The frame range is smaller than the flipbook tile count. The flipbook will contain some blank tiles. Python error: Traceback (most recent call last): File "<stdin>", line 1, in <module> File "labs::Driver/flipbook_textures::1.0, PythonModule", line 1328, in renderAndExport File "labs::Driver/flipbook_textures::1.0, PythonModule", line 1122, in renderAll File "labs::Driver/flipbook_textures::1.0, PythonModule", line 1115, in renderAll File "labs::Driver/flipbook_textures::1.0, PythonModule", line 1084, in renderDepth File "labs::Driver/flipbook_textures::1.0, PythonModule", line 1077, in renderDepth File "C:\PROGRA~1/SIDEEF~1/HOUDIN~1.303/houdini/python3.9libs\houpythonportion\ui.py", line 1112, in decorator return func(*args, **kwargs) File "C:\PROGRA~1/SIDEEF~1/HOUDIN~1.303/houdini/python3.9libs\hou.py", line 74137, in render return _hou.RopNode_render(self, *args, **kwargs) hou.OperationFailed: The attempted operation failed. Error: The specified resolution exceeds the maximum of 1280x720 allowed in the Non-Commercial Edition.
时间: 2024-04-27 15:19:15 浏览: 182
这个错误包含了两个问题:
1. 第一个问题是帧范围小于翻页瓷砖数,这意味着翻页将包含一些空白瓷砖。你需要增加帧范围或减少翻页瓷砖数,以确保每个瓷砖都有对应的帧图像。
2. 第二个问题是分辨率超出了非商业版允许的最大分辨率1280x720。如果你正在使用非商业版Houdini,你需要将分辨率降低到1280x720或以下,或者升级到商业版以支持更高的分辨率。如果你已经在使用商业版,你需要检查设置中的分辨率是否正确设置,并且确保你的系统可以支持所需的分辨率。
相关问题
pytorch部分代码如下:train_loss, train_acc = train(model_ft, DEVICE, train_loader, optimizer, epoch,model_ema) if use_amp: with torch.cuda.amp.autocast(): # 开启混合精度 loss = torch.nan_to_num(criterion_train(output, targets)) # 计算loss scaler.scale(loss).backward() # 梯度放大 torch.nn.utils.clip_grad_norm_(model.parameters(), CLIP_GRAD) if not (self._backward_hooks or self._forward_hooks or self._forward_pre_hooks or _global_backward_hooks or global_forward_hooks or global_forward_pre_hooks): return forward_call(*input, **kwargs) class LDAMLoss(nn.Module): def init(self, cls_num_list, max_m=0.5, weight=None, s=30): super(LDAMLoss, self).init() m_list = 1.0 / np.sqrt(np.sqrt(cls_num_list)) m_list = m_list * (max_m / np.max(m_list)) m_list = torch.cuda.FloatTensor(m_list) self.m_list = m_list assert s > 0 self.s = s self.weight = weight def forward(self, x, target): index = torch.zeros_like(x, dtype=torch.uint8) index.scatter(1, target.data.view(-1, 1).type(torch.int64), 1) index_float = index.type(torch.cuda.FloatTensor) batch_m = torch.matmul(self.m_list[None, :], index_float.transpose(0,1)) batch_m = batch_m.view((-1, 1)) x_m = x - batch_m output = torch.where(index, x_m, x) return F.cross_entropy(self.s*output, target, weight=self.weight) 报错:Traceback (most recent call last): File "/home/adminis/hpy/ConvNextV2_Demo/train+ca.py", line 279, in <module> train_loss, train_acc = train(model_ft, DEVICE, train_loader, optimizer, epoch,model_ema) File "/home/adminis/hpy/ConvNextV2_Demo/train+ca.py", line 46, in train loss = torch.nan_to_num(criterion_train(output, targets)) # 计算loss File "/home/adminis/anaconda3/envs/wln/lib/python3.9/site-packages/torch/nn/modules/module.py", line 1051, in call_impl return forward_call(*input, **kwargs) File "/home/adminis/hpy/ConvNextV2_Demo/models/utils.py", line 621, in forward index.scatter(1, target.data.view(-1, 1).type(torch.int64), 1) RuntimeError: Expected index [112, 1] to be smaller than self [16, 7] apart from dimension 1
