def fitness_func(X): x = X[:, 0] y = X[:, 1] return x ** 2 + y ** 2 + x代码
时间: 2024-05-27 18:12:11 浏览: 28
def fitness_func(X):
x = X[:, 0]
y = X[:, 1]
return x ** 2 + y ** 2
# Note: the return statement should be x ** 2 + y ** 2, not x ** 2 y ** 2. The latter is invalid syntax.
相关问题
def fitness_func(X): x = X[:, 0] y = X[:, 1] return x ** 2 + y ** 2 + x
** 3 + y ** 3
This fitness function takes in a 2-dimensional array of solutions, where each row represents a solution with 2 variables x and y. It computes the fitness value for each solution using the formula x^2 + y^2 + x^3 + y^3.
The fitness value represents the quality of the solution, where higher values indicate better solutions. The function is designed to encourage solutions with higher x and y values, as well as solutions that have a balance between the x and y values.
Note that there is a syntax error in the code provided, where the return statement is not properly formatted. The corrected code should be:
def fitness_func(X):
x = X[:, 0]
y = X[:, 1]
return x ** 2 + y ** 2 + x ** 3 + y ** 3
请在不影响结果的条件下改变代码的样子:import numpy as np import matplotlib.pyplot as plt x1len = 21 x2len = 18 LEN = x1len + x2len POPULATION_SIZE = 100 GENERATIONS = 251 CROSSOVER_RATE = 0.7 MUTATION_RATE = 0.3 pop = np.random.randint(0,2,size=(POPULATION_SIZE,LEN)) def BinToX(pop): x1 = pop[:,0:x1len] x2 = pop[:,x1len:] x1 = x1.dot(2**np.arange(x1len)[::-1]) x2 = x2.dot(2**np.arange(x2len)[::-1]) x1 = -2.9 + x1*(12 + 2.9)/(np.power(2,x1len)-1) x2 = 4.2 + x2*(5.7 - 4.2)/(np.power(2,x2len)-1) return x1,x2 def func(pop): x1,x2 = BinToX(pop) return 21.5 + x1*np.sin(4*np.pi*x1) + x2*np.sin(20*np.pi*x2) def fn(pop): return func(pop); def selection(pop, fitness): idx = np.random.choice(np.arange(pop.shape[0]), size=POPULATION_SIZE, replace=True, p=fitness/fitness.sum()) return pop[idx] def crossover(IdxP1,pop): if np.random.rand() < CROSSOVER_RATE: C = np.zeros((1,LEN)) IdxP2 = np.random.randint(0, POPULATION_SIZE) pt = np.random.randint(0, LEN) C[0,:pt] = pop[IdxP1,:pt] C[0,pt:] = pop[IdxP2, pt:] np.append(pop, C, axis=0) return def mutation(idx,pop): if np.random.rand() < MUTATION_RATE: mut_index = np.random.randint(0, LEN) pop[idx,mut_index] = 1- pop[idx,mut_index] return best_chrom = np.zeros(LEN) best_score = 0 fig = plt.figure() for generation in range(GENERATIONS): fitness = fn(pop) pop = selection(pop, fitness) if generation%50 == 0: ax = fig.add_subplot(2,3,generation//50 +1, projection='3d', title = "generation:"+str(generation)+" best="+str(np.max(fitness))) x1,x2 = BinToX(pop) z = func(pop) ax.scatter(x1,x2,z) for idx in range(POPULATION_SIZE): crossover(idx,pop) mutation(idx,pop) idx = np.argmax(fitness) if best_score < fitness[idx]: best_score = fitness[idx] best_chrom = pop[idx, :] plt.show() print('最优解:', best_chrom, '| best score: %.2f' % best_score)
import numpy as np
import matplotlib.pyplot as plt
x1len = 21
x2len = 18
LEN = x1len + x2len
POPULATION_SIZE = 100
GENERATIONS = 251
CROSSOVER_RATE = 0.7
MUTATION_RATE = 0.3
pop = np.random.randint(0,2,size=(POPULATION_SIZE,LEN))
def BinToX(pop):
x1 = pop[:,0:x1len]
x2 = pop[:,x1len:]
x1 = -2.9 + x1*(12 + 2.9)/(np.power(2,x1len)-1)
x2 = 4.2 + x2*(5.7 - 4.2)/(np.power(2,x2len)-1)
return x1,x2
def func(x1,x2):
return 21.5 + x1*np.sin(4*np.pi*x1) + x2*np.sin(20*np.pi*x2)
def fn(pop):
x1,x2 = BinToX(pop)
return func(x1,x2);
def selection(pop, fitness):
idx = np.random.choice(np.arange(pop.shape[0]),size=POPULATION_SIZE, replace=True, p=fitness/fitness.sum())
return pop[idx]
def crossover(IdxP1,pop):
if np.random.rand() < CROSSOVER_RATE:
C = np.zeros((1,LEN))
IdxP2 = np.random.randint(0, POPULATION_SIZE)
pt = np.random.randint(0, LEN)
C[0,:pt] = pop[IdxP1,:pt]
C[0,pt:] = pop[IdxP2, pt:]
np.append(pop, C, axis=0)
return
def mutation(idx,pop):
if np.random.rand() < MUTATION_RATE:
mut_index = np.random.randint(0, LEN)
pop[idx,mut_index] = 1- pop[idx,mut_index]
return
best_chrom = np.zeros(LEN)
best_score = 0
fig = plt.figure()
for generation in range(GENERATIONS):
fitness = fn(pop)
pop = selection(pop, fitness)
if generation%50 == 0:
ax = fig.add_subplot(2,3,generation//50 +1, projection='3d',title = "generation:"+str(generation)+" best="+str(np.max(fitness)))
x1,x2 = BinToX(pop)
z = func(x1,x2)
ax.scatter(x1,x2,z)
for idx in range(POPULATION_SIZE):
crossover(idx,pop)
mutation(idx,pop)
idx = np.argmax(fitness)
if best_score < fitness[idx]:
best_score = fitness[idx]
best_chrom = pop[idx, :]
plt.show()
print('最优解:', best_chrom, '| best score: %.2f' % best_score)
相关推荐
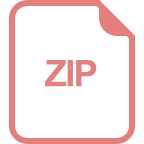
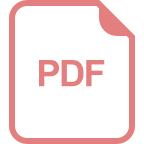












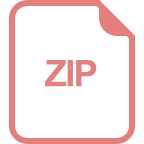