module 'tensorflow._api.v1.keras.layers' has no attribute 'MultiHeadAttention'
时间: 2023-07-06 21:31:26 浏览: 152
这个错误可能是因为你使用的TensorFlow版本不支持MultiHeadAttention层。MultiHeadAttention是在TensorFlow 2.0及以上版本中引入的。如果你使用的是TensorFlow 1.x版本,那么这个层是不存在的,因此会出现上述错误。
如果你正在使用TensorFlow 2.0及以上版本,那么可能是因为你没有正确导入MultiHeadAttention层。正确的导入方式应该是:
```
from tensorflow.keras.layers import MultiHeadAttention
```
请确保你的TensorFlow版本正确,并且导入方式正确,如果问题仍然存在,请检查你的代码实现是否存在其他问题。
相关问题
module 'tensorflow.python.keras.api._v1.keras.layers' has no attribute 'batch_norm'
The error message suggests that there is no attribute called `batch_norm` in the `keras.layers` module of TensorFlow 1.x.
It is possible that you are using an outdated version of TensorFlow, or the attribute has been removed or renamed in a newer version.
In TensorFlow 1.x, the equivalent attribute is `BatchNormalization`. You can try importing it as follows:
```python
from tensorflow.keras.layers import BatchNormalization
```
If you are using TensorFlow 2.x, the attribute is still `BatchNormalization`. However, the import statement would be:
```python
from tensorflow.keras.layers import BatchNormalization
```
Make sure you have the correct version of TensorFlow installed and import the attribute using the correct syntax based on the version you are using.
module 'tensorflow._api.v2.train' has no attribute 'Saver'
在 TensorFlow 2 中,`tf.train.Saver()` 已被移除,取而代之的是使用 `tf.saved_model` 模块保存和恢复模型。如果您想使用旧版本的 Saver,可以在 TensorFlow 2 中使用 `tf.compat.v1.train.Saver()`。
以下是一个使用 `tf.saved_model` 模块保存和恢复模型的示例:
```python
import tensorflow as tf
# 定义模型
model = tf.keras.models.Sequential([
tf.keras.layers.Dense(128, input_shape=(784,)),
tf.keras.layers.Dense(10)
])
# 编译模型
model.compile(optimizer='adam',
loss=tf.keras.losses.SparseCategoricalCrossentropy(from_logits=True),
metrics=['accuracy'])
# 训练模型
model.fit(train_images, train_labels, epochs=10)
# 保存模型
tf.saved_model.save(model, '/path/to/my_model')
# 加载模型
loaded_model = tf.saved_model.load('/path/to/my_model')
```
在这个例子中,我们使用 `tf.saved_model.save()` 方法将模型保存到磁盘上,然后使用 `tf.saved_model.load()` 方法将模型加载回来。注意,这两个方法都返回一个 `tf.saved_model` 对象,而不是一个 Saver 对象。
阅读全文
相关推荐
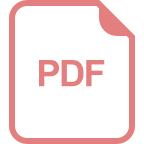
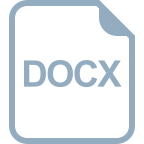
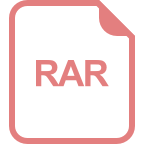













