def __init__(self, learning_rate=0.5, num_iterations=1000, regularization=None, reg_strength=0.01): self.learning_rate = learning_rate self.num_iterations = num_iterations self.regularization = regularization self.reg_strength = reg_strength self.weights = None self.bias = None的含义
时间: 2024-02-29 15:55:20 浏览: 134
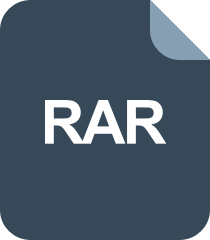
yicuansuanfa.rar_genetic iterations
这段代码是Python语言中的一个类的初始化函数,用于初始化类的属性值。该类的目的是实现逻辑回归算法。
其中,self.learning_rate表示学习率,用于控制模型学习的速度;self.num_iterations表示迭代次数,用于控制模型学习的轮数;self.regularization表示正则化方法,用于控制模型的复杂度;self.reg_strength表示正则化强度,用于控制正则化的影响程度;self.weights表示模型的权重,用于存储训练得到的权重值;self.bias表示模型的偏置,用于存储训练得到的偏置值。
在初始化函数中,我们可以通过传入参数来设置这些属性的初始值。如果没有传入参数,则使用默认值。例如,learning_rate的默认值是0.5,num_iterations的默认值是1000。在初始化函数中,我们还可以设置一些其他的属性,例如将权重和偏置初始化为None,等待训练模型后再进行设置。
阅读全文
相关推荐
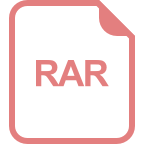
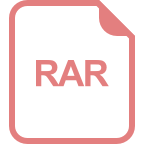
















