output = [F.interpolate(output[0], (h,w)), *output[1:]]
时间: 2023-10-30 22:04:53 浏览: 140
这段代码是对输出进行处理的逻辑。假设`output`是一个列表,其中第一个元素是预测结果的张量,而其他元素是其他相关信息。
代码中使用了`F.interpolate()`函数对预测结果的张量进行插值操作。插值操作的目的是将预测结果的大小调整为`(h, w)`的尺寸。
具体来说,`F.interpolate(output[0], (h,w))`将预测结果的张量进行插值,使其尺寸变为`(h, w)`。然后,使用`*output[1:]`将原始的预测结果列表中除第一个元素外的其他元素保持不变。
最终,将插值后的预测结果和其他元素组成一个新的列表,并将其赋值给`output`变量。这样,输出列表中的第一个元素就是插值后的预测结果,而其他元素保持不变。
相关问题
if name in self.return_layers: out_name = self.return_layers[name] if name == 'stage4' and self.hrnet_flag: # In HRNetV2, we upsample and concat all outputs streams together output_h, output_w = x[0].size(2), x[0].size(3) # Upsample to size of highest resolution stream x1 = F.interpolate(x[1], size=(output_h, output_w), mode='bilinear', align_corners=False) x2 = F.interpolate(x[2], size=(output_h, output_w), mode='bilinear', align_corners=False) x3 = F.interpolate(x[3], size=(output_h, output_w), mode='bilinear', align_corners=False) x = torch.cat([x[0], x1, x2, x3], dim=1) out[out_name] = x else: out[out_name] = x return out
这段代码包含在一个 PyTorch 模型的 forward 方法中,用于处理模型的输出。该模型是一个分割模型,返回多个分辨率的特征图。在这段代码中,使用了一个字典 out 来存储输出,其中键为特征图的名称,值为特征图。该模型中包含了多个子模块,其中一些子模块的输出需要单独返回。这个函数首先使用了 module.named_modules() 方法来遍历模块中的所有子模块,然后根据设定的 return_layers 字典来判断是否需要返回该子模块的输出。如果需要返回该子模块的输出,则将其存储到 out 字典中。在 HRNetV2 这个模型中,如果需要返回 stage4 子模块的输出,需要将其它三个子模块的输出进行上采样并拼接在一起,最后将拼接后的特征图存储到 out 字典中。最后,函数返回 out 字典。
for output, weight in zip(network_output, weights): loss += weight * one_scale(output, target_flow, sparse) return loss def realEPE(output, target, sparse=False): b, _, h, w = target.size() upsampled_output = F.interpolate(output, (h,w), mode='bilinear', align_corners=False) return EPE(upsampled_output, target, sparse, mean=True)
这段代码是用来计算神经网络的损失函数和真实误差的函数。其中,函数one_scale是一个用于计算单个像素误差的函数,网络输出为network_output,权重为weights,目标流为target_flow,sparse表示是否使用稀疏方法计算误差。函数realEPE用于计算网络输出与目标之间的真实误差,其中使用了双线性插值将网络输出上采样到与目标相同的大小,然后调用EPE函数计算误差。最终结果为平均误差。
阅读全文
相关推荐
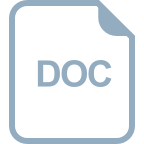
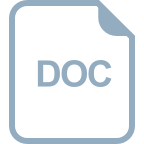
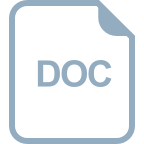













