gs = max(int(model.stride.max()), 32) # grid size (max stride) imgsz = check_img_size(opt.imgsz, gs, floor=gs * 2) # verify imgsz is gs-multiple
时间: 2024-03-11 19:44:02 浏览: 35
这段代码用于计算图像的输入大小`imgsz`。首先,它使用`model.stride.max()`方法计算模型中所有层的步长`stride`的最大值,并将其转换为整数类型。这个最大步长就是模型输出特征图的最小大小。然后,它使用`check_img_size`函数检查输入图像的大小`opt.imgsz`是否是步长`stride`的倍数,并将其向下取整为步长的两倍。这样做是为了保证模型输出的特征图大小是整数倍的步长,方便后续计算。最终,计算出的`imgsz`就是模型输入图像的大小。
相关问题
代码解释 # Load model model = attempt_load(weights, map_location=device) # load FP32 model stride = int(model.stride.max()) # model stride imgsz = check_img_size(imgsz, s=stride) # check img_size
这段代码的作用是加载模型(通过指定的权重文件路径),并检查模型的输入图像尺寸是否符合要求。具体解释如下:
- `attempt_load(weights, map_location=device)` 会返回一个加载了指定权重文件的模型。其中,`weights` 是指定的权重文件路径,`map_location` 是指定模型在哪个设备上运行。
- `stride = int(model.stride.max())` 用于获取模型的最大步幅(stride),并将其转换为整数类型。步幅是指模型在处理图像时,每次移动的像素数目。
- `check_img_size(imgsz, s=stride)` 用于检查输入图像的尺寸是否符合要求。其中,`imgsz` 是指定的输入图像尺寸,`s` 是模型的步幅。如果输入图像的尺寸不符合要求,则会将其调整为最接近的符合要求的尺寸。
self.stride = int(model.stride.max())
这行代码的作用是将模型的 stride(步长)属性的最大值转换为整数,并将其赋值给 self.stride 变量。stride 是指卷积核在对输入数据进行卷积操作时每次滑动的步长,即卷积核每次移动的像素数。在深度学习中,stride 是一个非常重要的超参数,可以影响模型的性能和速度。该行代码的目的可能是为了保证模型的步长是整数类型,方便后续计算。
相关推荐
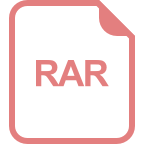
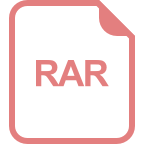
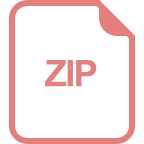













