python之中with是什么意思?用代码距离详细说明
时间: 2023-04-08 07:02:38 浏览: 94
with 是 Python 中的一个关键字,用于创建一个上下文环境,它可以自动管理资源,例如文件、网络连接等。使用 with 语句可以确保资源在使用完毕后被正确地释放,从而避免资源泄漏和错误。
下面是一个使用 with 语句打开文件的示例代码:
```
with open('file.txt', 'r') as f:
data = f.read()
print(data)
```
在这个例子中,with 语句打开了一个名为 file.txt 的文件,并将其赋值给变量 f。在 with 块中,我们可以像普通的文件对象一样读取文件内容。当 with 块结束时,文件对象 f 会自动关闭,无需手动调用 close() 方法。
总之,with 语句可以让我们更加方便地管理资源,避免出现一些常见的错误。
相关问题
帮我用python写一个最短路径法的代码
### 回答1:
您可以参考以下python代码来实现最短路径法:# Python program for Dijkstra's single
# source shortest path algorithm. The program is
# for adjacency matrix representation of the graph # Library for INT_MAX
import sys class Graph(): def __init__(self, vertices):
self.V = vertices
self.graph = [[0 for column in range(vertices)]
for row in range(vertices)] def printSolution(self, dist):
print ("Vertex \tDistance from Source")
for node in range(self.V):
print (node, "\t", dist[node] ) # A utility function to find the vertex with
# minimum distance value, from the set of vertices
# not yet included in shortest path tree
def minDistance(self, dist, sptSet): # Initilaize minimum distance for next node
min = sys.maxsize # Search not nearest vertex not in the
# shortest path tree
for v in range(self.V):
if dist[v] < min and sptSet[v] == False:
min = dist[v]
min_index = v return min_index # Funtion that implements Dijkstra's single source
# shortest path algorithm for a graph represented
# using adjacency matrix representation
def dijkstra(self, src): dist = [sys.maxsize] * self.V
dist[src] = 0
sptSet = [False] * self.V for cout in range(self.V): # Pick the minimum distance vertex from
# the set of vertices not yet processed.
# u is always equal to src in first iteration
u = self.minDistance(dist, sptSet) # Put the minimum distance vertex in the
# shotest path tree
sptSet[u] = True # Update dist value of the adjacent vertices
# of the picked vertex only if the current
# distance is greater than new distance and
# the vertex in not in the shotest path tree
for v in range(self.V):
if self.graph[u][v] > 0 and sptSet[v] == False and \
dist[v] > dist[u] + self.graph[u][v]:
dist[v] = dist[u] + self.graph[u][v] self.printSolution(dist) # Driver program
g = Graph(9)
g.graph = [[0, 4, 0, 0, 0, 0, 0, 8, 0],
[4, 0, 8, 0, 0, 0, 0, 11, 0],
[0, 8, 0, 7, 0, 4, 0, 0, 2],
[0, 0, 7, 0, 9, 14, 0, 0, 0],
[0, 0, 0, 9, 0, 10, 0, 0, 0],
[0, 0, 4, 14, 10, 0, 2, 0, 0],
[0, 0, 0, 0, 0, 2, 0, 1, 6],
[8, 11, 0, 0, 0, 0, 1, 0, 7],
[0, 0, 2, 0, 0, 0, 6, 7, 0]
]; g.dijkstra(0);
#参考自:https://www.geeksforgeeks.org/dijkstras-shortest-path-algorithm-in-python/
### 回答2:
当然可以帮您编写一个简单的Python代码来实现最短路径算法。在Python中,您可以使用图论库networkx和算法库Dijkstra来快速实现最短路径算法。以下是一个使用这两个库计算最短路径的示例代码:
```python
import networkx as nx
# 创建一个有向图
G = nx.DiGraph()
# 添加边(连接节点)及其对应的权重
G.add_edge('A', 'B', weight=5)
G.add_edge('A', 'C', weight=10)
G.add_edge('B', 'C', weight=3)
G.add_edge('B', 'D', weight=7)
G.add_edge('C', 'D', weight=1)
G.add_edge('C', 'E', weight=8)
G.add_edge('D', 'E', weight=4)
# 计算最短路径
shortest_path = nx.shortest_path(G, 'A', 'E', weight='weight')
