error: type mismatch; found : org.apache.spark.rdd.RDD[org.apache.spark.mllib.regression.LabeledPoint] required: org.apache.spark.sql.Dataset[_] val model = new NaiveBayes().fit(train)
时间: 2023-09-19 19:05:48 浏览: 44
这个错误是因为你传递给 NaiveBayes.fit() 方法的参数类型不正确。它需要一个 DataFrame,但你传递了一个 RDD。你需要将 RDD 转换为 DataFrame,可以使用 SparkSession.createDataFrame() 方法将 RDD 转换为 DataFrame,例如:
```
val spark = SparkSession.builder().appName("NaiveBayesExample").getOrCreate()
import spark.implicits._
val trainDF = train.toDF()
val model = new NaiveBayes().fit(trainDF)
```
其中,train 是一个 RDD[LabeledPoint],将其转换为 DataFrame 后才能传递给 NaiveBayes.fit() 方法。
相关问题
error: type mismatch; found : org.apache.spark.rdd.RDD[org.apache.spark.mllib.regression.LabeledPoint] required: org.apache.spark.sql.Dataset[_] val model = nb.fit(train)
这个错误是因为你传递给`fit()`函数的参数类型不正确。 它期望的是一个`org.apache.spark.sql.Dataset`类型的数据集,但是你传递的是一个`org.apache.spark.rdd.RDD`类型的数据集。
你需要将你的`train`数据集转换为`Dataset`类型,可以使用`toDF()`函数将RDD转换为DataFrame,然后使用`as`函数将DataFrame转换为Dataset。
示例代码如下:
```
import spark.implicits._
val trainDS = train.toDF().as[LabeledPoint]
val model = nb.fit(trainDS)
```
type mismatch;found : Array[String] required: org.apache.spark.sql.Column
这个错误通常发生在Spark SQL中,它表示您正在尝试将一个`Array[String]`类型的对象传递给需要`org.apache.spark.sql.Column`类型的对象的函数或方法。
解决此错误的方法是使用Spark SQL的内置函数`array()`将`Array[String]`转换为`Column`类型。例如,如果您正在编写一个使用`array_contains`函数来查找包含某个值的数组的查询,您可以按以下方式转换数组:
```scala
import org.apache.spark.sql.functions.array_contains
val myArray = Array("apple", "banana", "orange")
val myColumn = array_contains(array(myArray.map(lit(_)): _*), "apple")
```
在这个例子中,我们使用`array()`函数将`myArray`转换为`Column`类型,并使用`lit()`函数将每个元素转换为`Column`类型。然后,我们将转换后的列传递给`array_contains()`函数来执行我们的查询。
请注意,我们使用了`_*`操作符来展开数组,这是必需的,因为`array()`函数需要多个参数,而不是一个数组。
相关推荐
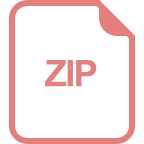
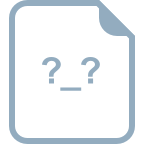













