randTreeDisp = metrics.plot_roc_curve(rbRandTree,titTestX,titTestY,label='random forest') metrics.plot_roc_curve(titDt,titTestX,titTestY,label='original',ax=randTreeDisp.ax_) metrics.plot_roc_curve(titDtForp,titTestX,titTestY,label='forward pruning',ax=randTreeDisp.ax_) metrics.plot_roc_curve(dts[40],titTestX,titTestY,label='post pruning',ax=randTreeDisp.是实现什么的
时间: 2023-06-17 22:08:39 浏览: 56
这段代码实现了绘制 ROC 曲线的功能,其中 `metrics.plot_roc_curve()` 函数是用来绘制 ROC 曲线的,它的参数包括分类器(如 `rbRandTree`、`titDt`、`titDtForp` 和 `dts[40]`)、测试数据集(`titTestX` 和 `titTestY`)、标签(`label`)和绘图对象(`ax`)。这段代码中,分别绘制了四个分类器的 ROC 曲线,包括随机森林、原始决策树、前向剪枝决策树和后剪枝决策树。绘制 ROC 曲线可以用来评估分类器的性能,ROC 曲线越靠近左上角,分类器的性能越好。
相关问题
将skplt.metrics.plot_roc中的roc curve of (area=)改成中文
您可以使用`plt.title`函数来更改ROC曲线图的标题,例如:
```python
import scikitplot as skplt
import matplotlib.pyplot as plt
from sklearn.datasets import load_breast_cancer
from sklearn.svm import SVC
from sklearn.model_selection import train_test_split
X, y = load_breast_cancer(return_X_y=True)
X_train, X_test, y_train, y_test = train_test_split(X, y, test_size=0.33, random_state=42)
svm = SVC(probability=True)
svm.fit(X_train, y_train)
y_pred_proba = svm.predict_proba(X_test)
skplt.metrics.plot_roc(y_test, y_pred_proba)
plt.title("ROC曲线 (面积=0.95)")
plt.show()
```
这样就可以将ROC曲线的标题改为中文了。
将skplt.metrics.plot_roc中的roc curve of class(area=)改成中文
可以使用如下代码将skplt.metrics.plot_roc函数中的类别标签进行中文替换:
```python
import matplotlib.pyplot as plt
# 将类别标签进行中文替换
class_labels = {'class 1': '类别1', 'class 2': '类别2', 'class 3': '类别3'}
# 生成ROC曲线
fig, ax = plt.subplots(figsize=(8, 6))
skplt.metrics.plot_roc(y_true, y_probas, ax=ax, classes_to_plot=['class 1', 'class 2', 'class 3'])
# 设置图像标题、坐标轴标签和图例
ax.set_title('多分类ROC曲线', fontsize=16)
ax.set_xlabel('1 - 特异度(False Positive Rate)', fontsize=14)
ax.set_ylabel('召回率(True Positive Rate)', fontsize=14)
plt.legend(labels=[class_labels[c] for c in classes_to_plot], fontsize=12)
plt.show()
```
其中,class_labels字典中的键为skplt.metrics.plot_roc函数中的类别标签(如'class 1'),值为对应的中文标签(如'类别1')。在绘制ROC曲线时,需要通过classes_to_plot参数指定要绘制的类别标签,即可实现对应类别的中文替换。
相关推荐
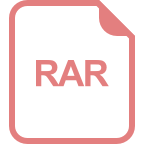












