contrast_a = np.take(temp_ab[1], indicesa)
时间: 2024-03-27 07:36:22 浏览: 13
`np.take()` 是一个从数组中提取元素的函数,它的使用方法如下:
```python
numpy.take(a, indices, axis=None, out=None, mode='raise')
```
其中,`a` 是需要提取元素的数组,`indices` 是需要提取元素的索引,`axis` 是需要提取元素的轴,`out` 是输出数组,`mode` 是越界处理模式。
具体来说,`np.take()` 函数会按照 `indices` 中的索引,从 `a` 数组中提取对应的元素,然后将这些元素组成一个新的数组返回。如果 `indices` 中的某个索引超出了 `a` 数组的索引范围,则根据 `mode` 参数的不同采取不同的处理方式。
下面是一个示例:
```python
import numpy as np
a = np.array([[1, 2], [3, 4]])
indices = [1, 0, 1, 0]
b = np.take(a, indices)
print(b) # 输出 [2 1 2 1]
```
在上面的示例中,`np.take()` 函数将原数组 `a` 中按照 `indices` 中的索引提取元素,得到的结果是 `[2, 1, 2, 1]`。其中,`indices` 中的第一个索引是 1,表示提取 `a` 数组中第二行第一个元素,因此结果中的第一个元素是 2;`indices` 中的第二个索引是 0,表示提取 `a` 数组中第一行第一个元素,因此结果中的第二个元素是 1;以此类推。
相关问题
解释代码contrast_count = features.shape[1]
代码`contrast_count = features.shape[1]`的含义是获取`features`张量的形状,并将其第二个维度的大小赋值给变量`contrast_count`。
`features.shape`返回一个元组,表示`features`张量的形状。元组的长度对应于张量的维度数,而每个元素表示该维度的大小。
通过索引操作`features.shape[1]`,我们可以获取元组中第二个元素的值,即表示第二个维度的大小。将这个值赋给`contrast_count`变量,即将`contrast_count`设置为`features`张量的第二个维度的大小。
这个代码片段的目的是为了获得`features`张量在第二个维度上的大小,并将其保存在名为`contrast_count`的变量中。
from PIL import Image img = Image.open("red1.jpg") lab_img = img.convert("LAB") l, a, b = lab_img.split() a = a.point(lambda i: int(i * 0.5)) a = a.contrast(1.5) lab_img = Image.merge("LAB", (l, a, b)) rgb_img = lab_img.convert("RGB") rgb_img.save("red3.jpg")报错AttributeError: contrast
这个错误是因为 `PIL` 库中的 `Image` 对象没有 `contrast` 属性。你可以尝试使用 `ImageEnhance` 模块中的 `Contrast` 类来增加图像的对比度。具体的代码如下所示:
```python
from PIL import Image, ImageEnhance
img = Image.open("red1.jpg")
lab_img = img.convert("LAB")
l, a, b = lab_img.split()
a = a.point(lambda i: int(i * 0.5))
enhancer = ImageEnhance.Contrast(a)
a = enhancer.enhance(1.5)
lab_img = Image.merge("LAB", (l, a, b))
rgb_img = lab_img.convert("RGB")
rgb_img.save("red3.jpg")
```
这段代码将图像转换为 `LAB` 颜色空间,然后对 `a` 通道进行了亮度减半的处理,并使用 `ImageEnhance.Contrast` 类来增加对比度。最后将图像转换回 `RGB` 颜色空间并保存。
相关推荐
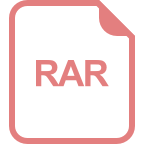













