n = int(input())max_num = (n // 17) * 17print(max_num)
时间: 2023-11-20 09:06:09 浏览: 30
This code takes an integer input from the user, calculates the maximum multiple of 17 that is less than or equal to the input number, and prints it out.
For example, if the user inputs 100, the code calculates that the largest multiple of 17 that is less than or equal to 100 is 85 (since 5 * 17 = 85), and prints out 85.
If the input number is already a multiple of 17, then the code will simply print out that number as the maximum.
Note that the code assumes that the input number is positive. If the input number is negative, the code will still calculate the maximum multiple of 17 that is less than or equal to the absolute value of the input number, but the result will be negative.
相关问题
ls1 = list(map(int, input().split())) set1 = set(ls1) max_num = -1 for i in set1: if ls1.count(i) == i: if i > max_num: max_num = i print(max_num)
这段代码的功能是从标准输入中读入一组整数,找到其中满足该整数在数组中出现的次数等于该整数本身的最大整数,并输出该整数。
具体实现过程如下:
1. 从标准输入中读入一组整数,使用 `input()` 函数获取输入的字符串,使用 `split()` 函数将字符串按空格分割为多个子字符串,使用 `map()` 函数将每个子字符串转换为整数,并使用 `list()` 函数将转换后的整数组成列表。
2. 使用 `set()` 函数将列表中的元素去重,得到一个集合 `set1`,其中每个元素都是不重复的。
3. 初始化变量 `max_num` 为 -1,用于记录满足条件的最大整数。
4. 使用 for 循环遍历集合 `set1` 中的每个元素 `i`。
5. 使用 `ls1.count(i)` 函数统计整数 `i` 在列表 `ls1` 中出现的次数,如果该次数等于整数 `i` 本身,则说明该整数满足要求。
6. 如果整数 `i` 满足要求,并且比当前记录的最大整数 `max_num` 大,则将 `max_num` 更新为 `i`。
7. 循环结束后,变量 `max_num` 就是满足条件的最大整数。使用 `print(max_num)` 输出该整数。
需要注意的是,这段代码中没有对输入的整数个数和范围做出任何限制,如果输入的整数范围较大,程序的效率可能会比较低下。
num1=int(input()) num2=int(input()) a=max(num1,num2) b=min(num1,num2) r=a%b while r!=0: a=b b=r r=b%a sum=(num1*num2)//b print(sum)
你给出的代码实现了辗转相除法求最大公约数,并最终计算出了最小公倍数。代码中的变量名和实现方式与我之前给出的略有不同,但基本思路相同,都是通过循环实现辗转相除法,得出最大公约数,再通过 `num1*num2//最大公约数` 计算最小公倍数。
不过,你的代码中有一点需要注意的是,当 `r=b%a` 时,应该将 `r` 赋值给 `b`,而不是 `a`。应该修改为:
```python
num1 = int(input())
num2 = int(input())
a = max(num1, num2)
b = min(num1, num2)
r = a % b
while r != 0:
a = b
b = r
r = a % b
sum = (num1 * num2) // b
print(sum)
```
另外,建议加上变量名的注释,以便代码更易读懂。
相关推荐
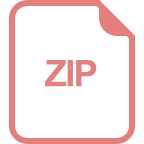
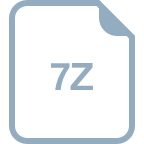
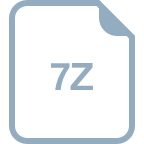













