dim = size(grad); result = zeros(dim,'uint8'); result(grad>0) = uint8(255); result(hsl>0) = uint8(255); figure() imshow(result)解释
时间: 2024-02-13 20:03:41 浏览: 21
这段代码的作用是将一个梯度图像(grad)和一个Hessian矩阵的值(hsl)大于零的像素点设置为白色(255),其余像素点设置为黑色(0),然后显示这个二值图像。具体来说,代码中的第一行计算了梯度图像(grad)的大小(dim),第二行创建一个与梯度图像大小相同的零矩阵(result),第三行将梯度图像(grad)和Hessian矩阵的值(hsl)大于零的像素点设置为白色(255),第四行将result矩阵的数据类型转换为8位无符号整型(uint8),第五行用imshow函数显示这个二值图像。
相关问题
Guided Grad-CAM如何使用
Guided Grad-CAM方法可以通过以下步骤实现:
1. 对输入图像进行预处理,包括归一化、缩放等操作。
2. 在模型中添加Guided Backpropagation模块,该模块通过反向传播特征图的梯度,并将与正激活相关的梯度保留。
3. 计算目标类别的Grad-CAM热力图,包括卷积层特征图的梯度和分类器权重的结合。
4. 对Grad-CAM热力图进行平滑处理,减少噪声和不确定性,并提高可视化效果。
5. 将平滑后的Grad-CAM热力图与Guided Backpropagation模块生成的梯度结合,生成Guided Grad-CAM热力图。
6. 将Guided Grad-CAM热力图与原始图像进行叠加,可视化模型的注意力区域。
下面是一个简单的代码示例,说明如何使用Guided Grad-CAM方法可视化图像分割模型的注意力区域:
```python
import torch
from torchvision import models, transforms
import cv2
import numpy as np
# 加载模型
model = models.segmentation.deeplabv3_resnet50(pretrained=True).eval()
# 定义预处理函数
preprocess = transforms.Compose([
transforms.ToTensor(),
transforms.Normalize(mean=[0.485, 0.456, 0.406], std=[0.229, 0.224, 0.225]),
])
# 定义反向传播函数
class GuidedBackpropRelu(torch.autograd.Function):
@staticmethod
def forward(ctx, input):
ctx.save_for_backward(input)
return input.clamp(min=0)
@staticmethod
def backward(ctx, grad_output):
input, = ctx.saved_tensors
grad_input = grad_output.clone()
grad_input[input < 0] = 0
return grad_input
# 定义Guided Grad-CAM函数
def guided_grad_cam(img, target_class):
# 转换为tensor格式
img_tensor = preprocess(img).unsqueeze(0)
# 前向传播
features = model.backbone(img_tensor)['out']
output = model.classifier(features)
# 计算梯度
target = output[0, target_class]
target.backward()
# 计算Grad-CAM热力图
grads = model.backbone.grads['out']
pooled_grads = torch.mean(grads, dim=[2, 3], keepdim=True)
cams = (pooled_grads * features).sum(dim=1, keepdim=True)
cams = torch.relu(cams)
# 平滑Grad-CAM热力图
sigma = 0.1
cams = torch.nn.functional.conv2d(cams, torch.ones((1, 1, 3, 3)).to(device=cams.device) / 9, padding=1, groups=1)
cams = torch.nn.functional.interpolate(cams, img_tensor.shape[-2:], mode='bilinear', align_corners=False)
cams = cams.squeeze().cpu().numpy()
cams = cv2.GaussianBlur(cams, (5, 5), sigmaX=sigma, sigmaY=sigma)
cams = np.maximum(cams, 0)
cams_max = cams.max()
if cams_max != 0:
cams /= cams_max
# 计算Guided Grad-CAM热力图
gb = model.backbone.grads['out']
gb = gb.cpu().numpy()[0]
cam_gb = np.zeros_like(cams)
for i, w in enumerate(model.classifier.weight[target_class]):
cam_gb += w * gb[i]
cam_gb = cv2.resize(cam_gb, img_tensor.shape[-2:])
cam_gb = np.maximum(cam_gb, 0)
cam_gb_max = cam_gb.max()
if cam_gb_max != 0:
cam_gb /= cam_gb_max
cam_gb = cv2.cvtColor(cv2.applyColorMap(np.uint8(255 * cam_gb), cv2.COLORMAP_JET), cv2.COLOR_BGR2RGB)
# 叠加Guided Grad-CAM热力图和原始图像
img = cv2.cvtColor(np.uint8(255 * img), cv2.COLOR_RGB2BGR)
cam_gb = cv2.resize(cam_gb, img.shape[:2][::-1])
result = cv2.addWeighted(img, 0.5, cam_gb, 0.5, 0)
return result
# 加载图像
img = cv2.imread('test.jpg')
img = cv2.cvtColor(img, cv2.COLOR_BGR2RGB)
# 可视化Guided Grad-CAM
result = guided_grad_cam(img, target_class=15)
cv2.imshow('Guided Grad-CAM', result)
cv2.waitKey()
```
这个代码示例是使用PyTorch框架实现的,其中`model`是已经训练好的图像分割模型,`preprocess`函数是预处理函数,`GuidedBackpropRelu`函数是Guided Backpropagation模块,`guided_grad_cam`函数是Guided Grad-CAM函数。你需要将模型和预处理函数替换为你自己的模型和预处理函数,然后将图像和目标类别作为输入,即可可视化Guided Grad-CAM热力图。
