首页 int page_count = counts % PageBean.PAGE_IETM == 0 ? counts / PageBean.PAGE_IETM : counts / PageBean.PAGE_IETM + 1;
int page_count = counts % PageBean.PAGE_IETM == 0 ? counts / PageBean.PAGE_IETM : counts / PageBean.PAGE_IETM + 1;
时间: 2024-05-23 22:12:35 浏览: 92
这段代码的作用是计算分页的总页数。其中counts表示总记录数,PageBean.PAGE_IETM表示每页显示的记录数。具体实现是:如果总记录数能够整除每页显示的记录数,则总页数等于总记录数除以每页显示的记录数;否则总页数等于总记录数除以每页显示的记录数再加1。最后将计算结果赋值给page_count。
阅读全文
CSDN会员
开通CSDN年卡参与万元壕礼抽奖
最新推荐
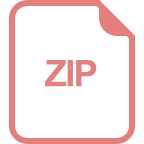
pandas-1.3.5-cp37-cp37m-macosx_10_9_x86_64.zip
pandas whl安装包,对应各个python版本和系统(具体看资源名字),找准自己对应的下载即可!
下载后解压出来是已.whl为后缀的安装包,进入终端,直接pip install pandas-xxx.whl即可,非常方便。
再也不用担心pip联网下载网络超时,各种安装不成功的问题。
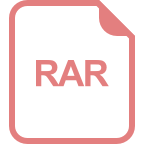
Aspose资源包:转PDF无水印学习工具
资源摘要信息:"Aspose.Cells和Aspose.Words是两个非常强大的库,它们属于Aspose.Total产品家族的一部分,主要面向.NET和Java开发者。Aspose.Cells库允许用户轻松地操作Excel电子表格,包括创建、修改、渲染以及转换为不同的文件格式。该库支持从Excel 97-2003的.xls格式到最新***016的.xlsx格式,还可以将Excel文件转换为PDF、HTML、MHTML、TXT、CSV、ODS和多种图像格式。Aspose.Words则是一个用于处理Word文档的类库,能够创建、修改、渲染以及转换Word文档到不同的格式。它支持从较旧的.doc格式到最新.docx格式的转换,还包括将Word文档转换为PDF、HTML、XAML、TIFF等格式。
Aspose.Cells和Aspose.Words都有一个重要的特性,那就是它们提供的输出资源包中没有水印。这意味着,当开发者使用这些资源包进行文档的处理和转换时,最终生成的文档不会有任何水印,这为需要清洁输出文件的用户提供了极大的便利。这一点尤其重要,在处理敏感文档或者需要高质量输出的企业环境中,无水印的输出可以帮助保持品牌形象和文档内容的纯净性。
此外,这些资源包通常会标明仅供学习使用,切勿用作商业用途。这是为了避免违反Aspose的使用协议,因为Aspose的产品虽然是商业性的,但也提供了免费的试用版本,其中可能包含了特定的限制,如在最终输出的文档中添加水印等。因此,开发者在使用这些资源包时应确保遵守相关条款和条件,以免产生法律责任问题。
在实际开发中,开发者可以通过NuGet包管理器安装Aspose.Cells和Aspose.Words,也可以通过Maven在Java项目中进行安装。安装后,开发者可以利用这些库提供的API,根据自己的需求编写代码来实现各种文档处理功能。
对于Aspose.Cells,开发者可以使用它来完成诸如创建电子表格、计算公式、处理图表、设置样式、插入图片、合并单元格以及保护工作表等操作。它也支持读取和写入XML文件,这为处理Excel文件提供了更大的灵活性和兼容性。
而对于Aspose.Words,开发者可以利用它来执行文档格式转换、读写文档元数据、处理文档中的文本、格式化文本样式、操作节、页眉、页脚、页码、表格以及嵌入字体等操作。Aspose.Words还能够灵活地处理文档中的目录和书签,这让它在生成复杂文档结构时显得特别有用。
