Error in ps(max_depth = p_int(lower = 3, upper = 5), num_leaves = p_int(lower = 2, : unused arguments (max_depth = p_int(lower = 3, upper = 5), num_leaves = p_int(lower = 2, upper = 30), bagging_fraction = p_dbl(lower = 0.8, upper = 1), feature_fraction = p_dbl(lower = 0.8, upper = 1), lambda_l1 = p_int(lower = 0, upper = 1000), lambda_l2 = p_int(lower = 0, upper = 1000))什么意思
时间: 2023-06-26 12:03:22 浏览: 65
这个错误提示表明,在运行某个函数时,你传递了无效的参数。具体来说,你传递了一个名为`max_depth`和`num_leaves`的参数,但是该函数没有定义这些参数。相反,该函数定义了其他参数,如`bagging_fraction`、`feature_fraction`、`lambda_l1`和`lambda_l2`等。你应该检查函数的定义并确保你传递的参数名称与函数定义中的名称匹配。
相关问题
mysql: [ERROR] unknown variable 'lower_case_table_names=1'.
根据提供的引用内容,出现了一个错误:mysql: [ERROR] unknown variable 'lower_case_table_names=1'。这个错误是由于在配置文件中设置了一个未知的变量'lower_case_table_names=1'导致的。为了解决这个问题,可以按照以下步骤进行操作:
1. 打开MySQL配置文件my.cnf:
```shell
cat /opt/mysql/etc/4444/my.cnf
```
2. 确保在配置文件中没有设置未知的变量'lower_case_table_names=1'。如果有,将其删除或注释掉。
3. 保存并关闭配置文件。
4. 重启MySQL服务:
```shell
systemctl restart mysqld_4444.service
```
这样就可以解决错误:mysql: [ERROR] unknown variable 'lower_case_table_names=1'。
def fitness_function(self, params): # 解压参数 learning_rate, n_estimators, max_depth, min_child_weight, subsample, colsample_bytree, gamma = params # 初始化模型 model = XGBRegressor( learning_rate=learning_rate, n_estimators=int(n_estimators), max_depth=int(max_depth), min_child_weight=int(min_child_weight), subsample=subsample, colsample_bytree=colsample_bytree, gamma=gamma, random_state=42, n_jobs=self.n_jobs ) # 训练模型 model.fit(train_features, train_target) # 预测 y_pred = model.predict(train_features) # 计算均方误差 mse = mean_squared_error(train_target, y_pred)
在这个函数中,`params` 是一个包含七个参数值的列表,用于设置 XGBoost 模型的超参数。如果 `params` 中的值不足七个,那么解包操作就会失败并引发错误。你可以尝试以下这些改进方式:
1. 检查 `params` 列表的长度:在函数体中,你可以先检查 `params` 列表的长度是否为七个,如果不是,就抛出一个异常或者返回一个错误代码。这样可以确保在解包 `params` 列表之前,列表中包含了正确数量的参数值。
```python
def fitness_function(self, params):
if len(params) != 7:
raise ValueError("params should contain 7 values")
# 解包参数
learning_rate, n_estimators, max_depth, min_child_weight, subsample, colsample_bytree, gamma = params
# ...
```
2. 使用默认值:如果你在定义函数时为这些参数提供了默认值,那么你可以在调用函数时不传递这些参数,从而使用默认值。这样可以避免解包 `params` 列表,也可以防止出现参数数量不足的错误。
```python
def fitness_function(self, params=[0.1, 100, 10, 1, 0.8, 0.8, 0.1]):
# 使用默认值
learning_rate, n_estimators, max_depth, min_child_weight, subsample, colsample_bytree, gamma = params
# ...
```
在这个例子中,`params` 列表包含了默认的参数值,如果调用函数时不传递 `params` 参数,则使用默认值。
3. 使用 `*args` 和 `**kwargs`:如果你不想限制参数的数量,可以使用可变长度参数 `*args` 和 `**kwargs`。这些参数可以接受任意数量的位置参数和关键字参数,使函数更加灵活。
```python
def fitness_function(self, *args, **kwargs):
# 获取参数值或使用默认值
learning_rate = kwargs.get('learning_rate', 0.1)
n_estimators = kwargs.get('n_estimators', 100)
max_depth = kwargs.get('max_depth', 10)
min_child_weight = kwargs.get('min_child_weight', 1)
subsample = kwargs.get('subsample', 0.8)
colsample_bytree = kwargs.get('colsample_bytree', 0.8)
gamma = kwargs.get('gamma', 0.1)
# ...
```
在这个例子中,`*args` 表示接受任意数量的位置参数,`**kwargs` 表示接受任意数量的关键字参数。在函数中,你可以使用 `kwargs.get()` 方法获取传递的参数值。如果某个参数没有传递,则使用默认值。
阅读全文
相关推荐
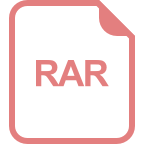
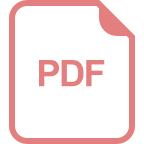