生成Pytorch实现 Grad-CAM 基于 VGG16模型的代码
以下是基于VGG16模型的Grad-CAM PyTorch实现代码:
```python
import torch
import torch.nn as nn
import torch.nn.functional as F
from torchvision import models
from torchvision import transforms
from PIL import Image
class VGG16(nn.Module):
def __init__(self):
super(VGG16, self).__init__()
self.features = models.vgg16(pretrained=True).features
self.avgpool = nn.AdaptiveAvgPool2d((1, 1))
self.classifier = nn.Sequential(
nn.Linear(512 * 7 * 7, 4096),
nn.ReLU(inplace=True),
nn.Dropout(),
nn.Linear(4096, 4096),
nn.ReLU(inplace=True),
nn.Dropout(),
nn.Linear(4096, 1000),
)
def forward(self, x):
x = self.features(x)
x = self.avgpool(x)
x = torch.flatten(x, 1)
x = self.classifier(x)
return x
class GradCAM:
def __init__(self, model):
self.model = model.eval()
self.feature_maps = []
self.gradient_maps = []
# Registering hooks for feature maps and gradient maps
self.model.features.register_forward_hook(self.save_feature_maps)
self.model.features.register_backward_hook(self.save_gradient_maps)
def save_feature_maps(self, module, input, output):
# Save feature maps during forward pass
self.feature_maps.append(output)
def save_gradient_maps(self, module, grad_input, grad_output):
# Save gradient maps during backward pass
self.gradient_maps.append(grad_output[0])
def forward(self, x):
return self.model(x)
def backward(self, idx):
# Calculate gradients of the output with respect to feature maps
self.model.zero_grad()
grad_output = torch.zeros_like(self.gradient_maps[-1])
grad_output[0][idx] = 1
self.gradient_maps[-1].backward(gradient=grad_output)
def generate(self, x, idx):
# Forward pass to get the predicted class
self.forward(x)
# Backward pass to get the gradients
self.backward(idx)
# Pool the gradients over the feature maps and normalize
pooled_gradients = torch.mean(self.gradient_maps[-1], dim=[2, 3])
feature_maps = self.feature_maps[-1]
for i in range(feature_maps.shape[1]):
feature_maps[:, i, :, :] *= pooled_gradients[i]
heatmap = torch.mean(feature_maps, dim=1).squeeze().detach().numpy()
heatmap = np.maximum(heatmap, 0)
heatmap /= np.max(heatmap)
# Resize the heatmap to match the input image size
heatmap = cv2.resize(heatmap, (x.shape[3], x.shape[2]))
# Convert heatmap to RGB
heatmap = np.uint8(255 * heatmap)
heatmap = cv2.applyColorMap(heatmap, cv2.COLORMAP_JET)
# Superimpose the heatmap on the input image
superimposed_img = np.uint8(0.5 * x[0].permute(1, 2, 0).detach().numpy() + 0.5 * heatmap)
return superimposed_img
# Load the pre-trained VGG16 model
model = VGG16()
# Create GradCAM object
gradcam = GradCAM(model)
# Load the input image
img = Image.open('input.jpg').convert('RGB')
# Preprocess the input image
transform = transforms.Compose([
transforms.Resize((224, 224)),
transforms.ToTensor(),
transforms.Normalize(mean=[0.485, 0.456, 0.406], std=[0.229, 0.224, 0.225])
])
input_tensor = transform(img).unsqueeze(0)
# Get the predicted class index
output = gradcam.forward(input_tensor)
predicted_idx = torch.argmax(output).item()
# Generate the Grad-CAM heatmap
cam = gradcam.generate(input_tensor, predicted_idx)
# Save the output image
output_img = Image.fromarray(cam)
output_img.save('output.jpg')
```
这段代码包括了VGG16模型的定义、Grad-CAM的实现、输入图像的预处理以及结果图像的保存。你只需将`input.jpg`替换为你自己的输入图像,运行代码即可得到Grad-CAM可视化结果图像`output.jpg`。
相关推荐
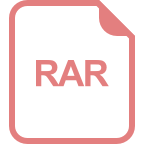












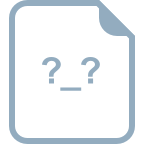