在使用这些库时,一个常见的场景是在企业应用中,需要将报告或者数据导出为PDF格式,以便于打印或者分发。这时,使用Aspose.Cells和Aspose.Words就可以实现从Excel或Word格式到PDF格式的转换,并且确保输出的文件中不包含水印,这提高了文档的专业性和可信度。
需要注意的是,虽然Aspose的产品提供了很多便利的功能,但它们通常是付费的。用户需要根据自己的需求购买相应的许可证。对于个人用户和开源项目,Aspose有时会提供免费的许可证。而对于商业用途,用户则需要购买商业许可证才能合法使用这些库的所有功能。"

管理建模和仿真的文件
管理Boualem Benatallah引用此版本:布阿利姆·贝纳塔拉。管理建模和仿真。约瑟夫-傅立叶大学-格勒诺布尔第一大学,1996年。法语。NNT:电话:00345357HAL ID:电话:00345357https://theses.hal.science/tel-003453572008年12月9日提交HAL是一个多学科的开放存取档案馆,用于存放和传播科学研究论文,无论它们是否被公开。论文可以来自法国或国外的教学和研究机构,也可以来自公共或私人研究中心。L’archive ouverte pluridisciplinaire
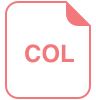
【R语言高性能计算秘诀】:代码优化,提升分析效率的专家级方法
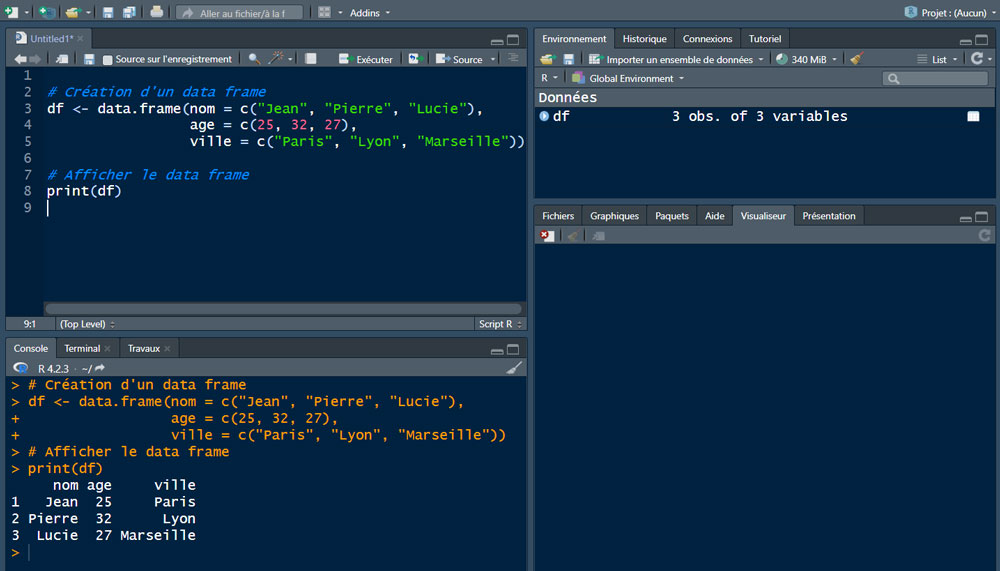
# 1. R语言简介与计算性能概述
R语言作为一种统计编程语言,因其强大的数据处理能力、丰富的统计分析功能以及灵活的图形表示法而受到广泛欢迎。它的设计初衷是为统计分析提供一套完整的工具集,同时其开源的特性让全球的程序员和数据科学家贡献了大量实用的扩展包。由于R语言的向量化操作以及对数据框(data frames)的高效处理,使其在处理大规模数据集时表现出色。
计算性能方面,R语言在单线程环境中表现良好,但与其他语言相比,它的性能在多

在构建视频会议系统时,如何通过H.323协议实现音视频流的高效传输,并确保通信的稳定性?
要通过H.323协议实现音视频流的高效传输并确保通信稳定,首先需要深入了解H.323协议的系统结构及其组成部分。H.323协议包括音视频编码标准、信令控制协议H.225和会话控制协议H.245,以及数据传输协议RTP等。其中,H.245协议负责控制通道的建立和管理,而RTP用于音视频数据的传输。
参考资源链接:[H.323协议详解:从系统结构到通信流程](https://wenku.csdn.net/doc/2jtq7zt3i3?spm=1055.2569.3001.10343)
在构建视频会议系统时,需要合理配置网守(Gatekeeper)来提供地址解析和准入控制,保证通信安全和地址管理
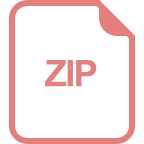
Go语言控制台输入输出操作教程
资源摘要信息:"在Go语言(又称Golang)中,控制台的输入输出是进行基础交互的重要组成部分。Go语言提供了一组丰富的库函数,特别是`fmt`包,来处理控制台的输入输出操作。`fmt`包中的函数能够实现格式化的输入和输出,使得程序员可以轻松地在控制台显示文本信息或者读取用户的输入。"
1. fmt包的使用
Go语言标准库中的`fmt`包提供了许多打印和解析数据的函数。这些函数可以让我们在控制台上输出信息,或者从控制台读取用户的输入。
- 输出信息到控制台
- Print、Println和Printf是基本的输出函数。Print和Println函数可以输出任意类型的数据,而Printf可以进行格式化输出。
- Sprintf函数可以将格式化的字符串保存到变量中,而不是直接输出。
- Fprint系列函数可以将输出写入到`io.Writer`接口类型的变量中,例如文件。
- 从控制台读取信息
