super(channel_selection, self).__init__() self.indexes = nn.Parameter(torch.ones(num_channels))
时间: 2024-05-23 12:15:53 浏览: 215
这两行代码是一个类的构造函数,用于初始化类的属性。第一行代码调用了父类的构造函数并传递了 `channel_selection` 参数,以便父类可以正确地初始化。第二行代码创建了一个名为 `indexes` 的可训练参数(`nn.Parameter`),它是一个大小为 `num_channels` 的张量,每个元素都被初始化为 1。这个参数在模型训练时会被动态更新,用于选择输入数据中的哪些通道参与计算。
相关问题
def forward(self, data, org_edge_index): x = data.clone().detach() edge_index_sets = self.edge_index_sets device = data.device batch_num, node_num, all_feature = x.shape x = x.view(-1, all_feature).contiguous() gcn_outs = [] for i, edge_index in enumerate(edge_index_sets): edge_num = edge_index.shape[1] cache_edge_index = self.cache_edge_index_sets[i] if cache_edge_index is None or cache_edge_index.shape[1] != edge_num*batch_num: self.cache_edge_index_sets[i] = get_batch_edge_index(edge_index, batch_num, node_num).to(device) batch_edge_index = self.cache_edge_index_sets[i] all_embeddings = self.embedding(torch.arange(node_num).to(device)) weights_arr = all_embeddings.detach().clone() all_embeddings = all_embeddings.repeat(batch_num, 1) weights = weights_arr.view(node_num, -1) cos_ji_mat = torch.matmul(weights, weights.T) normed_mat = torch.matmul(weights.norm(dim=-1).view(-1,1), weights.norm(dim=-1).view(1,-1)) cos_ji_mat = cos_ji_mat / normed_mat dim = weights.shape[-1] topk_num = self.topk topk_indices_ji = torch.topk(cos_ji_mat, topk_num, dim=-1)[1] self.learned_graph = topk_indices_ji gated_i = torch.arange(0, node_num).T.unsqueeze(1).repeat(1, topk_num).flatten().to(device).unsqueeze(0) gated_j = topk_indices_ji.flatten().unsqueeze(0) gated_edge_index = torch.cat((gated_j, gated_i), dim=0) batch_gated_edge_index = get_batch_edge_index(gated_edge_index, batch_num, node_num).to(device) gcn_out = self.gnn_layers[i](x, batch_gated_edge_index, node_num=node_num*batch_num, embedding=all_embeddings) gcn_outs.append(gcn_out) x = torch.cat(gcn_outs, dim=1) x = x.view(batch_num, node_num, -1) indexes = torch.arange(0,node_num).to(device) out = torch.mul(x, self.embedding(indexes)) out = out.permute(0,2,1) out = F.relu(self.bn_outlayer_in(out)) out = out.permute(0,2,1) out = self.dp(out) out = self.out_layer(out) out = out.view(-1, node_num) return out
这是一个PyTorch模型的前向传播函数,它接受两个参数:data和org_edge_index。该函数使用GCN(图卷积神经网络)来处理输入数据,并返回一个输出张量。具体实现细节可以参考代码中的注释。
class AbstractGreedyAndPrune(): def __init__(self, aoi: AoI, uavs_tours: dict, max_rounds: int, debug: bool = True): self.aoi = aoi self.max_rounds = max_rounds self.debug = debug self.graph = aoi.graph self.nnodes = self.aoi.n_targets self.uavs = list(uavs_tours.keys()) self.nuavs = len(self.uavs) self.uavs_tours = {i: uavs_tours[self.uavs[i]] for i in range(self.nuavs)} self.__check_depots() self.reachable_points = self.__reachable_points() def __pruning(self, mr_solution: MultiRoundSolution) -> MultiRoundSolution: return utility.pruning_multiroundsolution(mr_solution) def solution(self) -> MultiRoundSolution: mrs_builder = MultiRoundSolutionBuilder(self.aoi) for uav in self.uavs: mrs_builder.add_drone(uav) residual_ntours_to_assign = {i : self.max_rounds for i in range(self.nuavs)} tour_to_assign = self.max_rounds * self.nuavs visited_points = set() while not self.greedy_stop_condition(visited_points, tour_to_assign): itd_uav, ind_tour = self.local_optimal_choice(visited_points, residual_ntours_to_assign) residual_ntours_to_assign[itd_uav] -= 1 tour_to_assign -= 1 opt_tour = self.uavs_tours[itd_uav][ind_tour] visited_points |= set(opt_tour.targets_indexes) # update visited points mrs_builder.append_tour(self.uavs[itd_uav], opt_tour) return self.