balanced_accuracy_score怎么导入 python
时间: 2024-04-08 10:34:50 浏览: 115
要导入`balanced_accuracy_score`函数,你需要从`sklearn.metrics`模块中导入它。可以按照以下代码示例导入:
```python
from sklearn.metrics import balanced_accuracy_score
```
导入后,你就可以使用`balanced_accuracy_score`函数来计算平衡精度得分了。
如果你在使用中遇到任何问题,请随时提问。
相关问题
['accuracy', 'adjusted_mutual_info_score', 'adjusted_rand_score', 'average_precision', 'balanced_accuracy', 'completeness_score', 'explained_variance', 'f1', 'f1_macro', 'f1_micro', 'f1_samples', 'f1_weighted', 'fowlkes_mallows_score', 'homogeneity_score', 'jaccard', 'jaccard_macro', 'jaccard_micro', 'jaccard_samples', 'jaccard_weighted', 'matthews_corrcoef', 'max_error', 'mutual_info_score', 'neg_brier_score', 'neg_log_loss', 'neg_mean_absolute_error', 'neg_mean_absolute_percentage_error', 'neg_mean_gamma_deviance', 'neg_mean_poisson_deviance', 'neg_mean_squared_error', 'neg_mean_squared_log_error', 'neg_median_absolute_error', 'neg_negative_likelihood_ratio', 'neg_root_mean_squared_error', 'normalized_mutual_info_score', 'positive_likelihood_ratio', 'precision', 'precision_macro', 'precision_micro', 'precision_samples', 'precision_weighted', 'r2', 'rand_score', 'recall', 'recall_macro', 'recall_micro', 'recall_samples', 'recall_weighted', 'roc_auc', 'roc_auc_ovo', 'roc_auc_ovo_weighted', 'roc_auc_ovr', 'roc_auc_ovr_weighted', 'top_k_accuracy', 'v_measure_score']哪些适合xgboost回归
对于XGBoost回归问题,以下指标适合用于评估模型性能:
- explained_variance:解释方差
- neg_mean_absolute_error:负均绝对误差
- neg_mean_squared_error:负均方误差
- neg_mean_squared_log_error:负对数均方误差
- neg_median_absolute_error:负中值绝对误差
- r2:R平方值
这些指标可以通过scikit-learn库中的相关函数来计算。例如,使用neg_mean_absolute_error函数计算负均绝对误差:
```python
from sklearn.metrics import mean_absolute_error
# 假设y_true是真实值,y_pred是模型预测值
mae = mean_absolute_error(y_true, y_pred)
neg_mae = -mae
```
你可以根据需要选择适当的指标进行评估。请注意,这些指标适用于回归问题,不适用于分类问题。
raise ValueError( ValueError: For evaluating multiple scores, use sklearn.model_selection.cross_validate instead. ['accuracy', 'adjusted_mutual_info_score', 'adjusted_rand_score', 'average_precision', 'balanced_accuracy', 'completeness_score', 'explained_variance', 'f1', 'f1_macro', 'f1_micro', 'f1_samples', 'f1_weighted', 'fowlkes_mallows_score', 'homogeneity_score', 'jaccard', 'jaccard_macro', 'jaccard_micro', 'jaccard_samples', 'jaccard_weighted', 'matthews_corrcoef', 'max_error', 'mutual_info_score', 'neg_brier_score', 'neg_log_loss', 'neg_mean_absolute_error', 'neg_mean_absolute_percentage_error', 'neg_mean_gamma_deviance', 'neg_mean_poisson_deviance', 'neg_mean_squared_error', 'neg_mean_squared_log_error', 'neg_median_absolute_error', 'neg_negative_likelihood_ratio', 'neg_root_mean_squared_error', 'normalized_mutual_info_score', 'positive_likelihood_ratio', 'precision', 'precision_macro', 'precision_micro', 'precision_samples', 'precision_weighted', 'r2', 'rand_score', 'recall', 'recall_macro', 'recall_micro', 'recall_samples', 'recall_weighted', 'roc_auc', 'roc_auc_ovo', 'roc_auc_ovo_weighted', 'roc_auc_ovr', 'roc_auc_ovr_weighted', 'top_k_accuracy', 'v_measure_score'] was passed.
这个错误是因为你在调用某个函数时传入了多个评估指标,而该函数不支持同时对多个指标进行评估。建议使用 sklearn.model_selection.cross_validate 函数来对多个指标进行评估。你可以将评估指标作为参数传递给该函数。例如:
```python
from sklearn.model_selection import cross_validate
from sklearn.linear_model import LogisticRegression
from sklearn.datasets import make_classification
X, y = make_classification(random_state=0)
clf = LogisticRegression(random_state=0)
scoring = ['accuracy', 'precision_macro', 'recall_macro']
scores = cross_validate(clf, X, y, scoring=scoring)
```
这样就可以同时对 accuracy、precision_macro 和 recall_macro 三个指标进行评估了。
阅读全文
相关推荐
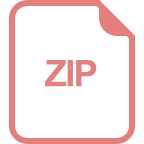
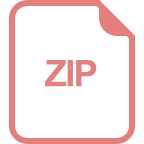
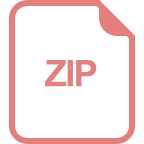
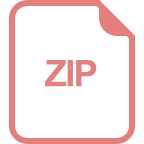
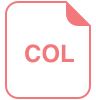
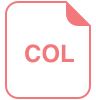
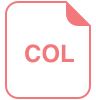
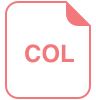
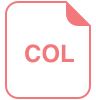
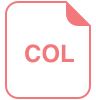
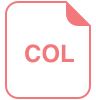
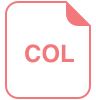
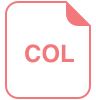
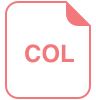
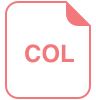
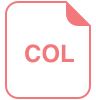
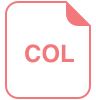
