self.linears = nn.ModuleList( [ nn.Linear(2,num_units), nn.ReLU(), nn.Linear(num_units,num_units), nn.ReLU(), nn.Linear(num_units,num_units), nn.ReLU(), nn.Linear(num_units,2), ] )代码的理解
时间: 2024-01-24 22:02:22 浏览: 71
这段代码定义了一个包含多个线性层和激活函数的神经网络模型。具体来说,这个模型包含4个线性层,其中前3个线性层后面跟着一个ReLU激活函数,最后一个线性层没有激活函数。这个模型的输入维度是2,输出维度是2。其中`num_units`是一个超参数,表示每个线性层的神经元数量。`nn.ModuleList`是一个PyTorch中的容器,用于管理神经网络中的各个子模块。这里使用`ModuleList`把多个线性层和激活函数组成一个列表,方便进行参数的管理和传递。
相关问题
self.linears = nn.ModuleList( [ nn.Linear(2,num_units), nn.ReLU(), nn.Linear(num_units,num_units), nn.ReLU(), nn.Linear(num_units,num_units), nn.ReLU(), nn.Linear(num_units,2), ] )的分析
这段代码定义了一个含有四个线性层和三个ReLU激活函数的神经网络,用于完成一个二维的回归任务。具体来说,这个神经网络的输入是二维向量,输出是一个二维向量,每个向量元素都是实数。其中,num_units是一个超参数,表示每个线性层的输出维度,即隐藏单元的数量。神经网络的结构如下:
- 第1个线性层:输入为2,输出为num_units
- 第1个ReLU激活函数:对第1个线性层的输出进行ReLU激活
- 第2个线性层:输入为num_units,输出为num_units
- 第2个ReLU激活函数:对第2个线性层的输出进行ReLU激活
- 第3个线性层:输入为num_units,输出为num_units
- 第3个ReLU激活函数:对第3个线性层的输出进行ReLU激活
- 第4个线性层:输入为num_units,输出为2
这个神经网络的输出可以用于回归任务,例如预测一个二维数据点的坐标值。需要注意的是,这个神经网络是使用ModuleList定义的,可以通过索引访问其中的每个模块,方便进行参数的初始化和优化。
def __init__(self,n_steps,num_units=128): super(MLPDiffusion,self).__init__() self.linears = nn.ModuleList( [ nn.Linear(2,num_units), nn.ReLU(), nn.Linear(num_units,num_units), nn.ReLU(), nn.Linear(num_units,num_units), nn.ReLU(), nn.Linear(num_units,2), ] ) self.step_embeddings = nn.ModuleList( [ nn.Embedding(n_steps,num_units), nn.Embedding(n_steps,num_units), nn.Embedding(n_steps,num_units), ] )代码的解释】
这段代码定义了一个名为MLPDiffusion的类,它继承自PyTorch的nn.Module类。该类包含了三个成员变量:linears、step_embeddings和n_steps。其中,linears是一个包含四个线性层和三个ReLU激活函数的神经网络模型,用于对输入进行多层线性变换和非线性变换。step_embeddings是三个Embedding层,用于将输入的时间步编码为向量表示。n_steps是一个整数,表示输入序列的时间步数。
在MLPDiffusion类的初始化函数__init__中,首先调用了父类nn.Module的初始化函数。然后使用nn.ModuleList创建了linears和step_embeddings两个ModuleList。其中,linears包含四个线性层和三个ReLU激活函数,step_embeddings包含三个Embedding层。利用Embedding层可以将时间步的整数编码为向量表示,这里Embedding层的输出向量维度与线性层的输出向量维度相同。在这个模型中,每个Embedding层的向量维度为num_units。最后,将输入序列的时间步数n_steps作为类的成员变量。
阅读全文
相关推荐
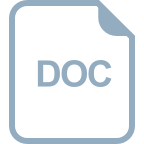
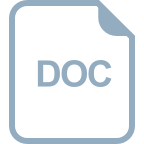












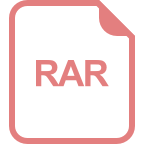


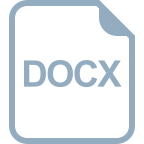