X_train = tf.convert_to_tensor(X_train, dtype=tf.float32) 修改成long型
时间: 2024-02-02 08:04:25 浏览: 140
如果需要将`X_train`转换为`long`类型,可以使用以下代码:
```
X_train = tf.convert_to_tensor(X_train, dtype=tf.int64)
```
这将把`X_train`转换为`int64`类型的张量。注意,如果`X_train`中的值不是整数,则会引发错误。
相关问题
test_x_H = tf.convert_to_tensor(test_x_H) test_x_H = tf.convert_to_tensor(test_x_H)
这段代码中,第二行的代码似乎是多余的,因为它在对 `test_x_H` 进行第二次转换时没有任何作用。
如果你已经将 `test_x_H` 转换为 TensorFlow 的 `Tensor` 类型,那么它已经可以被 TensorFlow 的函数使用了。如果你需要使用 `test_x_H`,只需要直接将它传递给 TensorFlow 的函数即可,不需要进行第二次转换。
因此,你可以只保留第一行代码,将 `test_x_H` 转换为 `Tensor` 类型:
```
test_x_H = tf.convert_to_tensor(test_x_H)
```
希望这个解决方案能够帮助你。如果你还有其他问题或疑问,请随时提出。
x_train = torch.as_tensor(x_train, dtype=torch.float32)
`x_train = torch.as_tensor(x_train, dtype=torch.float32)` 这行代码的作用是将 `x_train` 转换为一个 PyTorch 张量,并且指定数据类型为 `torch.float32`。`torch.as_tensor()` 函数将给定的数组或列表转换为张量,并且保留其原始数据类型。在这个例子中,`x_train` 应该是一个包含训练数据的数组或列表,通过调用 `torch.as_tensor()` 将其转换为张量,并且指定数据类型为 `torch.float32`,以便在后续的计算中使用。
阅读全文
相关推荐
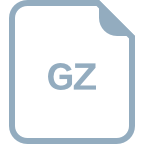
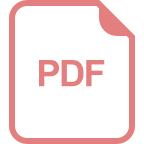
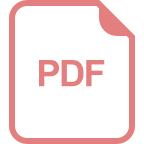













