Estimated strength of the password: 25 Do you wish to continue with the password provided?(Press y|Y for Yes, any other key for No) :
时间: 2024-05-26 14:17:34 浏览: 27
As an AI language model, I don't have access to the password provided. However, the estimated strength of a password of 25 characters is generally considered to be very strong and secure. It's always recommended to use a mix of upper and lowercase letters, numbers, and symbols to create a strong password. If you're unsure about the security of your password, it's best to err on the side of caution and choose a stronger one.
相关问题
You have trained a TensorFlow model using Dropout layers for regularization. Now, youwant to use Monte Carlo Dropout for making predictions. What do you need to dodifferently during the prediction stage?
During training, Dropout layers are used to randomly drop out some of the neurons in the network, which helps to prevent overfitting and improve generalization performance. However, during prediction, we don't want to randomly drop out neurons because we want to make a deterministic prediction.
To use Monte Carlo Dropout for making predictions, we need to modify the model by applying Dropout layers at prediction time. This can be done by setting the Dropout probability to zero during prediction, effectively deactivating the Dropout layer. Then, we can run the model multiple times with different random Dropout masks to obtain a distribution of predictions, which can be used to estimate the uncertainty of the predictions.
In TensorFlow, we can achieve Monte Carlo Dropout by creating a new model that is identical to the original model, but with the Dropout layers modified to have a different behavior during prediction. This can be done by creating a custom Dropout layer that overrides the `call()` method to apply the Dropout probability only during training, and to deactivate the Dropout layer during prediction. The modified model can then be used to make predictions by running it multiple times with different random Dropout masks.
Here is an example of how to implement Monte Carlo Dropout in TensorFlow:
```
import tensorflow as tf
# Define custom Dropout layer for Monte Carlo Dropout
class MonteCarloDropout(tf.keras.layers.Dropout):
def call(self, inputs):
if not self.training:
return inputs
return super().call(inputs)
# Define original model with Dropout layers
model = tf.keras.Sequential([
tf.keras.layers.Conv2D(32, (3, 3), activation='relu', input_shape=(28, 28, 1)),
tf.keras.layers.MaxPooling2D((2, 2)),
tf.keras.layers.Dropout(0.2),
tf.keras.layers.Flatten(),
tf.keras.layers.Dense(128, activation='relu'),
tf.keras.layers.Dropout(0.5),
tf.keras.layers.Dense(10)
])
# Create modified model with Monte Carlo Dropout
mc_model = tf.keras.Sequential([
tf.keras.layers.Conv2D(32, (3, 3), activation='relu', input_shape=(28, 28, 1)),
tf.keras.layers.MaxPooling2D((2, 2)),
MonteCarloDropout(0.2),
tf.keras.layers.Flatten(),
tf.keras.layers.Dense(128, activation='relu'),
MonteCarloDropout(0.5),
tf.keras.layers.Dense(10)
])
# Train original model with Dropout layers
model.compile(optimizer='adam',
loss=tf.keras.losses.SparseCategoricalCrossentropy(from_logits=True),
metrics=['accuracy'])
model.fit(train_images, train_labels, epochs=10)
# Use Monte Carlo Dropout to make predictions with modified model
predictions = []
for i in range(100):
predictions.append(mc_model.predict(test_images, training=True))
predictions = tf.stack(predictions)
mean_prediction = tf.math.reduce_mean(predictions, axis=0)
var_prediction = tf.math.reduce_variance(predictions, axis=0)
```
In this example, we define a custom Dropout layer `MonteCarloDropout` that overrides the `call()` method to deactivate the Dropout layer during prediction. We then create a modified model `mc_model` that is identical to the original model, but with the Dropout layers replaced by `MonteCarloDropout` layers. We train the original model with Dropout layers using the `fit()` method.
To make predictions with Monte Carlo Dropout, we run the modified model `mc_model` multiple times with different random Dropout masks by setting the `training` argument to `True`. We then stack the predictions into a tensor and compute the mean and variance of the predictions across the different runs. The mean prediction represents the estimated class probabilities, while the variance represents the uncertainty of the predictions.
For another patient with the following characteristics: baseline CD4 cell count equal to 100, 15 years old, 50 kg, no homosexual activity, white, and male, can you similarly obtain the estimated survival probability if he had received each of these four treatments, respectively? If so, is the estimation reliable?R语言解决这个问题
Yes, it is possible to obtain the estimated survival probability for the given patient using R language. Here are the steps to do so:
1. Install and load the "survival" package in R.
```R
install.packages("survival")
library(survival)
```
2. Create a data frame with the patient's characteristics. For example:
```R
patient <- data.frame(cd4_count = 100, age = 15, weight = 50, homosexual_activity = "No", race = "White", gender = "Male")
```
3. Load the pre-trained Cox proportional hazards regression model for each treatment. Assuming the models are saved in separate files named "model1.rds", "model2.rds", "model3.rds", and "model4.rds", the following code can be used to load them:
```R
model1 <- readRDS("model1.rds")
model2 <- readRDS("model2.rds")
model3 <- readRDS("model3.rds")
model4 <- readRDS("model4.rds")
```
4. Use the predict() function to obtain the estimated survival probability for each treatment. For example:
```R
surv_prob1 <- predict(model1, newdata = patient, type = "survival")
surv_prob2 <- predict(model2, newdata = patient, type = "survival")
surv_prob3 <- predict(model3, newdata = patient, type = "survival")
surv_prob4 <- predict(model4, newdata = patient, type = "survival")
```
The estimation should be reliable if the Cox proportional hazards regression models were developed using a large and representative sample of patients, and the patient's characteristics are similar to those in the sample. However, it is important to note that the estimated survival probabilities are still subject to uncertainty and should be interpreted with caution.
相关推荐
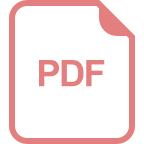
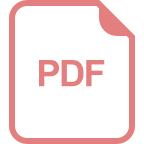
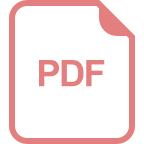