- Scan、Scanln和Scanf函数可以读取用户输入的数据。
- Sscan、Sscanln和Sscanf函数则可以从字符串中读取数据。
- Fscan系列函数与上面相对应,但它们是将输入读取到实现了`io.Reader`接口的变量中。
2. 输入输出的格式化
Go语言的格式化输入输出功能非常强大,它提供了类似于C语言的`printf`和`scanf`的格式化字符串。
- Print函数使用格式化占位符
- `%v`表示使用默认格式输出值。
- `%+v`会包含结构体的字段名。
- `%#v`会输出Go语法表示的值。
- `%T`会输出值的数据类型。
- `%t`用于布尔类型。
- `%d`用于十进制整数。
- `%b`用于二进制整数。
- `%c`用于字符(rune)。
- `%x`用于十六进制整数。
- `%f`用于浮点数。
- `%s`用于字符串。
- `%q`用于带双引号的字符串。
- `%%`用于百分号本身。
3. 示例代码分析
在文件main.go中,可能会包含如下代码段,用于演示如何在Go语言中使用fmt包进行基本的输入输出操作。
```go
package main
import "fmt"
func main() {
var name string
fmt.Print("请输入您的名字: ")
fmt.Scanln(&name) // 读取一行输入并存储到name变量中
fmt.Printf("你好, %s!\n", name) // 使用格式化字符串输出信息
}
```
以上代码首先通过`fmt.Print`函数提示用户输入名字,并等待用户从控制台输入信息。然后`fmt.Scanln`函数读取用户输入的一行信息(包括空格),并将其存储在变量`name`中。最后,`fmt.Printf`函数使用格式化字符串输出用户的名字。
4. 代码注释和文档编写
在README.txt文件中,开发者可能会提供关于如何使用main.go代码的说明,这可能包括代码的功能描述、运行方法、依赖关系以及如何处理常见的输入输出场景。这有助于其他开发者理解代码的用途和操作方式。
总之,Go语言为控制台输入输出提供了强大的标准库支持,使得开发者能够方便地处理各种输入输出需求。通过灵活运用fmt包中的各种函数,可以轻松实现程序与用户的交互功能。

"互动学习:行动中的多样性与论文攻读经历"
多样性她- 事实上SCI NCES你的时间表ECOLEDO C Tora SC和NCESPOUR l’Ingén学习互动,互动学习以行动为中心的强化学习学会互动,互动学习,以行动为中心的强化学习计算机科学博士论文于2021年9月28日在Villeneuve d'Asq公开支持马修·瑟林评审团主席法布里斯·勒菲弗尔阿维尼翁大学教授论文指导奥利维尔·皮耶昆谷歌研究教授:智囊团论文联合主任菲利普·普雷教授,大学。里尔/CRISTAL/因里亚报告员奥利维耶·西格德索邦大学报告员卢多维奇·德诺耶教授,Facebook /索邦大学审查员越南圣迈IMT Atlantic高级讲师邀请弗洛里安·斯特鲁布博士,Deepmind对于那些及时看到自己错误的人...3谢谢你首先,我要感谢我的两位博士生导师Olivier和Philippe。奥利维尔,"站在巨人的肩膀上"这句话对你来说完全有意义了。从科学上讲,你知道在这篇论文的(许多)错误中,你是我可以依
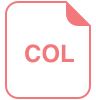
【R语言机器学习新手起步】:caret包带你进入预测建模的世界
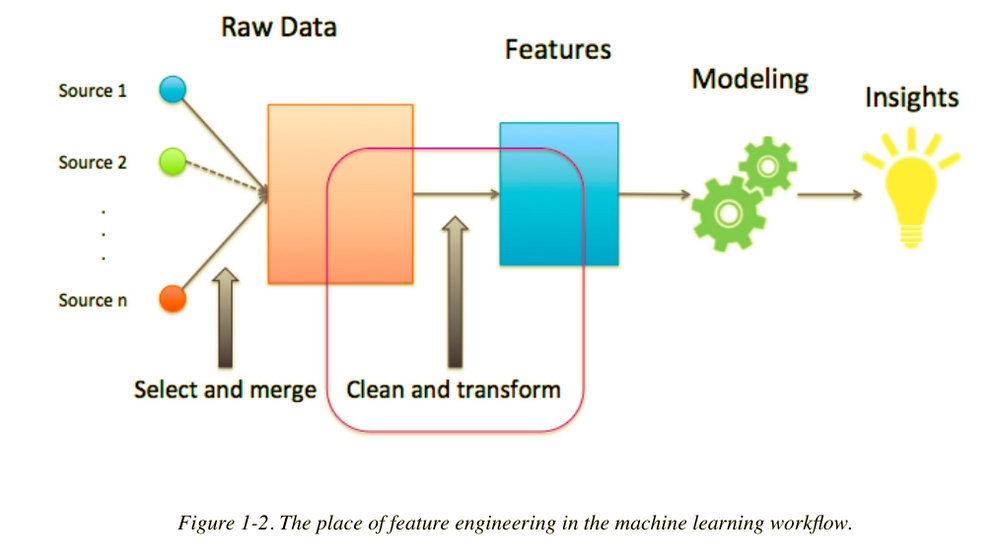
# 1. R语言机器学习概述
在当今大数据驱动的时代,机器学习已经成为分析和处理复杂数据的强大工具。R语言作为一种广泛使用的统计编程语言,它在数据科学领域尤其是在机器学习应用中占据了不可忽视的地位。R语言提供了一系列丰富的库和工具,使得研究人员和数据分析师能够轻松构建和测试各种机器学

在选择PL2303和CP2102/CP2103 USB转串口芯片时,应如何考虑和比较它们的数据格式和波特率支持能力?
