请解释以下代码 month=xr.DataAraay(['Jan','Jun','Jan'],[data_array.coords['y']],name='month')
时间: 2024-01-12 18:04:23 浏览: 89
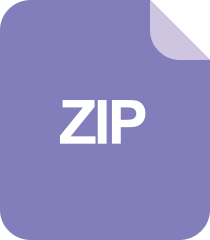
月份英文显示

这段代码使用了 xarray 库,用于创建一个名为 "month" 的 Xarray 数据数组。该数组包含三个元素,分别是 "Jan"、"Jun" 和 "Jan",这些元素对应着 data_array 数据数组的 y 坐标轴上的三个位置。
具体来说,这里使用了 xr.DataArray() 函数,该函数的第一个参数是数据数组的值,第二个参数是数据数组的坐标轴,第三个参数是数组的名称。在这里,数据数组的值是一个包含三个字符串元素的列表 "['Jan','Jun','Jan']",第二个参数是 data_array 数据数组的 y 坐标轴,第三个参数是 "month"。由于 y 坐标轴只有一个维度,所以第二个参数也只包含了一个元素,即 "[data_array.coords['y']]"。
阅读全文
相关推荐
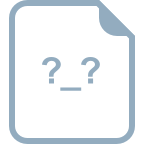
















