transform注意力机制代码
时间: 2023-10-22 16:09:18 浏览: 125
transformer的注意力机制代码如下:
```python
import torch
import torch.nn.functional as F
class MultiHeadAttention(torch.nn.Module):
def __init__(self, d_model, num_heads):
super().__init__()
self.d_model = d_model
self.num_heads = num_heads
self.head_dim = d_model // num_heads
self.query = torch.nn.Linear(d_model, d_model)
self.key = torch.nn.Linear(d_model, d_model)
self.value = torch.nn.Linear(d_model, d_model)
self.fc = torch.nn.Linear(d_model, d_model)
def forward(self, query, key, value, mask=None):
batch_size = query.shape[0]
# Linear layers
Q = self.query(query)
K = self.key(key)
V = self.value(value)
# Split into heads
Q = Q.view(batch_size, -1, self.num_heads, self.head_dim).transpose(1, 2)
K = K.view(batch_size, -1, self.num_heads, self.head_dim).transpose(1, 2)
V = V.view(batch_size, -1, self.num_heads, self.head_dim).transpose(1, 2)
# Scaled Dot-Product Attention
scores = torch.matmul(Q, K.transpose(-1, -2)) / torch.sqrt(torch.tensor(self.head_dim).float())
if mask is not None:
scores += mask.unsqueeze(1)
attention_weights = F.softmax(scores, dim=-1)
output = torch.matmul(attention_weights, V)
# Concatenate heads and pass through final linear layer
output = output.transpose(1, 2).contiguous().view(batch_size, -1, self.d_model)
output = self.fc(output)
return output, attention_weights
```
其中,`query`、`key`、`value`分别表示查询、键、值,`mask`表示掩码。该函数返回注意力机制的输出和注意力权重。
阅读全文
相关推荐
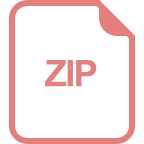
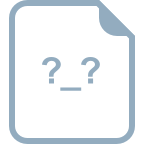
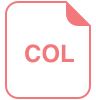
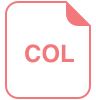
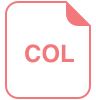
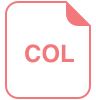
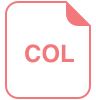
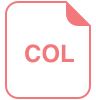
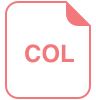