__pruning(mrs_builder.build()) class CumulativeGreedyCoverage(AbstractGreedyAndPrune): choice_dict = {} for ind_uav in range(self.nuavs): uav_residual_rounds = residual_ntours_to_assign[ind_uav] if uav_residual_rounds > 0: uav_tours = self.uavs_tours[ind_uav] for ind_tour in range(len(uav_tours)): tour = uav_tours[ind_tour] quality_tour = self.evaluate_tour(tour, uav_residual_rounds, visited_points) choice_dict[quality_tour] = (ind_uav, ind_tour) best_value = max(choice_dict, key=int) return choice_dict[best_value] def evaluate_tour(self, tour : Tour, round_count : int, visited_points : set): new_points = (set(tour.targets_indexes) - visited_points) return round_count * len(new_points) 如何改写上述程序,使其能返回所有已经探索过的目标点visited_points的数量,请用代码表示
可以在 `solution()` 方法中添加一个变量来记录已经探索过的目标点数量,然后在每次更新 `visited_points` 后更新这个变量。下面是修改后的代码:
```
class AbstractGreedyAndPrune():
def __init__(self, aoi: AoI, uavs_tours: dict, max_rounds: int, debug: bool = True):
self.aoi = aoi
self.max_rounds = max_rounds
self.debug = debug
self.graph = aoi.graph
self.nnodes = self.aoi.n_targets
self.uavs = list(uavs_tours.keys())
self.nuavs = len(self.uavs)
self.uavs_tours = {i: uavs_tours[self.uavs[i]] for i in range(self.nuavs)}
self.__check_depots()
self.reachable_points = self.__reachable_points()
def __pruning(self, mr_solution: MultiRoundSolution) -> MultiRoundSolution:
return utility.pruning_multiroundsolution(mr_solution)
def solution(self) -> Tuple[MultiRoundSolution, int]:
mrs_builder = MultiRoundSolutionBuilder(self.aoi)
for uav in self.uavs:
mrs_builder.add_drone(uav)
residual_ntours_to_assign = {i : self.max_rounds for i in range(self.nuavs)}
tour_to_assign = self.max_rounds * self.nuavs
visited_points = set()
explored_points = 0
while not self.greedy_stop_condition(visited_points, tour_to_assign):
itd_uav, ind_tour = self.local_optimal_choice(visited_points, residual_ntours_to_assign)
residual_ntours_to_assign[itd_uav] -= 1
tour_to_assign -= 1
opt_tour = self.uavs_tours[itd_uav][ind_tour]
new_points = set(opt_tour.targets_indexes) - visited_points
explored_points += len(new_points)
visited_points |= new_points # update visited points
mrs_builder.append_tour(self.uavs[itd_uav], opt_tour)
return self.__pruning(mrs_builder.build()), explored_points
class CumulativeGreedyCoverage(AbstractGreedyAndPrune):
def evaluate_tour(self, tour : Tour, round_count : int, visited_points : set):
new_points = set(tour.targets_indexes) - visited_points
return round_count * len(new_points)
def local_optimal_choice(self, visited_points, residual_ntours_to_assign):
choice_dict = {}
for ind_uav in range(self.nuavs):
uav_residual_rounds = residual_ntours_to_assign[ind_uav]
if uav_residual_rounds > 0:
uav_tours = self.uavs_tours[ind_uav]
for ind_tour in range(len(uav_tours)):
tour = uav_tours[ind_tour]
quality_tour = self.evaluate_tour(tour, uav_residual_rounds, visited_points)
choice_dict[quality_tour] = (ind_uav, ind_tour)
best_value = max(choice_dict, key=int)
return choice_dict[best_value]
阅读全文
相关推荐
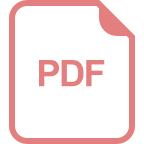
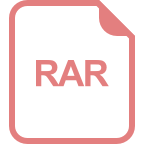
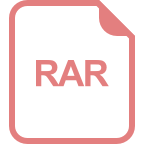












