pytorch_lightning的pl.LightningModule没有forward吗?
时间: 2024-04-23 20:23:36 浏览: 9
在 PyTorch Lightning 中,`pl.LightningModule` 类仍然有 `forward` 方法,它是用来定义模型的向前传递逻辑的。但是,与原生的 PyTorch 中的 `nn.Module` 不同,`pl.LightningModule` 还包含许多其他的方法,例如 `training_step`、`validation_step`、`configure_optimizers` 等等,这些方法使得训练过程更加易于管理和自定义。`training_step` 和 `validation_step` 可以用来定义训练和验证过程的逻辑,`configure_optimizers` 用来定义优化器和学习率调度器。通过使用 PyTorch Lightning,您可以将精力集中在模型设计和研究上,而不是在编写训练循环和调试代码上。
相关问题
import pytorch_lightning as pl
# As an AI language model, I cannot provide a personal experience with PyTorch Lightning, but I can provide information and examples on how to use it.
PyTorch Lightning is a lightweight PyTorch wrapper for high-performance AI research. It allows researchers and practitioners to focus on the core research problem by abstracting the engineering details. PyTorch Lightning provides a high-level interface for building complex deep learning models and training pipelines. It also simplifies the process of scaling models to multiple GPUs or TPUs.
Here is an example of how to use PyTorch Lightning to train a simple neural network for image classification:
```python
import torch
import torch.nn as nn
import torch.optim as optim
from torch.utils.data import DataLoader
from torchvision.datasets import MNIST
from torchvision.transforms import ToTensor
import pytorch_lightning as pl
class Net(nn.Module):
def __init__(self):
super(Net, self).__init__()
self.conv1 = nn.Conv2d(1, 32, 3, 1)
self.conv2 = nn.Conv2d(32, 64, 3, 1)
self.dropout1 = nn.Dropout2d(0.25)
self.dropout2 = nn.Dropout2d(0.5)
self.fc1 = nn.Linear(9216, 128)
self.fc2 = nn.Linear(128, 10)
def forward(self, x):
x = self.conv1(x)
x = nn.ReLU()(x)
x = self.conv2(x)
x = nn.ReLU()(x)
x = nn.MaxPool2d(2)(x)
x = self.dropout1(x)
x = torch.flatten(x, 1)
x = self.fc1(x)
x = nn.ReLU()(x)
x = self.dropout2(x)
x = self.fc2(x)
output = nn.LogSoftmax(dim=1)(x)
return output
class LitMNIST(pl.LightningModule):
def __init__(self):
super().__init__()
self.net = Net()
def forward(self, x):
return self.net(x)
def training_step(self, batch, batch_idx):
x, y = batch
y_hat = self(x)
loss = nn.NLLLoss()(y_hat, y)
self.log('train_loss', loss)
return loss
def configure_optimizers(self):
optimizer = optim.Adam(self.parameters(), lr=1e-3)
return optimizer
train_data = MNIST('.', train=True, download=True, transform=ToTensor())
train_loader = DataLoader(train_data, batch_size=64)
trainer = pl.Trainer(gpus=1, max_epochs=10)
model = LitMNIST()
trainer.fit(model, train_loader)
```
In this example, we define a simple neural network for image classification using PyTorch. We then wrap the model in a PyTorch Lightning module, which provides hooks for training and validation steps. We define a training step that calculates the loss and logs it to the PyTorch Lightning log, and we configure the optimizer to use the Adam optimizer. Finally, we create a PyTorch DataLoader for the MNIST dataset, create a PyTorch Lightning trainer with one GPU, and fit the model to the training data for 10 epochs.
Overall, PyTorch Lightning simplifies the process of training deep learning models while still allowing for flexibility and customization.
pytorch_lightning.utilities.exceptions.MisconfigurationException: No `test_dataloader()` method defined to run `Trainer.test`.
这个错误是由 PyTorch Lightning 框架抛出的,通常出现在测试阶段缺少 `test_dataloader()` 方法的情况下。
要解决这个错误,你需要在你的 PyTorch Lightning 模型中实现一个 `test_dataloader()` 方法,该方法应该返回一个 `DataLoader` 对象,用于加载测试数据。例如:
```python
from torch.utils.data import DataLoader, Dataset
class MyDataset(Dataset):
def __init__(self):
pass
def __len__(self):
return 10
def __getitem__(self, idx):
return idx, idx + 1
class MyModel(pl.LightningModule):
def __init__(self):
super().__init__()
self.linear = torch.nn.Linear(1, 1)
def forward(self, x):
return self.linear(x)
def training_step(self, batch, batch_idx):
x, y = batch
y_hat = self(x)
loss = F.mse_loss(y_hat, y)
self.log('train_loss', loss)
return loss
def configure_optimizers(self):
return torch.optim.Adam(self.parameters(), lr=0.001)
def test_dataloader(self):
return DataLoader(MyDataset(), batch_size=2)
```
在上面的代码中,我们实现了一个简单的 PyTorch Lightning 模型 `MyModel`,并在其中定义了一个 `test_dataloader()` 方法,该方法返回一个 `DataLoader` 对象,用于加载测试数据。在这个例子中,我们使用了一个简单的数据集 `MyDataset`,用于生成一些虚拟数据。
如果你已经实现了 `test_dataloader()` 方法,但仍然遇到了这个错误,你可以检查一下你的 `test_dataloader()` 方法是否正确实现。你可以使用 `print` 语句或调试器来检查你的 `test_dataloader()` 方法是否返回了一个有效的数据加载器对象。
相关推荐












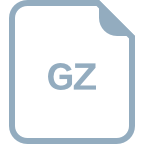