根据你提供的代码和错误信息,可以看出问题是在`LDAMLoss`模块的`forward`函数中。具体来说,`index.scatter()`函数的第一个参数是一个张量,这个张量用于指示哪些元素需要被更新。在你的代码中,`index`是一个由0和1组成的布尔型张量,用于指示哪些类需要被更新。第二个参数是需要被更新的元素的索引,这里使用了`target.data.view(-1, 1).type(torch.int64)`。由于`target`的形状是`(batch_size,)`,因此`target.data.view(-1, 1)`将其变形为`(batch_size, 1)`的张量,而`.type(torch.int64)`将其转换为整型。这样,第二个参数的形状是`(batch_size, 1)`。
但是,由于`index`的形状是`(batch_size, num_classes)`,其中`num_classes`是类别数,因此`index.scatter()`函数期望的第一个参数的形状应该与`index`相同,即`(batch_size, num_classes)`。由于这里的错误信息是`Expected index [112, 1] to be smaller than self [16, 7] apart from dimension 1`,这表明`index`的形状是`(16, 7)`,而第二个参数的形状是`(112, 1)`,这与期望的形状不匹配。因此,你需要检查`target`的形状是否正确,并确保`index`和第二个参数具有相同的形状。
另外,你的`LDAMLoss`模块中还有一些其他的问题。例如,在`__init__`函数中,应该调用`super().__init__()`而不是`super(LDAMLoss, self).__init__()`,因为后者是Python 2的用法。此外,在计算`batch_m`时,你可以使用`torch.sum()`函数来代替矩阵乘法运算。
pytorch部分代码如下:train_loss, train_acc = train(model_ft, DEVICE, train_loader, optimizer, epoch,model_ema) if use_amp: with torch.cuda.amp.autocast(): # 开启混合精度 loss = torch.nan_to_num(criterion_train(output, targets)) # 计算loss scaler.scale(loss).backward() # 梯度放大 torch.nn.utils.clip_grad_norm_(model.parameters(), CLIP_GRAD) if not (self._backward_hooks or self._forward_hooks or self._forward_pre_hooks or _global_backward_hooks or global_forward_hooks or global_forward_pre_hooks): return forward_call(input, **kwargs) class LDAMLoss(nn.Module): def init(self, cls_num_list, max_m=0.5, weight=None, s=30): super(LDAMLoss, self).init() m_list = 1.0 / np.sqrt(np.sqrt(cls_num_list)) m_list = m_list * (max_m / np.max(m_list)) m_list = torch.cuda.FloatTensor(m_list) self.m_list = m_list assert s > 0 self.s = s self.weight = weight def forward(self, x, target): index = torch.zeros_like(x, dtype=torch.uint8) index.scatter(1, target.data.view(-1, 1).type(torch.int64), 1) index_float = index.type(torch.cuda.FloatTensor) batch_m = torch.matmul(self.m_list[None, :], index_float.transpose(0,1)) batch_m = batch_m.view((-1, 1)) x_m = x - batch_m output = torch.where(index, x_m, x) return F.cross_entropy(self.soutput, target, weight=self.weight) 报错:Traceback (most recent call last): File "/home/adminis/hpy/ConvNextV2_Demo/train+ca.py", line 279, in <module> train_loss, train_acc = train(model_ft, DEVICE, train_loader, optimizer, epoch,model_ema) File "/home/adminis/hpy/ConvNextV2_Demo/train+ca.py", line 46, in train loss = torch.nan_to_num(criterion_train(output, targets)) # 计算loss File "/home/adminis/anaconda3/envs/wln/lib/python3.9/site-packages/torch/nn/modules/module.py", line 1051, in call_impl return forward_call(*input, **kwargs) File "/home/adminis/hpy/ConvNextV2_Demo/models/utils.py", line 621, in forward index.scatter(1, target.data.view(-1, 1).type(torch.int64), 1) RuntimeError: Expected index [112, 1] to be smaller than self [16, 7] apart from dimension 1 帮我看看如何修改
根据你提供的代码,可以看到报错信息来自于 LDAMLoss 类的 forward 函数中的 index.scatter 函数。这个函数的作用是将 target 中的每个元素在 index 中对应的位置上设为 1,其他位置设为 0。但是报错信息显示 index 的形状是 [112, 1],而 LDAMLoss 模型的输出 output 的形状是 [16, 7],因此 index 与 output 的形状不匹配,导致了这个错误。
为了解决这个问题,你需要检查一下你的代码,看看是否有错误的数据维度或者参数传递。你可以尝试在 forward 函数中添加一些打印语句,输出 index 和 target 的形状,以及其他相关的参数信息,以便更好地理解问题所在。另外,你也可以检查一下你的模型输出和标签的形状是否匹配,以及数据加载器是否正确地加载数据。
阅读全文
相关推荐
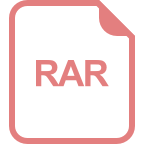
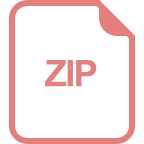
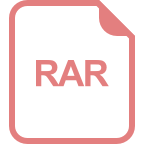













