注意力机制代码_深入理解图注意力机制(Graph Attention Network)
时间: 2023-07-03 10:26:26 浏览: 79
好的,关于注意力机制的代码,我们先来了解一下图注意力机制(Graph Attention Network,简称GAT)。
GAT是一种基于图结构的注意力机制,它在图结构中进行节点分类、图分类等任务时,能够自适应地学习每个节点在不同邻居节点上所占的重要性,从而更准确地进行分类。下面是一个简单的GAT实现:
```python
import torch
import torch.nn as nn
import torch.nn.functional as F
class GraphAttentionLayer(nn.Module):
def __init__(self, in_features, out_features, dropout, alpha, concat=True):
super(GraphAttentionLayer, self).__init__()
self.in_features = in_features
self.out_features = out_features
self.dropout = dropout
self.alpha = alpha
self.concat = concat
self.W = nn.Parameter(torch.zeros(size=(in_features, out_features)))
nn.init.xavier_uniform_(self.W.data, gain=1.414)
self.a = nn.Parameter(torch.zeros(size=(2*out_features, 1)))
nn.init.xavier_uniform_(self.a.data, gain=1.414)
self.leakyrelu = nn.LeakyReLU(self.alpha)
def forward(self, h, adj):
Wh = torch.mm(h, self.W)
a_input = self._prepare_attentional_mechanism_input(Wh)
e = self.leakyrelu(torch.matmul(a_input, self.a).squeeze(2))
zero_vec = -9e15*torch.ones_like(e)
attention = torch.where(adj > 0, e, zero_vec)
attention = F.softmax(attention, dim=1)
attention = F.dropout(attention, self.dropout, training=self.training)
h_prime = torch.matmul(attention, Wh)
if self.concat:
return F.elu(h_prime)
else:
return h_prime
def _prepare_attentional_mechanism_input(self, Wh):
N = Wh.size()[0]
Wh_repeated_in_chunks = Wh.repeat_interleave(N, dim=0)
Wh_repeated_alternating = Wh.repeat(N, 1)
all_combinations_matrix = torch.cat([Wh_repeated_in_chunks, Wh_repeated_alternating], dim=1)
return all_combinations_matrix.view(N, N, 2 * self.out_features)
```
在这个代码中,我们定义了一个名为GraphAttentionLayer的类,它继承于nn.Module类。在它的__init__方法中,我们定义了一些必要的参数,包括输入特征维度、输出特征维度、dropout率、LeakyReLU函数的负斜率系数以及是否将节点特征与注意力机制的输出进行拼接。W和a是需要学习的参数,其中W是线性变换的权重矩阵,a是注意力机制的权重矩阵。我们使用xavier_uniform_方法对这两个参数进行初始化。
在forward方法中,我们首先将节点特征矩阵h与权重矩阵W相乘,得到Wh。然后,我们通过_prepare_attentional_mechanism_input方法将Wh转换为用于注意力计算的输入矩阵a_input。接着,我们将a_input与注意力权重矩阵a相乘,得到每个节点与其邻居之间的注意力系数e。我们使用LeakyReLU函数将e中的负值裁剪掉。然后,我们对每个节点的邻居节点计算softmax,得到它们之间的注意力权重。我们使用dropout对注意力权重进行随机失活,以防止过拟合。最后,我们将注意力权重与Wh相乘,得到每个节点的新特征h_prime。如果concat参数为True,我们将h_prime与原始节点特征进行拼接并使用ELU函数作为输出;否则,我们直接返回h_prime作为输出。
需要注意的是,这个代码中的实现是基于PyTorch框架的,如果你使用其他的深度学习框架,可能需要做一些调整。同时,这个代码只是GAT的一个简单实现,如果你想深入学习GAT,还需要阅读相关论文并了解更多细节。
相关推荐
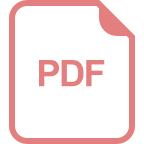
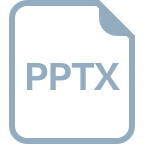
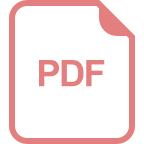
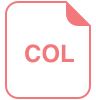
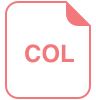
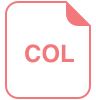
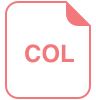









