def weights(self): if not self._warmed_up(): return np.ones([self.diffusion.num_timesteps], dtype=np.float64) weights = np.sqrt(np.mean(self._loss_history ** 2, axis=-1)) weights /= np.sum(weights) weights *= 1 - self.uniform_prob weights += self.uniform_prob / len(weights) return weights具体解释
时间: 2024-04-25 13:26:27 浏览: 105
这段代码是一个函数,名为`weights()`,可能是在某个类中定义的。下面是代码的解释:
1. `if not self._warmed_up():` 这行代码判断是否已经完成了模型的热身,如果没有,则返回一个所有权重都为1的数组。
2. `weights = np.sqrt(np.mean(self._loss_history ** 2, axis=-1))` 这行代码计算了一个权重数组,这个数组是用历史损失的平均值的平方根来计算的。
3. `weights /= np.sum(weights)` 这行代码将权重数组归一化,使其总和为1。
4. `weights *= 1 - self.uniform_prob` 这行代码对归一化后的权重数组进行了调整,使其总和为1-self.uniform_prob。
5. `weights += self.uniform_prob / len(weights)` 这行代码将uniform_prob除以权重数组的长度,并将结果添加到权重数组中,以确保总和为1。
6. 最后,函数返回了计算得到的权重数组。
总体来说,这个函数的作用是计算一个权重数组,用于在模型训练时平衡不同时间步的重要性。它将历史损失的平均值作为权重,并将其归一化,以确保总和为1。此外,它还添加了一个uniform_prob参数,用于控制所有时间步的平均权重。
相关问题
这段代码实现什么功能# 指数加权平均 class ExponentialMovingAverage(Callback): def __init__(self, decay=0.9): super().__init__() self.decay = decay self.weights = None def on_epoch_begin(self, epoch, logs=None): self.weights = None def on_batch_end(self, batch, logs=None): # 计算指数加权平均 if self.weights is None: self.weights = [np.ones_like(p) for p in self.model.get_weights()] for i, p in enumerate(self.model.get_weights()): self.weights[i] = self.decay * self.weights[i] + (1 - self.decay) * p smoothed_p = self.weights[i] / (1 - self.decay ** (batch + 1)) K.set_value(p, smoothed_p)
这段代码实现的是指数加权平均的功能,其中ExponentialMovingAverage类是一个回调函数,用于在训练神经网络时进行参数平滑处理。在每个batch结束时,该回调函数将计算指数加权平均,平滑模型权重并更新模型参数。其中decay参数是平滑系数,用于控制指数加权平均的权重分配。在每个epoch开始时,将self.weights设置为None,以确保每个epoch的平滑处理是独立的。
class Client(object): def __init__(self, conf, public_key, weights, data_x, data_y): self.conf = conf self.public_key = public_key self.local_model = models.LR_Model(public_key=self.public_key, w=weights, encrypted=True) #print(type(self.local_model.encrypt_weights)) self.data_x = data_x self.data_y = data_y #print(self.data_x.shape, self.data_y.shape) def local_train(self, weights): original_w = weights self.local_model.set_encrypt_weights(weights) neg_one = self.public_key.encrypt(-1) for e in range(self.conf["local_epochs"]): print("start epoch ", e) #if e > 0 and e%2 == 0: # print("re encrypt") # self.local_model.encrypt_weights = Server.re_encrypt(self.local_model.encrypt_weights) idx = np.arange(self.data_x.shape[0]) batch_idx = np.random.choice(idx, self.conf['batch_size'], replace=False) #print(batch_idx) x = self.data_x[batch_idx] x = np.concatenate((x, np.ones((x.shape[0], 1))), axis=1) y = self.data_y[batch_idx].reshape((-1, 1)) #print((0.25 * x.dot(self.local_model.encrypt_weights) + 0.5 * y.transpose() * neg_one).shape) #print(x.transpose().shape) #assert(False) batch_encrypted_grad = x.transpose() * (0.25 * x.dot(self.local_model.encrypt_weights) + 0.5 * y.transpose() * neg_one) encrypted_grad = batch_encrypted_grad.sum(axis=1) / y.shape[0] for j in range(len(self.local_model.encrypt_weights)): self.local_model.encrypt_weights[j] -= self.conf["lr"] * encrypted_grad[j] weight_accumulators = [] #print(models.decrypt_vector(Server.private_key, weights)) for j in range(len(self.local_model.encrypt_weights)): weight_accumulators.append(self.local_model.encrypt_weights[j] - original_w[j]) return weight_accumulators
这段代码看起来是一个客户端的类实现,其中包含了初始化函数和本地训练函数。初始化函数接受一些参数,包括全局配置conf、公钥public_key、权重weights、数据x和数据y。在初始化函数中,使用公钥public_key和权重weights创建一个加密的逻辑回归模型local_model,并将数据x和y保存在类实例中。
本地训练函数local_train接受一个权重参数weights,并将其设置为local_model的加密权重。在函数中,使用随机梯度下降算法对模型进行训练,其中每次迭代从数据集中随机选择一个batch_size大小的样本进行训练。在计算梯度时,使用加密权重对样本进行预测,并使用公钥对-1进行加密,然后计算损失函数的梯度并进行加密。最后,使用学习率lr对加密权重进行更新,并将更新的权重返回给调用者。
需要注意的是,本地训练函数中使用了加密的权重和加密的梯度进行计算,以确保数据隐私不会被泄露。
阅读全文
相关推荐
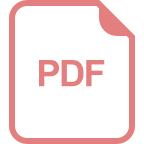
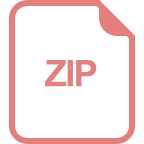
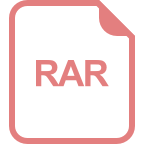













