sensor.set_pixformat(sensor.RGB565) sensor.set_framesize(sensor.QVGA) sensor.skip_frames(time = 100)
时间: 2024-05-20 07:12:53 浏览: 19
这段代码是在MicroPython中使用OpenMV相机模块时的一些设置。
- `sensor.set_pixformat(sensor.RGB565)` 设置像素格式为RGB565,即每个像素由16比特的颜色值表示。
- `sensor.set_framesize(sensor.QVGA)` 设置帧大小为QVGA(Quarter VGA),即320x240像素。
- `sensor.skip_frames(time=100)` 跳过100毫秒的帧以让相机调整曝光和白平衡。
这些设置可以根据需要进行调整。
相关问题
import sensor, image, time, math # 初始化摄像头 sensor.reset() sensor.set_pixformat(sensor.RGB565) sensor.set_framesize(sensor.QVGA) sensor.set_windowing((240, 240)) sensor.skip_frames(time=2000) # 加载人脸检测器 face_cascade = image.HaarCascade("frontalface", stage = 10) while(True): # 拍摄一张图片并进行人脸检测 img = sensor.snapshot() faces = img.find_features(face_cascade, threshold=0.5, scale_factor=1.5) # 如果检测到了人脸 if faces: for r in faces: img.draw_rectangle(r)我要如何获得生成的矩形框的中心坐标
可以通过以下方式获得矩形框的中心坐标:
```python
if faces:
for r in faces:
img.draw_rectangle(r)
# 获取矩形框的中心坐标
x = r.x() + r.w() // 2
y = r.y() + r.h() // 2
print("矩形框的中心坐标为:({},{})".format(x, y))
```
其中 `r.x()` 和 `r.y()` 分别表示矩形框左上角的 x 坐标和 y 坐标,`r.w()` 和 `r.h()` 分别表示矩形框的宽度和高度,所以中心坐标可以通过加上一半的宽度和高度来计算出来。
帮我分析这段代码import sensor, image, time, os, tf sensor.reset() # Reset and initialize the sensor. sensor.set_pixformat(sensor.GRAYSCALE) # Set pixel format to RGB565 (or GRAYSCALE) sensor.set_framesize(sensor.QVGA) # Set frame size to QVGA (320x240) sensor.set_windowing((240, 240)) # Set 240x240 window. sensor.skip_frames(time=2000) # Let the camera adjust. clock = time.clock() while(True): clock.tick() img = sensor.snapshot().binary([(0,64)]) for obj in tf.classify("trained.tflite", img, min_scale=1.0, scale_mul=0.5, x_overlap=0.0, y_overlap=0.0): output = obj.output() number = output.index(max(output)) print(number) print(clock.fps(), "fps")
这段代码是在使用OpenMV摄像头进行目标识别。具体分析如下:
1. 导入需要的模块:sensor、image、time、os和tf
2. 对摄像头进行初始化设置:
a. reset()函数重置并初始化摄像头。
b. set_pixformat()函数将像素格式设置为灰度图像。
c. set_framesize()函数将帧大小设置为QVGA(320x240)。
d. set_windowing()函数将窗口设置为240x240。
e. skip_frames()函数让摄像头进行自动调整。
3. 定义一个time.clock()对象用于计时。
4. 进入while循环,不断地进行目标识别。
5. 使用sensor.snapshot()函数获取实时图像,并将其转换为二值图像,使用binary()函数将图像二值化,只保留黑色和白色两种像素值。
6. 使用tf.classify()函数进行目标分类,传入参数为训练好的模型文件、处理后的图像、最小缩放比例、缩放比例步长、x轴重叠率和y轴重叠率。
7. 对分类结果进行处理,使用obj.output()函数获取输出值,输出值是一个列表,每个元素表示对应类别的可能性,选择其中最大的一个作为分类结果,并使用print()函数输出分类结果。
8. 使用time.clock()计算处理一帧图像所需的时间,使用clock.fps()函数计算处理帧率,并使用print()函数输出处理帧率。
相关推荐
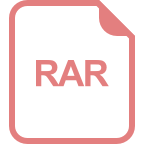
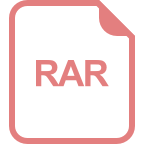
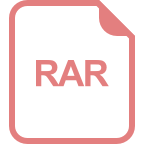