为了确保选择正确的USB转串口芯片,深入理解PL2303和CP2102/CP2103的数据格式和波特率支持能力至关重要。建议查看《USB2TTL芯片对比:PL2303与CP2102/CP2103详解》以获得更深入的理解。
参考资源链接:[USB2TTL芯片对比:PL2303与CP2102/CP2103详解](https://wenku.csdn.net/doc/5ei92h5x7x?spm=1055.2569.3001.10343)
首先,PL2303和CP2102/CP2103都支持多种数据格式,包括数据位、停止位和奇偶校验位的设置。PL2303芯片支持5位到8位数据位,1位或2位停止位
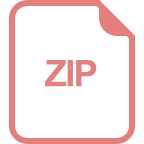
红外遥控报警器原理及应用详解下载
资源摘要信息:"红外遥控报警器"
红外遥控报警器是一种基于红外线技术的安防设备,主要用于监控特定区域的安全,当有人或物进入检测范围时,能够立即触发报警系统。该设备主要由红外线发射器和接收器两大部分构成。发射器不断发送红外线,如果这些红外线被遮挡或中断,接收器会检测到这一变化,并启动报警机制。红外遥控报警器广泛应用于家庭、办公室、仓库等场所,可以有效提高这些区域的安全防范能力。
从技术角度分析,红外遥控报警器的工作原理主要依赖于红外线的直线传播特性。红外线发射器连续发送红外线信号,这些信号构成了一道无形的"红外线帘",覆盖了报警器的监控区域。当有人或物体通过这道红外线帘时,红外线的正常传播路径会被中断,接收器检测到这种中断后,就会输出信号给到报警电路,从而触发报警。
红外遥控报警器的安装和使用相对简便,用户可以根据使用环境和需求进行设置。一般情况下,该设备具有较低的误报率,能够可靠地进行监控。但是,它也存在一些限制。例如,小型动物的移动可能引起误报,强光或低光环境下可能会降低设备的检测能力。因此,用户需要根据实际情况对红外遥控报警器进行适当的调整,以避免误报和漏报。
红外遥控报警器通常还配备有附加功能,如电话语音报警系统。这意味着,一旦报警器被触发,它可以自动拨打预设的电话号码,通过电话语音系统通知房主或者保安中心,提高报警的及时性和有效性。
关于提供的文件资源,包含了红外遥控报警器的相关设计资料和软件代码。资源文件列表包括"红外遥控报警器(原理图+PCB图+程序+说明文档)",这些内容对于设计、开发和使用红外遥控报警器具有重要参考价值。
原理图提供了报警器设计的电路结构,显示了发射器和接收器的工作原理和相互作用方式。PCB图(印刷电路板图)则展示了电路元件的布局,对于实际生产制造电路板十分关键。程序则包含了报警器的控制逻辑代码,通常是用某种编程语言实现的,如C语言或汇编语言,这些代码会在微控制器上运行以控制整个报警系统的行为。说明文档则详细解释了产品的安装、配置和操作步骤,对于用户理解和正确使用设备至关重要。
综合来看,文件中的这些资源能够帮助用户更好地了解红外遥控报警器的工作原理,为设计、制造和调试提供了必要的信息。无论是专业的电子工程师还是对电子技术感兴趣的爱好者,这些资源都是宝贵的参考资料。