cuda = not cpu and torch.cuda.is_available()
时间: 2023-11-13 18:40:31 浏览: 111
这行代码是用来判断是否可以使用 GPU 运行 PyTorch 的程序。它首先判断是否当前系统中有可用的 GPU(即 torch.cuda.is_available() 是否为 True),如果有可用的 GPU,则将 cuda 变量设置为 True;否则将其设置为 False,表示只能在 CPU 上运行 PyTorch。这个判断语句一般用于在程序中选择使用 GPU 还是 CPU 运行 PyTorch。
相关问题
try: import thop except ImportError: thop = None logger = logging.getLogger(__name__) @contextmanager def torch_distributed_zero_first(local_rank: int): if local_rank not in [-1, 0]: torch.distributed.barrier() yield if local_rank == 0: torch.distributed.barrier() def init_torch_seeds(seed=0): torch.manual_seed(seed) if seed == 0: cudnn.benchmark, cudnn.deterministic = False, True else: cudnn.benchmark, cudnn.deterministic = True, False def select_device(device='', batch_size=None): s = f'YOLOv5 🚀 {git_describe() or date_modified()} torch {torch.__version__} ' cpu = device.lower() == 'cpu' if cpu: os.environ['CUDA_VISIBLE_DEVICES'] = '-1' elif device: # non-cpu device requested os.environ['CUDA_VISIBLE_DEVICES'] = device assert torch.cuda.is_available(), f'CUDA unavailable, invalid device {device} requested' cuda = not cpu and torch.cuda.is_available() if cuda: n = torch.cuda.device_count() if n > 1 and batch_size: # check that batch_size is compatible with device_count assert batch_size % n == 0, f'batch-size {batch_size} not multiple of GPU count {n}' space = ' ' * len(s) for i, d in enumerate(device.split(',') if device else range(n)): p = torch.cuda.get_device_properties(i) s += f"{'' if i == 0 else space}CUDA:{d} ({p.name}, {p.total_memory / 1024 ** 2}MB)\n" s += 'CPU\n' logger.info(s.encode().decode('ascii', 'ignore') if platform.system() == 'Windows' else s) # emoji-safe return torch.device('cuda:0' if cuda else 'cpu') def time_synchronized(): if torch.cuda.is_available(): torch.cuda.synchronize() return time.time()
这段代码是一个Python脚本,包含了一些用于PyTorch训练的实用函数。具体来说,它包含了以下函数:
- import thop:导入PyTorch的模块thop,用于计算模型的FLOPs和参数数量。
- torch_distributed_zero_first:用于在分布式训练中同步所有进程的执行顺序。
- init_torch_seeds:用于初始化PyTorch的随机数种子。
- select_device:用于选择PyTorch的设备,可以选择CPU或GPU。
- time_synchronized:用于在CPU和GPU之间同步时间。
这些函数都是用于方便PyTorch训练的实用函数,可以大大简化代码编写。
use_cuda = torch.cuda.is_available() FloatTensor = torch.cuda.FloatTensor if use_cuda else torch.FloatTensor device = torch.device("cpu") #使用GPU进行训练 from torch.autograd import Variable from replay_buffer import ReplayMemory, Transition # set up matplotlib is_ipython = 'inline' in matplotlib.get_backend() if is_ipython: from IPython import display #plt.ion() use_cuda = torch.cuda.is_available() FloatTensor = torch.cuda.FloatTensor if use_cuda else torch.FloatTensor device = torch.device("cpu")把这段代码改成CPU训练
您可以像这样将上述代码更改为在CPU上进行训练:
```
import torch
from torch.autograd import Variable
from replay_buffer import ReplayMemory, Transition
device = torch.device("cpu") # 使用CPU进行训练
# 设置matplotlib
is_ipython = 'inline' in matplotlib.get_backend()
if is_ipython:
from IPython import display
# 定义FloatTensor
FloatTensor = torch.FloatTensor
# 创建replay buffer
memory = ReplayMemory(10000)
# 将模型和优化器移动到CPU上
model = DQN().to(device)
optimizer = optim.Adam(model.parameters())
# 定义状态转换函数
def state_to_tensor(state):
return FloatTensor([state])
# 训练模型
for i in range(num_episodes):
state = env.reset()
for t in count():
# 获取动作
action = select_action(state)
# 执行动作并获取下一状态、奖励、是否结束
next_state, reward, done, _ = env.step(action.item())
# 转换状态和动作为张量
state_tensor = state_to_tensor(state)
action_tensor = LongTensor([action])
next_state_tensor = state_to_tensor(next_state)
# 存储状态转换
memory.push(state_tensor, action_tensor, next_state_tensor, reward)
# 更新状态
state = next_state
# 训练模型
optimize_model()
if done:
break
```
这里我们将使用CPU进行训练,所以我们将`device`设为`torch.device("cpu")`;将`FloatTensor`设为`torch.FloatTensor`即可。同时,我们还需要将模型和优化器移动到CPU上,可以使用`to`方法将它们移动到CPU上。
阅读全文
相关推荐
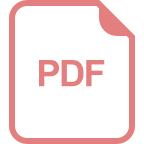












