解释代码 with open("result/ner_predict.utf8", "r", encoding="utf-8") as f: data = f.read() data = [i.split(" ") for i in data.split("\n") if i] print(data) y1 = [i for _, i, _ in data] y2 = [i for _, _, i in data] label = set(list(y1)) acc = accuracy_score(y1, y2) precision = precision_score(y1, y2, average='micro') recall = recall_score(y1, y2, average='micro') f1score = f1_score(y1, y2, average='micro') mcc = matthews_corrcoef(y1, y2) print('accuracy_score is :', acc) print('precision_score is : ', precision) print('recall_score is : ', recall) print('f1_score is : ', f1score) print('matthews_corrcoef is : ', mcc) label = list(set(y1)) matrixs = pd.DataFrame(confusion_matrix(y1, y2, labels=label), index=label, columns=label) del matrixs['O'] matrixs = matrixs[matrixs.index != 'O'] print(matrixs) sns.heatmap(matrixs, cmap="Wistia") # plt.show() plt.savefig("matrixs.png", dpi=300)
时间: 2024-04-28 15:21:28 浏览: 10
这段代码主要是对 NER(命名实体识别)的预测结果进行评估和可视化。下面是代码的具体解释:
1. `with open("result/ner_predict.utf8", "r", encoding="utf-8") as f:`:打开 NER 预测结果文件 ner_predict.utf8,并使用 utf-8 编码方式读取文件内容,使用 with 语句可以确保文件读取完毕后自动关闭文件。
2. `data = f.read()`:读取文件内容并赋值给变量 data。
3. `data = [i.split(" ") for i in data.split("\n") if i]`:根据换行符对 data 进行分割,得到多行文本,然后对每一行文本使用空格进行分割,得到一个二维列表。
4. `y1 = [i for _, i, _ in data]` 和 `y2 = [i for _, _, i in data]`:从二维列表中分别提取出第二列和第三列的值,分别赋值给 y1 和 y2。
5. `label = set(list(y1))`:将 y1 转换成集合类型,得到命名实体类型,赋值给变量 label。
6. `acc = accuracy_score(y1, y2)`、`precision = precision_score(y1, y2, average='micro')`、`recall = recall_score(y1, y2, average='micro')`、`f1score = f1_score(y1, y2, average='micro')` 和 `mcc = matthews_corrcoef(y1, y2)`:使用 sklearn 库中的函数计算各种评估指标,包括准确率、精确率、召回率、f1 分数和 Matthews 相关系数。
7. `print`:输出各种评估指标的值。
8. `label = list(set(y1))`:将 y1 转换成列表类型,得到命名实体类型,赋值给变量 label。
9. `matrixs = pd.DataFrame(confusion_matrix(y1, y2, labels=label), index=label, columns=label)`:使用 pandas 库中的 DataFrame 函数构建混淆矩阵,其中 confusion_matrix 函数可以计算出混淆矩阵,labels 参数用于指定行和列的标签。
10. `del matrixs['O']` 和 `matrixs = matrixs[matrixs.index != 'O']`:将矩阵中的 O 类别删除,并将矩阵中行标签为 O 的行删除,因为 O 类别通常表示无命名实体。
11. `print(matrixs)`:打印出处理后的混淆矩阵。
12. `sns.heatmap(matrixs, cmap="Wistia")`:使用 seaborn 库中的 heatmap 函数将混淆矩阵以热力图的形式进行可视化,cmap 参数指定使用的颜色映射。
13. `plt.savefig("matrixs.png", dpi=300)`:将热力图保存为图片文件。
相关推荐
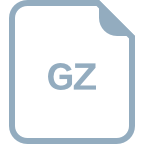