shortest_distance = nx.shortest_path_length(G, 'A', 'E', weight='weight')
# 输出结果
print("最短路径:", shortest_path)
print("最短距离:", shortest_distance)
```
上述代码创建了一个有向图,并添加了一些有权重的边。然后使用networkx库中的`shortest_path`函数和`shortest_path_length`函数分别计算了从节点'A'到节点'E'的最短路径与最短距离。最后,输出了计算结果。
请注意,上述代码只是一个简单的示例,以帮助您快速上手最短路径算法。在实际应用中,您可能需要根据具体需求来构建图,并使用适当的权重作为输入。此外,网络库networkx还提供了其他常见的最短路径算法,如Bellman-Ford算法和Floyd-Warshall算法,您可以根据需要选择适合您问题的算法。
### 回答3:
当然可以帮你写一个使用Python的最短路径算法的代码。
在Python中,最常用的最短路径算法是迪杰斯特拉算法(Dijkstra's Algorithm)。这个算法用于在加权图中找到两个节点之间的最短路径。
以下是一个使用Python编写的迪杰斯特拉算法的例子:
```python
import sys
def dijkstra(graph, start, end):
# 创建一个字典用于保存每个节点的最短路径
shortest_paths = {start: (None, 0)}
# 创建一个集合用于保存已访问过的节点
visited = set()
# 当还有未访问的节点时
while len(visited) < len(graph):
# 选择当前路径中权重最小的节点
current_node = None
for node in graph:
if node not in visited:
if current_node is None:
current_node = node
elif shortest_paths[node][1] < shortest_paths[current_node][1]:
current_node = node
# 如果无法找到权重最小的节点,则算法结束
if current_node is None:
break
# 标记当前节点为已访问
visited.add(current_node)
# 更新与当前节点相邻节点的最短路径和权重
for neighbor, weight in graph[current_node].items():
if neighbor not in visited:
new_distance = shortest_paths[current_node][1] + weight
if shortest_paths[neighbor][1] is None or new_distance < shortest_paths[neighbor][1]:
shortest_paths[neighbor] = (current_node, new_distance)
# 构建最短路径
path = []
current_node = end
while current_node is not None:
path.insert(0, current_node)
current_node = shortest_paths[current_node][0]
# 返回最短路径和权重
return path, shortest_paths[end][1]
# 举例测试
graph = {
'A': {'B': 1, 'C': 4},
'B': {'A': 1, 'C': 2, 'D': 5},
'C': {'A': 4, 'B': 2, 'D': 1},
'D': {'B': 5, 'C': 1}
}
start_node = 'A'
end_node = 'D'
shortest_path, shortest_distance = dijkstra(graph, start_node, end_node)
print(f"最短路径:{shortest_path}")
print(f"最短路径长度:{shortest_distance}")
```
上述代码通过字典数据结构来表示图,其中节点是键,与之相邻节点及其权重是值。在以上示例中,我们在一个四个节点的图中找到从节点'A'到节点'D'的最短路径。
希望这个代码能够帮助到你!
用python解决使用随机数库生成随机整数功能,范围是[0,1000]: 在内存中,产生三维空间10个随机点 将10个点的坐标分别存入文件: dataX.txt dataYtxt dataZtxt 算出第一个点与其他9个点的距离之和,并将该距离和存入 distanceAll.txt
好的,以下是代码实现:
```python
import random
import math
# 产生三维空间10个随机点
points = []
for i in range(10):
point = (random.randint(0, 1000), random.randint(0, 1000), random.randint(0, 1000))
points.append(point)
# 将10个点的坐标分别存入文件
with open('dataX.txt', 'w') as f:
for point in points:
f.write(str(point[0]) + '\n')
with open('dataY.txt', 'w') as f:
for point in points:
f.write(str(point[1]) + '\n')
with open('dataZ.txt', 'w') as f:
for point in points:
f.write(str(point[2]) + '\n')
# 计算第一个点与其他9个点的距离之和
distance_sum = 0
for i in range(1, 10):
distance = math.sqrt((points[i][0] - points[0][0]) ** 2 + (points[i][1] - points[0][1]) ** 2 + (points[i][2] - points[0][2]) ** 2)
distance_sum += distance
# 将距离和存入文件
with open('distanceAll.txt', 'w') as f:
f.write(str(distance_sum))
```
这样就可以在内存中产生10个随机点,并将这些点的坐标分别存入文件。然后计算第一个点与其他9个点的距离之和,并将该距离和存入文件。
相关推荐
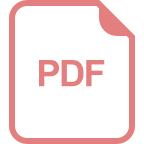










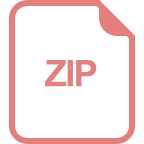