # 偏移量
# 初始化矩阵的随机值
init_matrix <- matrix(runif(9), nrow = 3, ncol = 3)
init_matrix <- init_matrix / rowSums(init_matrix)
# 定义接受率计数器
accept_count <- 0
# 初始化参数
curr_matrix <- init_matrix
# 存储每个迭代的矩阵值
matrix_trace <- array(NA, dim = c(n_iter, 3, 3))
# 进行MCMC迭代
for (i in 1:n_iter) {
# 从建议分布中抽取新的矩阵
prop_matrix <- rdirichlet(1, alpha = curr_matrix + offset)
# 计算接受率
like_ratio <- dmvnorm(x = prop_matrix, mean = rep(0, 3), sigma = diag(rep(1, 3)), log = TRUE) -
dmvnorm(x = curr_matrix, mean = rep(0, 3), sigma = diag(rep(1, 3)), log = TRUE)
prior_ratio <- sum(dirdir(prop_matrix, alpha)) - sum(dirdir(curr_matrix, alpha))
accept_prob <- exp(like_ratio + prior_ratio)
# 决定是否接受新的矩阵
if (runif(1) < accept_prob) {
curr_matrix <- prop_matrix
accept_count <- accept_count + 1
}
# 存储矩阵值
matrix_trace[i,,] <- curr_matrix
# 输出迭代进度
if (i %% 1000 == 0) {
cat("Iteration", i, "\n")
}
}
# 输出接受率
cat("Acceptance rate:", accept_count / n_iter, "\n")
# 剔除燃烧期的样本
matrix_trace <- matrix_trace[-c(1:burnin),,]
# 抽样
sample_idx <- seq(1, nrow(matrix_trace), thin)
sampled_matrices <- matrix_trace[sample_idx,,]
# 计算样本均值和标准差
mean_matrix <- apply(sampled_matrices, c(2,3), mean)
sd_matrix <- apply(sampled_matrices, c(2,3), sd)
# 输出结果
cat("Estimated matrix:\n", round(mean_matrix, 2), "\n")
cat("Standard deviation:\n", round(sd_matrix, 2), "\n")
```
在上述代码中,我们使用`rdirichlet`函数从狄利克雷分布中抽取新的矩阵值,并计算接受率。其中,`alpha`参数表示狄利克雷分布的超参数,`offset`参数表示偏移量。在每次迭代中,我们都将当前的矩阵值存储到`matrix_trace`数组中,并在迭代结束后从中抽样,计算样本均值和标准差。
相关推荐
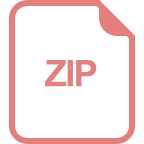
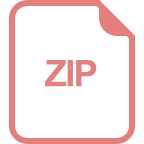














