用tensorflow写一个包含多头注意力机制的模型代码
时间: 2024-01-21 22:03:36 浏览: 35
好的,以下是使用TensorFlow实现多头注意力机制的模型代码:
```python
import tensorflow as tf
class MultiHeadAttention(tf.keras.layers.Layer):
def __init__(self, num_heads, d_model):
super(MultiHeadAttention, self).__init__()
self.num_heads = num_heads
self.d_model = d_model
assert d_model % self.num_heads == 0
self.depth = d_model // self.num_heads
self.wq = tf.keras.layers.Dense(d_model)
self.wk = tf.keras.layers.Dense(d_model)
self.wv = tf.keras.layers.Dense(d_model)
self.dense = tf.keras.layers.Dense(d_model)
def split_heads(self, x, batch_size):
x = tf.reshape(x, (batch_size, -1, self.num_heads, self.depth))
return tf.transpose(x, perm=[0, 2, 1, 3])
def call(self, v, k, q, mask):
batch_size = tf.shape(q)[0]
q = self.wq(q)
k = self.wk(k)
v = self.wv(v)
q = self.split_heads(q, batch_size)
k = self.split_heads(k, batch_size)
v = self.split_heads(v, batch_size)
scaled_attention, attention_weights = scaled_dot_product_attention(q, k, v, mask)
scaled_attention = tf.transpose(scaled_attention, perm=[0, 2, 1, 3])
concat_attention = tf.reshape(scaled_attention, (batch_size, -1, self.d_model))
output = self.dense(concat_attention)
return output, attention_weights
def scaled_dot_product_attention(q, k, v, mask):
matmul_qk = tf.matmul(q, k, transpose_b=True)
dk = tf.cast(tf.shape(k)[-1], tf.float32)
scaled_attention_logits = matmul_qk / tf.math.sqrt(dk)
if mask is not None:
scaled_attention_logits += (mask * -1e9)
attention_weights = tf.nn.softmax(scaled_attention_logits, axis=-1)
output = tf.matmul(attention_weights, v)
return output, attention_weights
```
希望可以帮到你,如有疑问欢迎随时提出。
相关推荐
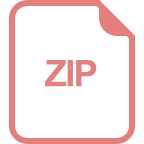














