X_train,X_test,y_train,y_test=train_test_split(X ,y,test_size=0.2,random_state=1) from sklearn import tree DecTreeModel = tree.DecisionTreeClassifier().fit(X_train, y_train) preds = DecTreeModel.predict(X_test) print('accuracy', metrics.accuracy_score(y_test, preds)) print('recall', metrics.recall_score(y_test, preds)) print('precision', metrics.precision_score(y_test, preds)) print('f1-score', metrics.f1_score(y_test, preds)) print(classification_report(y_test, preds)) y_scores = DecTreeModel.predict_proba(X_test) from sklearn.metrics import roc_curve from sklearn.metrics import confusion_matrix import matplotlib import matplotlib.pyplot as plt %matplotlib inline # calculate ROC curve fpr, tpr, thresholds = roc_curve(y_test, y_scores[:,1]) # plot ROC curve fig = plt.figure(figsize=(16, 8)) # Plot the diagonal 50% line plt.plot([0, 1], [0, 1], 'k--') # Plot the FPR and TPR achieved by our model plt.plot(fpr, tpr) plt.xlabel('False Positive Rate') plt.ylabel('True Positive Rate') plt.title('ROC Curve') plt.show()
时间: 2024-04-01 20:38:10 浏览: 53
这段代码实现了一个基于决策树的分类器,并对其性能进行了评估和可视化分析。首先,使用 train_test_split 函数将数据集分为训练集和测试集,然后使用决策树分类器进行训练,预测测试集上的结果并计算准确率、召回率、精确度和 F1 得分等指标。接着,使用 predict_proba 函数计算测试集上的预测得分,然后使用 roc_curve 函数计算接收器操作特征曲线(ROC 曲线)的 FPR 和 TPR,最后使用 Matplotlib 库绘制 ROC 曲线,并且将其展示出来。
相关问题
X_train, X_test, y_train, y_test = train_test_split(X, y, test_size=0.3, random_state=42) X_val, X_test, y_val, y_test = train_test_split(X_test, y_test, test_size=0.5, random_state=42)
这段代码是用于将数据集划分为训练集、验证集和测试集,并按照一定比例进行划分。
首先,使用train_test_split函数将原始数据集X和标签y按照test_size参数的比例(30%)划分为训练集X_train和y_train,测试集X_test和y_test。
接着,再次使用train_test_split函数,将X_test和y_test按照test_size参数的比例(50%)划分为验证集X_val和y_val,测试集X_test和y_test。
其中,random_state参数用于设置随机种子,可以保证每次运行代码时得到相同的随机结果,以便于重现实验结果。
划分数据集的目的是为了在模型训练过程中进行验证和测试,以评估模型的性能和泛化能力。训练集用于模型训练,验证集用于模型调参和选择最优模型,测试集用于最终评估模型的性能。
def split_data(): test_size = float(entry.get()) X_train, X_test, y_train, y_test = train_test_split(X, y, test_size=test_size, random_state=0) return X_train, X_test, y_train, y_test X_train, X_test, y_train, y_test = split_data() # 在函数外部调用split_data函数并保存返回值到全局变量中报错could not convert string to float:
这个错误通常是因为`entry.get()`返回的字符串不能被转换为浮点数。你可以检查一下`entry.get()`返回的具体内容是否为一个有效的浮点数,例如:
```
test_size_str = entry.get()
print(test_size_str)
test_size = float(test_size_str)
```
如果`entry.get()`返回的不是一个浮点数的字符串,你需要确保用户输入的是一个有效的浮点数,或者你可以添加一些异常处理代码来处理这种情况,例如:
```
try:
test_size = float(entry.get())
except ValueError:
print("Invalid input, please enter a valid float number.")
```
阅读全文
相关推荐
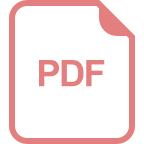
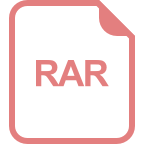













